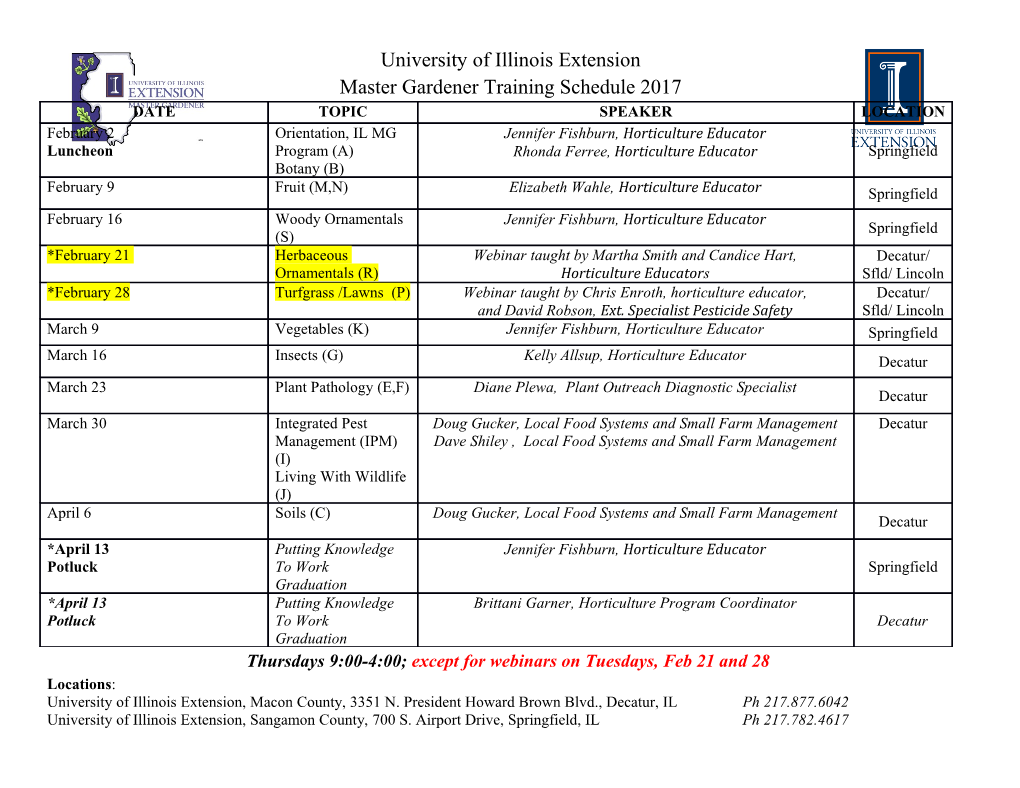
Artificial Intelligence and Augmented Intelligence for Automated Investigations for Scientific Discovery Dial-a-Molecule Grand Challenge Network Directed Assembly Grand Challenge Network AI3 Science Discovery Network+ & Dial-a-Molecule Network & Directed Assembly Network: AI for Reaction Outcome & Synthetic Route Prediction Conference 09-11/03/2020 DeVere Tortworth Court Hotel Tortworth, Wotton Under Edge, GL12 8HH Dr. Wendy A. Warr Wendy Warr & Associates 04/05/2020 AI3SD-Event-Series:Report-17 AI3 Science Discovery Network+ & Dial-a-Molecule Network & Directed Assembly Network: AI for Reaction Outcome & Synthetic Route Prediction Conference AI3SD-Event-Series:Report-17 04/05/2020 DOI: 10.5258/SOTON/P0021 Published by University of Southampton Network: Artificial Intelligence and Augmented Intelligence for Automated Investigations for Scientific Discovery This Network+ is EPSRC Funded under Grant No: EP/S000356/1 Principal Investigator: Professor Jeremy Frey Co-Investigator: Professor Mahesan Niranjan Network+ Coordinator: Dr Samantha Kanza Network: Dial-a-Molecule This Network+ is EPSRC Funded under Grant No: EP/P007589/1 Principal Investigator: Professor Richard Whitby Co-Investigator: Dr Mimi Hii Network+ Coordinator: Dr Gill Smith Network: Directed Assembly This Network+ is EPSRC Funded under Grant No: EP/P007279/1 Principal Investigator: Professor Harris Makatsoris Co-Investigator: Professor Paul Raithby Network+ Coordinator: Mr Martin Elliott Contents 1 Event Details........................................ 1 2 Welcome and Introduction ................................ 1 3 Computer-assisted design of complex organic syntheses, 50 years on......... 1 4 Gathering molecules: representations and machine learning with minimal data... 4 5 Introduction to ML and structured matrix methods for learning outliers....... 7 6 Applying AI to retrosynthesis in the wilderness..................... 11 7 Reproducibility in chemistry ............................... 13 8 Accurate excited states calculations on near term quantum computers . 14 9 Making sense of predicted routes: the use of data as evidence for predictions in SciFinder.......................................... 16 10 What is the importance of false reactions for efficient data-driven retrosynthetic analysis? .......................................... 17 11 Combining artificial intelligence with structured high quality data in chemistry: delivering outstanding predictive chemistry applications................ 17 12 Intelligence from data: towards prediction in organometallic catalysis . 18 13 Chemistry ontologies and artificial intelligence ..................... 21 14 UDM: a community-driven data format for the exchange of comprehensive reaction information.................................... 24 15 Retrosynthesis via machine learning........................... 26 16 From mechanisms to reaction selectivity......................... 29 17 Reaction prediction in process chemistry with hybrid mechanistic and machine learning models ...................................... 31 18 Automated mining of a database of 9.3 million reactions from the patent literature, and its application to synthesis planning .................. 33 19 The semantic laboratory ................................. 37 20 ASKCOS: data-driven chemical synthesis........................ 38 21 Integrating AI with robust automated chemistry: AI-driven route design and automated reaction and route validation ........................ 42 22 A nondeterministic Chemputer for running chemical programs............ 45 23 Data-driven exploration of the catalytic reductive amination reaction . 48 24 Machine-assisted flow chemistry for organic synthesis ................. 50 25 Encoding solvents and product outcomes to improve reaction prediction systems . 51 26 Evolutionary computing strategies and feedback control for directed execution and optimization of chemical reactions............................ 54 27 Computational design via metal-driven self-assembly: from molecular building blocks to emerging functional materials......................... 56 28 Predictive models for assessing conditions of hydrogenation reactions . 59 29 Retrosynthetic software for practicing chemists: novel and efficient in silico pathway design validated at the bench.......................... 61 30 Conclusion......................................... 63 References............................................. 63 A report by Wendy A. Warr ([email protected]) on a three-day residential meeting organized by the Dial-a-Molecule, Directed Assembly and AI3SD Networks at the De Vere Tortworth on March 9-11, 2020. 1 Event Details Title AI for Reaction Outcome & Synthetic Route Prediction Conference Organisers AI3 Science Discovery Network+, Dial-a-Molecule Grand Challenge Network & Directed Assembly Grand Challenge Network Dates 09-11/03/2020 Programme Programme No. Participants 99 Location Tortworth, Wotton Under Edge, GL12 8HH Organisation Dr Gill Smith, Dial-a-Molecule Network, Committee Dr Samantha Kanza, AI3 Science Discovery Network+ & Mr Martin Elliott, Directed Assembly Conference Chairs Professor Richard Whitby, Dial-a-Molecule Network, Professor Jeremy Frey, AI3 Science Discovery Network+ & Professor Harris Makatsoris, Directed Assembly 2 Welcome and Introduction Professor Richard Whitby, University of Southampton The meeting was organized by the Dial-a-Molecule,1,2 Directed Assembly,3 andAI 3 Science Discovery4 Networks. Dial-a-Molecule’s vision is that in 20-40 years, scientists will be able to deliver any desired molecule within a timeframe useful to the end-user, using safe, economically viable and sustainable processes. Predicting the outcome of unknown reactions is a key challenge, and a key problem is lack of data, particularly on “failed” reactions. Synthesis must become a data-driven discipline. Since 2011 the Dial-a-Molecule Network has organized many meetings around the themes of collecting better data and using automated reaction platforms for repeatable and captured procedures. A recent success has been the establishment of the center for Rapid Online Analysis of Reactions (ROAR) at Imperial College.5 3 Computer-assisted design of complex organic syntheses, 50 years on Professor Peter Johnson, University of Leeds (talk sponsored by CAS) The goal of Corey and Wipke’s seminal work on computer-aided organic synthesis, the foundation for the Logic and Heuristics Applied to Synthetic Analysis (LHASA) program, was to assist chemists to find complete synthetic routes to target molecules.6 Organic Chemical Synthesis Simulation (OCSS) preceded LHASA. It used a large PDP10 computer7 that probably had a fraction of the computer power of today’s smart cell phones. 1 In a typical retrosynthetic analysis,8 level one precursors of a target molecule are found, and then precursors of those precursors are found, and so on, until an available starting material is identified, at which point the search terminates. In the absence of powerful strategies or frequent user pruning, the combinatorial nature of this approach prohibits very broad searches (many alternative reactions) as well as very deep searches (many steps between starting materials and products). For many systems the retrosynthetic analysis is driven by rules (“transforms”) describing the scope, limitations, and structure changes associated with a reaction. The reaction core provides a description of the essential structural features which must be present in the product for the transform to be applied; the extended reaction core contains additional information about individual atoms to make sure it is only applied in the correct situation. This may include the exclusion of structural features. In LHASA transforms were hand coded. Throughput was slow and newer advances in synthetic chemistry can invalidate older rules. Usage of LHASA declined when reaction databases became available. In the 2000s, Pfizer approached Simbiosys, a Toronto based cheminformatics company, to develop a retrosynthetic tool “ARChem Route Designer” (later named ChemPlanner). The aim was to perform rule- and precedent-based retrosynthetic analysis of target molecules back to readily available materials, and where possible, provide automated generation of retrosynthetic reaction rules. Other requirements were to provide a comprehensive set of alternative routes to a given target; allow user guidance and control; and provide literature examples of the suggested transformations, and direct access to details of starting materials. In ARChem, rules are extracted from a reaction database, and reaction cores identified from a reaction file with mapping of the atoms and bonds which were changed, made or broken in the reaction. The extracted core is extended to include all structural features essential for the reaction to occur, not those that are just “passengers”. Literature examples of the reaction type, for example esterification, are clustered together and an esterification rule is generated. Generalized rules might involve a nucleofuge (NF), that is, a leaving group which carries away a bonding electron pair. In the completed reaction rule, the generalized NF group is replaced by the most common group, but it is recognized that many alternative nucleofuges could also work. This grouping together of similar reactions in a single rule is one of many heuristics used to reduce the combinatorial explosion. Extended core perception requires chemistry judgment and knowledge of reaction mechanism. Examples are classified according to perceived mechanism
Details
-
File Typepdf
-
Upload Time-
-
Content LanguagesEnglish
-
Upload UserAnonymous/Not logged-in
-
File Pages82 Page
-
File Size-