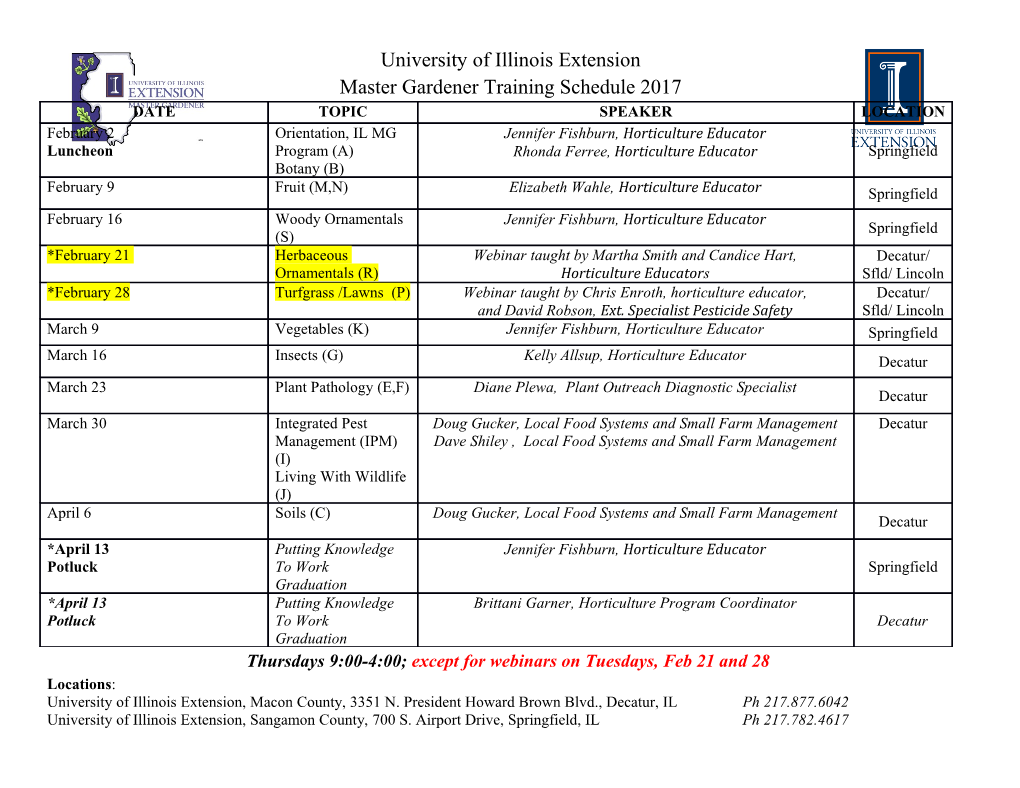
Assortativity of links in directed networks Mahendra Piraveenan1, Kon Shing Kenneth Chung1, and Shahadat Uddin1 1Centre for Complex Systems Research, Faculty of Engineering and IT, The University of Sydney, NSW 2006, Australia Abstract— Assortativity is the tendency in networks where many technological and biological networks are slightly nodes connect with other nodes similar to themselves. De- disassortative, while most social networks, predictably, tend gree assortativity can be quantified as a Pearson correlation. to be assortative in terms of degrees [3], [5]. Recent work However, it is insufficient to explain assortative or disassor- defined degree assortativity for directed networks in terms tative tendencies of individual links, which may be contrary of in-degrees and out-degrees, and showed that an ensemble to the overall tendency in the network. In this paper we of definitions are possible in this case [6], [7]. define and analyse link assortativity in the context of directed It could be argued, however, that the overall assortativity networks. Using synthesised and real world networks, we tendency of a network may not be reflected by individual show that the overall assortativity of a network may be due links of the network. For example, it is possible that in a to the number of assortative or disassortative links it has, social network, which is overall assortative, some people the strength of such links, or a combination of both factors, may maintain their ‘fan-clubs’. In this situation, people who which may be reinforcing or opposing each other. We also have a great number of friends may be connected to people show that in some directed networks, link assortativity can who are less famous, or even loners. If there is a link which be used to highlight subnetworks which have vastly different connects such a popular person with a loner, that link cannot topological structures. The quantity we propose is limited be called an assortative link, even though the network is to directed networks and complements the earlier proposed overall assortative. Similarly, in a network which is overall metric of node assortativity. disassortative many links could arguably be assortative. A good example for this is the so-called rich club scenario Keywords: Complex networks, graph theory, assortativity, social in internet AS networks [8]. Even though these networks networks, biological networks display overall disassortativity, it has been shown that the hubs among them are strongly connected to each other, 1. Introduction forming a ‘rich-club’. The links that connect these hubs, In the last few decades, network approaches have been therefore should be considered assortative in nature. These widely used to analyze complex systems in a number of examples highlight that the ‘local assortativity’ of links is a domains, including technical, biological, social and physical quantity, which, if defined, can throw light on the topological domains [1], [2]. Assortativity is a much studied concept in structure of networks. In directed networks, this can be the topological analysis of complex networks [3], [4], [5], defined separately for out-degree based mixing patterns and [6]. Assortativity has been defined to quantify the tendency in-degree based mixing patterns. in networks where individual nodes connect with other nodes Indeed, decomposing the assortativity coefficient of a which are similar to themselves [3]. Thus, a social network network into values for network components has already of people tends to be assortative, since people often prefer to been attempted, and the metric of ‘local assortativity’ has be friends with, or have links to, other people who are like been defined and analysed in detail [4], [6]. However, this them. A food web could be argued as disassortative, because decomposition has been done in terms of network nodes, predator and prey are unlikely to be similar in many respects. rather than network links. In this work however, we propose However, it is clear that the assortativity of a network needs to analyze the ‘local assortativity’ of individual links, and to be defined in terms of a particular attribute of nodes in will demonstrate that this approach has its advantages, that network. A social network could be assortative when particularly for defining the assortativity of sub networks the considered attribute is the age of people, because people or regions. We limit our analysis to directed networks. tend to be friends with other people similar to their age: Analyzing the ‘local assortativity’ of links can give us however, the same network could be disassortative, when a number of insights about networks. We will be able to the gender of individuals is the considered attribute. Degree identify ‘positive’ and ‘negative’ links in the network in assortativity is the most common form of assortativity used terms of assortativity, and based on these we can see which in network analysis, whereby similarity between nodes is links help, or hinder, the overall assortative tendency in defined in terms of the number of connections the nodes networks. We can identify if assortativity of a network is have. Degree assortativity can be defined and quantified primarily determined by the number of a particular type as a Pearson correlation [3], [7]. It has been shown that of links, or rather by the strength of such links. If the ‘strength’ of a minority of links is the primary reason for out-degrees. Therefore, we must consider the probability a network’s assortativity, then we could predict that, if the distribution of links going out of source nodes with j out- out network evolves, its assortativity may change rapidly. Link degrees, denoted as qj , and the probability distribution of in assortativity can be an indicator of the importance of links in links going into target nodes with k in-degrees, denoted qk . the network, particularly if the links are highly assortative. In addition, we may consider the probability distribution of Furthermore, profiles of link assortativity will provide us links going into target nodes with k out-degrees, denoted out with yet another tool to classify networks. q˘k , and the probability distribution of links going out of in Our paper is structured as follows: in the next section, source nodes with j in-degrees, denoted q˘j . In general, out 6 out in 6 in we will introduce the concept of link assortativity for di- qk =q ˘k and qj =q ˘j [6]. rected networks. We will use the definition of assortativity out;out We can also consider distribution ej;k , abbreviated as described in [7] to establish this concept, since this form of out ej;k , as the joint probability distribution of links going into definition is most conducive for link-based decomposition. target nodes with k out-degrees, and out of source nodes of Then we will analyze the link-based assortativity profiles of j out-degrees (i.e., the joint distribution in terms of out- a number of synthesized and real world directed networks. in in;in degrees). Similarly, ej;k = ej;k is the joint probability These include citation networks, Gene regulatory networks, distribution of links going into target nodes of k in-degrees, transcription networks, foodwebs and neural networks. We and out of source nodes of j in-degrees (i.e., the joint will draw observations from this analysis, showing that a distribution of in-degrees). network could be either assortative, disassortative or non- we can therefore define the out-assortativity of directed assortative, due to a number of combinations between the networks, as the tendency of nodes with similar out-degrees ratio of ‘positive’ and ‘negative’ links, and the average to connect to each other. Using the above distributions, out- strength of such links. We will also look at link-assortativity assortativity is formally defined, for out-degrees j and k, by distributions of a number of networks, and discuss what Piraveenan et al [6] as insights can be gained from these about the evolution and functionality of these networks. Finally we will present our 20 1 3 X summary and conclusions. 1 r = 4@ jkeoutA − µoutµout5 (1) out σoutσout j;k q q˘ q q˘ jk 2. Link assortativity of directed net- out out out out where µq is the mean of qk , µq˘ is the mean of q˘k , σq works out out is the standard deviation of qk , and σq˘ is the standard out Degree assortativity has been defined by Newman, as a deviation of q˘k . Pearson correlation between the ‘expected degree’ distri- Similarly, Piraveenan et al. [6] defined in-assortativity as bution qk, and the ‘joint degree’ distribution ej;k [3]. The the tendency in a network for nodes with similar in-degrees expected degree distribution is the probability distribution of to connect to each other, and this was formally specified as: traversing the links of the network, and finding nodes with 20 1 3 degree k at the end of the links. Similarly, the ‘joint degree’ 1 X r = 4@ jkein A − µinµin5 (2) distribution is the probability distribution of an link having in σinσin j;k q q˘ degree j on one end and degree k on the other end. In the q q˘ jk undirected case, the normalized Pearson coefficient of ej;k where µin is the mean of qin, µin is the mean of q˘in, σin is q q k q˘ k q and k gives us the assortativity coefficient of the network, the standard deviation of qin, σin is the standard deviation r. k q˘ of q˘in. If a network has perfect assortativity (r = 1), then all k Meanwhile, Foster et al. [7] also defined assortativity of nodes connect only with nodes with the same degree. For directed networks in terms of the above distributions.
Details
-
File Typepdf
-
Upload Time-
-
Content LanguagesEnglish
-
Upload UserAnonymous/Not logged-in
-
File Pages7 Page
-
File Size-