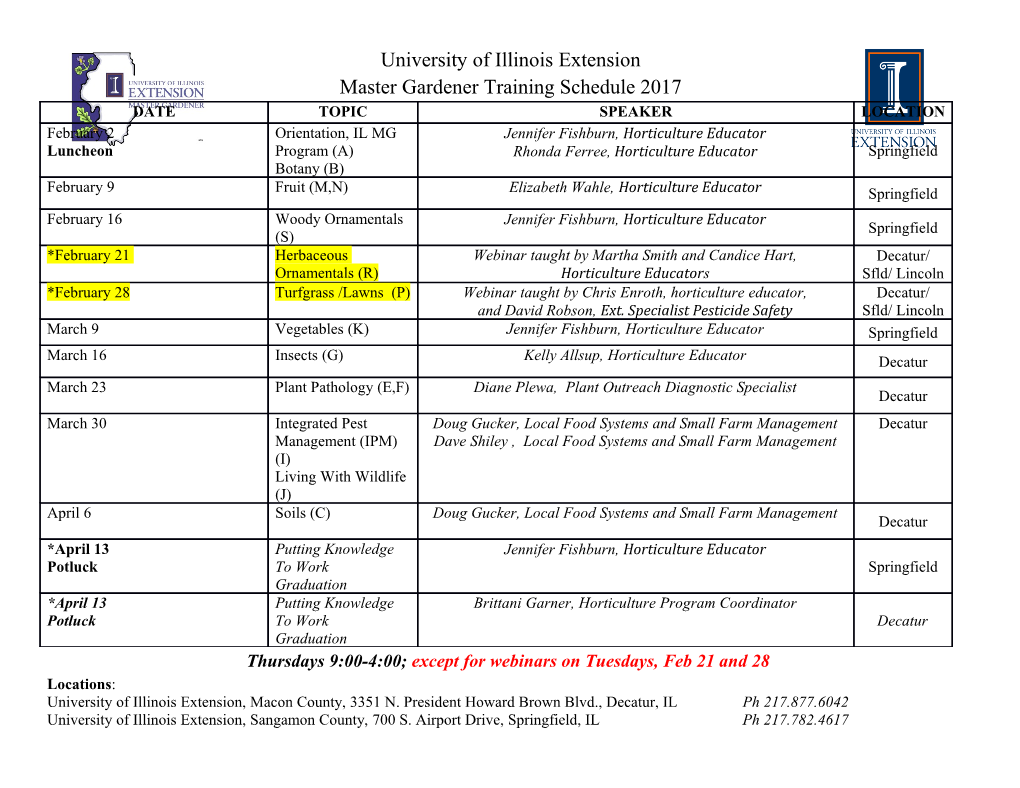
www.nature.com/scientificreports OPEN Disease biomarker identifcation from gene network modules for metastasized breast cancer Received: 25 January 2017 Pooja Sharma1, Dhruba K. Bhattacharyya1 & Jugal Kalita2 Accepted: 21 March 2017 Published: xx xx xxxx Advancement in science has tended to improve treatment of fatal diseases such as cancer. A major concern in the area is the spread of cancerous cells, technically refered to as metastasis into other organs beyond the primary organ. Treatment in such a stage of cancer is extremely difcult and usually palliative only. In this study, we focus on fnding gene-gene network modules which are functionally similar in nature in the case of breast cancer. These modules extracted during the disease progression stages are analyzed using p-value and their associated pathways. We also explore interesting patterns associated with the causal genes, viz., SCGB1D2, MET, CYP1B1 and MMP9 in terms of expression similarity and pathway contexts. We analyze the genes involved in both the stages– non metastasis and metastatsis and change in their expression values, their associated pathways and roles as the disease progresses from one stage to another. We discover three additional pathways viz., Glycerophospholipid metablism, h-Efp pathway and CARM1 and Regulation of Estrogen Receptor, which can be related to the metastasis phase of breast cancer. These new pathways can be further explored to identify their relevance during the progression of the disease. A normal cell follows a well-known path of growth, divison and death although there are exceptions. Some cells do not die. Tey keep on dividing again and again, leading to abnormal growth. Such condition of cells is called cancerous, and it corresponds to a class of diseases known as cancer. Unrestricted growth of cells leads to the formation of lumps known as tumors, which can interfere in activities of bodily systems such as the nevous sys- tem or the circulatory system. Tese lumps of cells can be benign or malignant. Benign tumors do not disturb the normal functioning of the body. Tey are still usually controlled by genes controlling apoptosis. On the other hand, malignant tumor cells invade organs, thereby disrupting their normal functioning. Tey can also move throughout the body using the blood or the lymphatic system as the channel and invade other parts. Such spread of the disease is referred to as the metastatic stage. When cancerous cells metastasize, it becomes very difcult to treat them. Te current characterization of this crucial stage of the disease is incomplete and as a result, proper diagnosis is ofen not feasible. Certain studies such as1–3 have analyzed diferentially expressed genes for meta- static stages of lymphoma, lung cancer and leukaemia. Veer et al.4 and Wang et al.5 have identifed 70 genes that may be associated with the metastatic stage of breast cancer. Chuang et al.6 use a protein-protein interaction network to determine the markers in the metastasis stage. All these studies focus on extracting individual disease markers responsible for disease progression. However, studies have shown that these genes may be associated with a variety of functions in the body, and interplay among the genes may lead to disorders that fnally lead to cancer. Terefore, it would be benefcial to study the disease progression from the pathway point of view. Tis is because a biological pathway involves a number of molecules simultaneously, and these molecules have to work in coordination to perform normal cellular activities. A slight change in any of the molecules may lead others in the chain to behave abnormally, resulting in disease diagnosis. Treating cancer for the non-metastatic stage is possible by drugs or by chemotherapy, but if the disease has spread to other organs, it becomes very difcult to diagnose on time and accordingly provide treatment. In this paper, we use the biological similarity between genes to identify functionally related modules. Gene expression data only consider the preparatory information available during the experiments. Analyzing the modules using only a part of the laboratory information would not be justifable as genes are known to be functionally correlated in nature. Terefore, we use both topological and functional properties of genes to fnd biologically signifcant 1epur niersit omputer cience an nineerin Dept epur ssam 78408 Inia. Department of omputer cience niersit of oorao oorao prins nite tates. orresponence an reuests for materias sou e aresse to D... emai: teu.ernet.in) Scientific RepoRts | 7: 1072 | DOI:10.1038/s41598-017-00996-x 1 www.nature.com/scientificreports/ SST = 0.3 SST = 0.5 SST = 0.7 SST = 0.3 SST = 0.5 SST = 0.7 SST = 0.3 SST = 0.5 SST = 0.7 Modules CCT = 0.3 CCT = 0.3 CCT = 0.3 CCT = 0.5 CCT = 0.5 CCT = 0.5 CCT = 0.7 CCT = 0.7 CCT = 0.7 M1 5.41E-6 2.25E-5 5.41E-6 4.14E-6 5.41E-6 5.10E-7 5.41E-6 2.25E-5 5.41E-6 M2 3.41E-5 3.41E-5 4.14E-6 5.41E-6 3.41E-5 7.14E-7 3.41E-5 1.56E-5 3.41E-5 M3 6.25E-5 1.68E-4 3.41E-5 3.41E-5 2.25E-5 8.41E-6 1.68E-4 1.68E-4 2.25E-5 Table 1. p–value of top 3 modules obtained using diferent thresholds in metastasis stage. Figure 1. Chemokine signalling pathway (source-http://www.kegg.jp/kegg/kegg1.html). modules. Finding modules with high coherence is very essential in our case, as the main target of this work is to analyze the diferent characteristics of genes including the causal genes of breast cancer from diferent per- spective. Such an analysis incorporating evidence from published literature can be used by biologists and other researchers to carry forward their work on these genes and their associated features. Tis work provides a comprehensive study of biomarkers associated with breast cancer. In this paper, we make the following contributions. t We propose an efective gene-gene network module extraction technique from micro-array gene expression data. We use both the toplogical and functional characteristics of the gene networks to fnd highly enriched modules. t We analyze members of these modules from diferent perspectives, eg., expression patterns and pathways. t We identify relevant pathways by analyzing modules for both the stages. Such pathways provide an insight into the role of each gene in the body and can be further extended to identify its role during disease progression. t We discover certain interesting characteristics of causal genes such as MET and WARS. t We fnd three more pathways to be associated with the metastasis stage of the disease. Tese pathways can be further explored to fnd their role in progression of the disease. Experimental Results We implemented our network construction and module extraction method in MATLAB running on an HP Z 800 workstation with two 2.4 GHz Intel(R) Xeon(R) processors and 12 GB RAM, using the Windows7 operating system. The semantic similarity for a given pair of genes is found using the GOSemSim package7 in R. Parameter tuning for p-value computation. We carried out our experiments at various CCT and SST thresholds. CCT and SST are a clustering coefcient and a semantic similarity threshold respectively, set by the Scientific RepoRts | 7: 1072 | DOI:10.1038/s41598-017-00996-x 2 www.nature.com/scientificreports/ Non-metastasis modules Pathway associated Module No.: Members Pathways gene names p-value antigen processing and presentation CTSS purine metabolism PDE4B cytokine-cytokine receptor interaction, chemokine signalling pathway, NOD-like receptor signaling pathway, cytosolic DNA-sensing pathway, CCL5 toll-like receptor signaling pathway. cytokine-cytokine receptor interaction, chemokine signalling pathway, NOD-like receptor signaling pathway, cytosolic DNA-sensing pathway, CXCL10 1: SRGN, MX1, GBP1, PLEK, toll-like receptor signaling pathway, RIG-I-like receptor signaling pathway PDE4B, SLA, IL32, CXCL9, RUNX3, p53 signaling pathway, Wnt signaling pathway, focal adhesion, Jak-STAT IFI44L, CD52, LRMP, TRBV19, CCND2 signaling pathway, cyclins and cell cycle regulation 5.38E-6 CTSS, PDE4B, CCL5, CXCL10, CCND2, CYP1B1, WARS, MMP9, steroid hormone biosynthesis CYP1B1 PFKP, TAP1, ARHGAP4, SLC2A3 tryptophan metabolism, Aminoacyl-tRNA biosynthesis WARS leukocyte transendothelial migration, pathways in cancer MMP9 glycolysis/gluconeogenesis pentose phosphate pathway, fructose and PFKP mannose metabolism, galactose metabolism antigen processing and presentation TAP1 Rho cell motility signaling pathway ARHGAP4 facilitated glucose transporter SLC2A3 cytokine-cytokine receptor interaction, chemokine signalling pathway, NOD-like receptor signaling pathway, cytosolic DNA-sensing pathway, CCL5 toll-like receptor signaling pathway. 2: CCL5, CCND2, WARS, LAG3 3.08E-5 p53 signaling pathway, Wnt signaling pathway, focal adhesion, Jak-STAT CCND2 signaling pathway, cyclins and cell cycle regulation tryptophan metabolism, aminoacyl-tRNA biosynthesis WARS cytokine-cytokine receptor interaction, chemokine signalling pathway, NOD-like receptor signaling pathway, cytosolic DNA-sensing pathway, CCL5 toll-like receptor signaling pathway. 3: CCL5, CCND2, WARS, IGK@, 1.68E-4 IGLV5-45 p53 signaling pathway, Wnt signaling pathway, focal adhesion, Jak-STAT CCND2 signaling pathway, cyclins and cell cycle regulation tryptophan metabolism, aminoacyl-tRNA biosynthesis WARS cytokine-cytokine receptor interaction, chemokine signalling pathway, NOD-like receptor signaling pathway, cytosolic DNA-sensing pathway, CCL5 toll-like receptor signaling pathway. 4: CCL5, CCND2, WARS, IGLV3-19 1.68E-4 p53 signaling pathway, Wnt signaling pathway, focal
Details
-
File Typepdf
-
Upload Time-
-
Content LanguagesEnglish
-
Upload UserAnonymous/Not logged-in
-
File Pages11 Page
-
File Size-