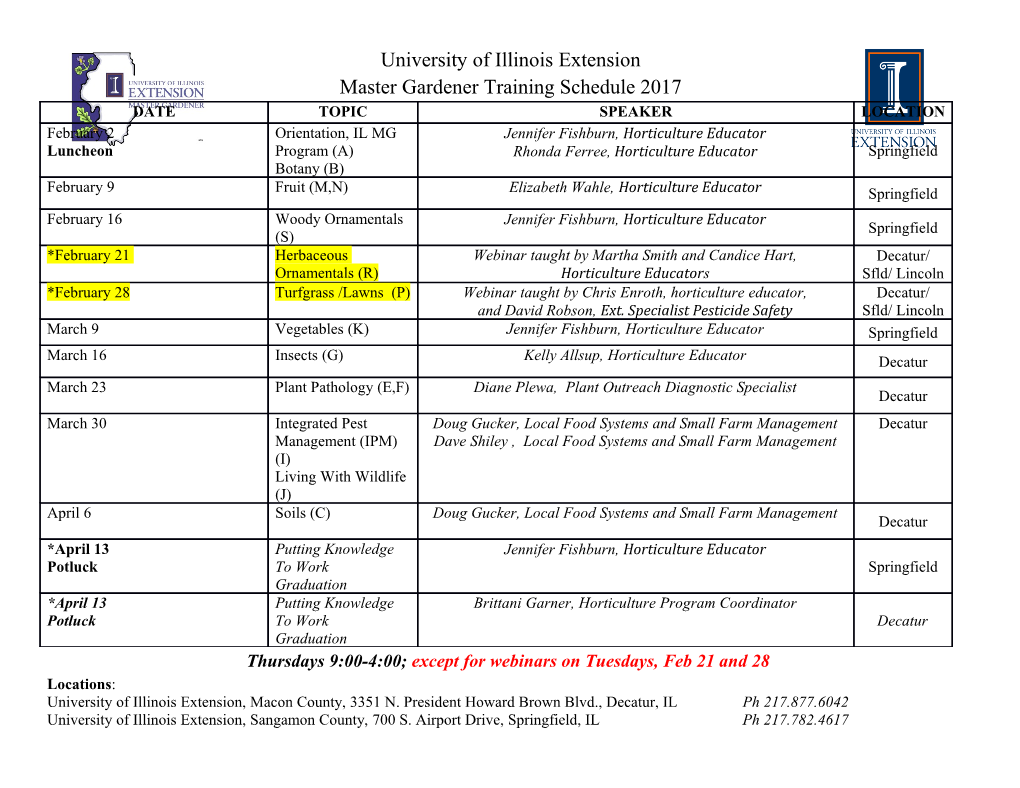
Lectures on L¶evyProcesses, Stochastic Calculus and Financial Applications, Ovronnaz September 2005 David Applebaum Probability and Statistics Department, University of She±eld, Hicks Building, Houns¯eld Road, She±eld, England, S3 7RH e-mail: D.Applebaum@she±eld.ac.uk Introduction A L¶evyprocess is essentially a stochastic process with stationary and in- dependent increments. The basic theory was developed, principally by Paul L¶evyin the 1930s. In the past 15 years there has been a renaissance of interest and a plethora of books, articles and conferences. Why ? There are both theoretical and practical reasons. Theoretical ² There are many interesting examples - Brownian motion, simple and compound Poisson processes, ®-stable processes, subordinated processes, ¯nancial processes, relativistic process, Riemann zeta process . ² L¶evyprocesses are simplest generic class of process which have (a.s.) continuous paths interspersed with random jumps of arbitrary size oc- curring at random times. ² L¶evyprocesses comprise a natural subclass of semimartingales and of Markov processes of Feller type. ² Noise. L¶evyprocesses are a good model of \noise" in random dynamical systems. 1 Input + Noise = Output Attempts to describe this di®erentially leads to stochastic calculus.A large class of Markov processes can be built as solutions of stochastic di®erential equations driven by L¶evynoise. L¶evydriven stochastic partial di®erential equations are beginning to be studied with some intensity. ² Robust structure. Most applications utilise L¶evyprocesses taking val- ues in Euclidean space but this can be replaced by a Hilbert space, a Banach space (these are important for spdes), a locally compact group, a manifold. Quantised versions are non-commutative L¶evyprocesses on quantum groups. Applications These include: ² Turbulence via Burger's equation (Bertoin) ² New examples of quantum ¯eld theories (Albeverio, Gottshalk, Wu) ² Viscoelasticity (Bouleau) ² Time series - L¶evydriven CARMA models (Brockwell) ² Finance ( a cast of thousands) The biggest explosion of activity has been in mathematical ¯nance. Two major areas of activity are: ² option pricing in incomplete markets. ² interest rate modelling. 2 1 Lecture 1: In¯nite Divisibility and L¶evy Processes 1.1 Some Basic Ideas of Probability Notation. Our state space is Euclidean space Rd. The inner product be- tween two vectors x = (x1; : : : ; xd) and y = (y1; : : : ; yd) is Xd (x; y) = xiyi: i=1 The associated norm (length of a vector) is à ! 1 d 2 1 X 2 2 jxj = (x; x) = xi : i=1 Let (­; F;P ) be a probability space, so that ­ is a set, F is a σ-algebra of subsets of ­ and P is a probability measure de¯ned on (­; F). Random variables are measurable functions X : ­ ! Rd. The law of X is pX , where for each A 2 F; pX (A) = P (X 2 A). (Xn; n 2 N) are independent if for all i1; i2; : : : ir 2 N;Ai1 ;Ai2 ;:::;Air 2 B(Rd), P (Xi1 2 A1;Xi2 2 A2;:::;Xir 2 Ar) = P (Xi1 2 A1)P (Xi2 2 A2) ¢ ¢ ¢ P (Xir 2 Ar): If X and Y are independent, the law of X + Y is given by convolution of measures Z pX+Y = pX ¤ pY ; where (pX ¤ pY )(A) = pX (A ¡ y)pY (dy): Rd Equivalently Z Z Z g(y)(pX ¤ pY )(dy) = g(x + y)pX (dx)pY (dy); Rd Rd Rd d d for all g 2 Bb(R ) (the bounded Borel measurable functions on R ). If X and Y are independent with densities fX and fY , respectively, then X + Y has density fX+Y given by convolution of functions: Z fX+Y = fX ¤ fY ; where (fX ¤ fY )(x) = fX (x ¡ y)fY (y)dy: Rd 3 d The characteristic function of X is ÁX : R ! C, where Z i(u;x) ÁX (u) = e pX (dx): Rd Theorem 1.1 (Kac's theorem) X1;:::;Xn are independent if and only if à à !! Xn E exp i (uj;Xj) = ÁX1 (u1) ¢ ¢ ¢ ÁXn (un) j=1 d for all u1; : : : ; un 2 R . More generally, the characteristic function of a probability measure ¹ on Rd is Z i(u;x) Á¹(u) = e ¹(dx): Rd Important properties are:- 1. Á¹(0) = 1. P 2. Á¹ is positive de¯nite i.e. i;j cic¹jÁ¹(ui ¡ uj) ¸ 0, for all ci 2 C; ui 2 Rd; 1 · i; j · n; n 2 N. 3. Á¹ is uniformly continuous - Hint: Look at jÁ¹(u + h) ¡ Á¹(u)j and use dominated convergence)). Conversely Bochner's theorem states that if Á : Rd ! C satis¯es (1), (2) and is continuous at u = 0, then it is the characteristic function of some probability measure ¹ on Rd. à : Rd ! C is conditionally positive de¯nite if for all n 2 N and Pn c1; : : : ; cn 2 C for which j=1 cj = 0 we have Xn cjc¹kÃ(uj ¡ uk) ¸ 0; j;k=1 d d for all u1; : : : ; un 2 R . à : R ! C will be said to be hermitian if Ã(u) = Ã(¡u), for all u 2 Rd. Theorem 1.2 (Schoenberg correspondence) à : Rd ! C is hermitian and conditionally positive de¯nite if and only if età is positive de¯nite for each t > 0. 4 Proof. We only give the easy part here. Suppose that età is positive de¯nite for all t > 0. Fix n 2 N and choose c1; : : : ; cn and u1; : : : ; un as above. We then ¯nd that for each t > 0, 1 Xn c c¹ (etÃ(uj ¡uk) ¡ 1) ¸ 0; t j k j;k=1 and so Xn 1 Xn tÃ(uj ¡uk) cjc¹kÃ(uj ¡ uk) = lim cjc¹k(e ¡ 1) ¸ 0: t!0 t j;k=1 j;k=1 ¤ 5 1.2 In¯nite Divisibility We study this ¯rst because a L¶evyprocess is in¯nite divisibility in motion, i.e. in¯nite divisibility is the underlying probabilistic idea which a L¶evy process embodies dynamically. Let ¹ be a probability measure on Rd. De¯ne ¹¤n = ¹ ¤ ¢ ¢ ¢ ¤ ¹ (n times). We say that ¹ has a convolution nth root, if there exists a probability measure 1 1 ¤n ¹ n for which (¹ n ) = ¹. ¹ is in¯nitely divisible if it has a convolution nth root for all n 2 N. In 1 this case ¹ n is unique. Theorem 1.3 ¹ is in¯nitely divisible i® for all n 2 N, there exists a proba- bility measure ¹n with characteristic function Án such that n Á¹(u) = (Án(u)) ; d 1 for all u 2 R . Moreover ¹n = ¹ n . Proof. If ¹ is in¯nitely divisible, take Án = Á 1 . Conversely, for each ¹ n n 2 N, by Fubini's theorem, Z Z i(u;y1+¢¢¢+yn) Á¹(u) = ¢ ¢ ¢ e ¹n(dy1) ¢ ¢ ¢ ¹n(dyn) ZRd Rd i(u;y) ¤n = e ¹n (dy): Rd R i(u;y) But Á¹(u) = Rd e ¹(dy) and Á determines ¹ uniquely. Hence ¹ = ¤n ¹n : ¤ - If ¹ and º are each in¯nitely divisible, then so is ¹ ¤ º. w - If (¹n; n 2 N) are in¯nitely divisible and ¹n ) ¹, then ¹ is in¯nitely divisible. w [Note: Weak convergence. ¹n ) ¹ means Z Z lim f(x)¹n(dx) = f(x)¹(dx); n!1 Rd Rd d for each f 2 Cb(R ).] A random variable X is in¯nitely divisible if its law pX is in¯nitely di- d (n) (n) (n) (n) visible, e.g. X = Y1 + ¢ ¢ ¢ + Yn , where Y1 ;:::;Yn are i.i.d., for each n 2 N. 6 1.2.1 Examples of In¯nite Divisibility In the following, we will demonstrate in¯nite divisibility of a random variable (n) (n) d (n) (n) X by ¯nding i.i.d. Y1 ;:::;Yn such that X = Y1 + ¢ ¢ ¢ + Yn , for each n 2 N. Example 1 - Gaussian Random Variables Let X = (X1;:::;Xd) be a random vector. We say that it is (non ¡ degenerate)Gaussian if it there exists a vector m 2 Rd and a strictly positive-de¯nite symmetric d £ d matrix A such that X has a pdf (probability density function) of the form:- µ ¶ 1 1 ¡1 f(x) = d p exp ¡ (x ¡ m; A (x ¡ m)) ; (1.1) (2¼) 2 det(A) 2 for all x 2 Rd. In this case we will write X » N(m; A). The vector m is the mean of X, so m = E(X) and A is the covariance matrix so that A = E((X ¡m)(X ¡m)T ). A standard calculation yields 1 Á (u) = exp fi(m; u) ¡ (u; Au)g; (1.2) X 2 and hence ½ ³ ´ µ ¶¾ 1 m 1 1 (Á (u)) n = exp i ; u ¡ u; Au ; X n 2 n (n) m 1 so we see that X is in¯nitely divisible with each Yj » N( n ; n A) for each 1 · j · n. We say that X is a standard normal whenever X » N(0; σ2I) for some σ > 0. We say that X is degenerate Gaussian if (1.2) holds with det(A) = 0, and these random variables are also in¯nitely divisible. Example 2 - Poisson Random Variables In this case, we take d = 1 and consider a random variable X taking values in the set n 2 N [ f0g. We say that is Poisson if there exists c > 0 for which cn P (X = n) = e¡c: n! In this case we will write X » ¼(c). We have E(X) = Var(X) = c. It is easy to verify that iu ÁX (u) = exp[c(e ¡ 1)]; (n) c from which we deduce that X is in¯nitely divisible with each Yj » ¼( n ), for 1 · j · n; n 2 N. 7 Example 3 - Compound Poisson Random Variables Let (Z(n); n 2 N) be a sequence of i.i.d.
Details
-
File Typepdf
-
Upload Time-
-
Content LanguagesEnglish
-
Upload UserAnonymous/Not logged-in
-
File Pages30 Page
-
File Size-