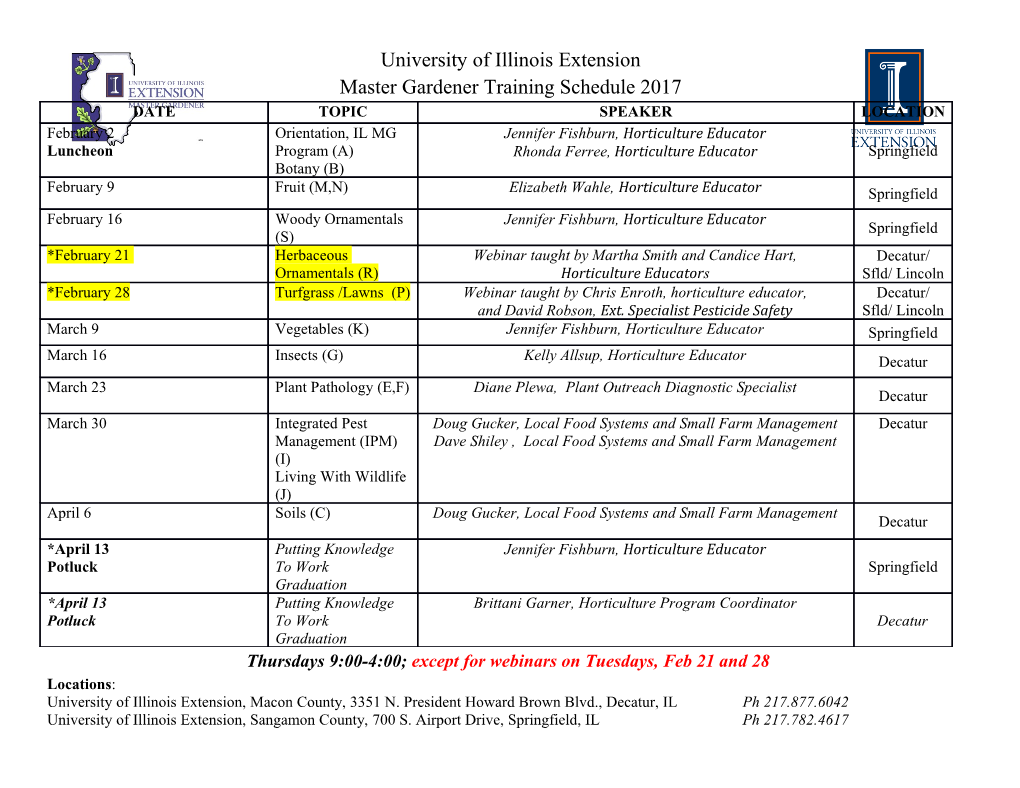
Proceedings of the Twenty-Fourth International Joint Conference on Artificial Intelligence (IJCAI 2015) Character-Based Parsing with Convolutional Neural Network Xiaoqing Zheng, Haoyuan Peng, Yi Chen, Pengjing Zhang, Wenqiang Zhang School of Computer Science, Fudan University, Shanghai, China Shanghai Key Laboratory of Intelligent Information Processing {zhengxq, penghy11, yichen11, pengjingzhang13, wqzhang}@fudan.edu.cn Abstract preserves the relative positions of the most relevant features, and thus it is sensitive to the order of the words in the input We describe a novel convolutional neural network k sentence. Experimental results show that the KMCNN can architecture with -max pooling layer that is able successfully recover the structure of Chinese sentences. to successfully recover the structure of Chinese sentences. This network can capture active fea- The KMCNN is different from an adapted Graph Trans- tures for unseen segments of a sentence to mea- former Network with single local max pooling operation [ ] sure how likely the segments are merged to be the (GTN) introduced by Collobert, 2011 for discriminative constituents. Given an input sentence, after all the parsing. The advantage of the GTN is that it does not depend scores of possible segments are computed, an ef- on external language-specific features such as those derived ficient dynamic programming parsing algorithm is from the constituency or dependency parse trees. However, used to find the globally optimal parse tree. A sim- its single max pooling operation cannot discriminate whether ilar network is then applied to predict syntactic cat- a relevant feature occurs just one or several times, and also egories for every node in the parse tree. Our net- neglects the relative order in which the features occur. The works archived competitive performance to exist- order of the words does matter to the meaning or the structure ing benchmark parsers on the CTB-5 dataset with- of the sentences, which is very useful for parsing or semantic out any task-specific feature engineering. analysis. Another limitation of the convolutional neural net- work with single max pooling operator is that if most or all of the highest values occur in a segment, the outputs of the max 1 Introduction pooling layer for larger segments containing this segment are Syntactic parsing of natural langauge sentences is one of the very close or equivalent to each other, and those segments most fundamental NLP tasks because of its importance in cannot be distinguished in such cases. mediating between linguistic meaning and expression. Chi- Kalchbrenner et al [2014] proposed a more sophisticated nese parsing has also received steady attention since the re- network, called Dynamic Convolutional Neural Network lease of Penn Chinese Treebank [Xue et al., 2005]. Most of (DCNN), in which multiple convolutional layers interleaved the previous work has focused on finding relevant features with k-max pooling layers are stacked to derive higher level for the model component, and on finding effective statistical features. In the DCNN, the k is a variable that depends on techniques for parameter estimation. Although such perfor- the length of an input sentence and the depth of the net- mance improvements can be useful in practice, there is a great work. The DCNN is effective in modeling whole sentences temptation to optimize the system’s performance for a spe- for many sentence-level tasks including sentiment prediction, cific benchmark. Furthermore, such systems, especially for and question type classification, but its benefit may not be ap- those that use joint solution, usually involve a great number plicable to the case of sentence segment, much smaller units of features, which makes engineering effective task-specific than sentence. The KMCNN is also different from the DCNN features and structured learning of parameters very hard. in how the convolution is computed. The DCNN used one- We address the Chinese parsing task by avoiding feature dimensional convolution that is to take the dot product of the engineering, which falls in line with the recent efforts to sur- filter vector with each n-gram in the sentence at the same pass the state-of-the-art for many NLP tasks by “deep” learn- dimension to obtain another feature vector, whereas the con- ing. We introduce a convolutional neural network with k-max volution in the KMCNN is calculated by performing affine pooling layer, called KMCNN, which can be used to produce transformations over each feature window (concatenation of a score for each segment in an input sentence. Each produced multiple consecutive feature vectors) to extract local features. score measures how likely the segment is to become a con- There are two major contributions in this paper. (1) We stituent in the parse tree. The k-max pooling operation is describe a convolutional neural network architecture with k- applied on the output of the convolutional layer, and pools max pooling layer (KMCNN), which is able to capture the the k most active features in each dimension to capture syn- most active features of sentence segments of varying length, tactic and semantic information of a sentence segment, which and can be used to recover the structure of sentences in com- 1054 bination with a dynamic programming decoder; (2) We ap- of this new node is the concatenation of replaced node plied KMCNN to parse Chinese sentences, and achieved the labels, such as “IP-VP” in Figure 1 (b). state-of-art performance in constituent parsing by transferring • Functional labels as well as traces are removed. intermediate representations learned on large unlabeled data without task-specific feature engineering. This transformation added 61 extra tags to 22 already had in the CTB. The first step breaks multi-branching nodes down 2 Parse Tree Representation into binary-branching nodes by inserting temporary nodes. Parsing can be viewed as a “chunking” process which recur- Temporary nodes are collapsed and removed when we trans- sively finds chunks of phrases or constituents in a sentence. form a binary tree back into the multi-branching tree for test- Words are first merged into phrases, and then the phrases are ing. The inverse operation is also performed on the nodes further merged into larger constituents in a syntactically and having concatenated labels at test time. semantically meaningful order until the full parse tree is de- Previous studies show that Chinese syntactic parsing by us- duced. The search space is proportional to the number of ing character-level information and joint solution (i.e., per- possible parse trees. Even in grammars without unary chains forming word segmentation, POS tagging, and parsing at the or empty elements, the number of parses is generally expo- same time) usually leads to the improvement in accuracy over nential in the length of the sentence. For reducing the search pipelined systems because the error propagation is avoided space, we focus on binary trees, which also allow us to use and the various information normally used in the different efficient dynamic programming algorithms for decoding. steps of pipelined systems can be integrated [Qian and Liu, 2012; Wang et al., 2013; Zhang et al., 2013]. Characters, in /6 fact, play an important role in the character-based languages, 44 <6 such as Chinese and Japanese. They act as basic morpholog- ڃݛ Government ical, syntactic and semantic units in a sentence. << 46 /6 Ԋ The internal structures of words can be recovered through encourage 00 44 <6 ’ࠨਗ ѢЎ؞ morphological analysis. For example, ‘entrepreneur private entrepreneur << 46 is a word derived by adding the suffix ‘expert noun plural ઞۯ invest 44 44 44 suffix’ to the base ‘enterprize’, as shown in Figure 1 (c). ؞ ׁक ੦ݲ֡ /6 (a) national basic facilities Like [Zhang et al., 2013], we extended the notation of phrase- structure trees, and expanded word nodes into their internal 44 <6 ڃݛ Government structures. Using these extended annotations, the joint word << <6) Ԋ segmentation, POS tagging, and parsing can be performed by encourage 46 /6<6 starting with the character-level in a unified manner. 00 44 << 46 ࠨਗ ѢЎ؞ ઞۯ private entrepreneur invest 44 46) 3 The Network Architecture ؞֡ national 44 44 क ੦ݲ We choose to use a variant of convolutional neural networkׁ (b) basic facilities k 44 44 with -max pooling layer to find the structure of sentences. ѢЎ؞ entrepreneur k ؞ The network architecture is shown in Figure 2. The -max (44 expert Ѣ Ў pooling operations are applied in the network after the con- attempt business k (c) volutional layers, which are used to pool the most active features at low levels. This network preserves the relative po- sitions of the most relevant features, and thus it is sensitive to Figure 1: Parse tree representations. (a) Parse tree as in the the order of the words in input sentences. Convolutional lay- CTB. (b) Transformed binary tree after concatenating nodes ers are often stacked, interleaved with a non-linearity func- spanning the same constituents. (c) Expanding the word node tion, to extract higher level features (only two convolutional to its character-level tree through morphological analysis. layers are drawn in Figure 2 for clarity). The topmost k-max A parse tree in the Penn Chinese Treebank (CTB) is shown pooling layer also guarantees that the input to the next layer in Figure 1 (a). The root spans all words of the sentence, and of the network is independent of the length of an input sen- it is recursively decomposed into smaller constituents with la- tence, in order to apply the same subsequent layers. bels like VP (verb phrase), NP (noun phrase), etc. We trans- The input to the KMCNN is a sentence segment, and the formed the parse trees into the equivalent binary forms like network induces a score for the segment which measures how Figure 1 (b) for training.
Details
-
File Typepdf
-
Upload Time-
-
Content LanguagesEnglish
-
Upload UserAnonymous/Not logged-in
-
File Pages7 Page
-
File Size-