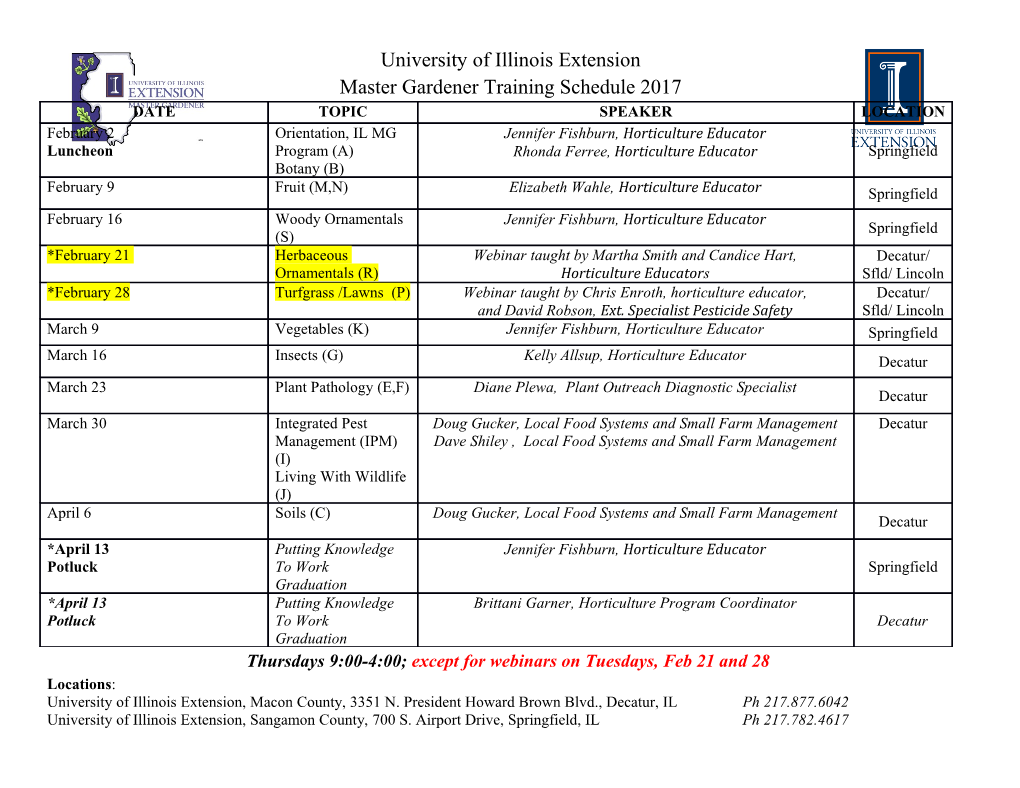
Outlier Detection and Robust Estimation in Nonparametric Regression Dehan Kong Howard Bondell Weining Shen University of Toronto University of Melbourne University of California, Irvine Abstract ical imaging and signal processing. In the presence of outliers, likelihood-based inference can be unreli- able. For example, ordinary least squares estimates This paper studies outlier detection and ro- for regression problems are highly sensitive to out- bust estimation for nonparametric regression liers. To facilitate valid statistical inference, an active problems. We propose to include a subject- area of research has been devoted to outlier detection specific mean shift parameter for each data and robust statistical estimation. Popular methods point such that a nonzero parameter will include M-estimators (Huber, 1981), Generalized M- identify its corresponding data point as an estimators (Mallows, 1975), least median of squares outlier. We adopt a regularization approach (Hampel, 1975), least trimmed squares (Rousseeuw, by imposing a roughness penalty on the re- 1984), S-estimators (Rousseeuw and Yohai, 1984), gression function and a shrinkage penalty on MM-estimators (Yohai, 1987), weighted least squares the mean shift parameter. An efficient algo- (Gervini and Yohai, 2002) and empirical likelihood rithm has been proposed to solve the double (Bondell and Stefanski, 2013). Although many of the penalized regression problem. We discuss a existing robust regression approaches enjoy nice the- data-driven simultaneous choice of two reg- oretical properties and satisfactory numerical perfor- ularization parameters based on a combina- mances, they usually focus on linear regression mod- tion of generalized cross validation and modi- els. While nonparametric regression models have been fied Bayesian information criterion. We show widely used in modern statistics, there is a consider- that the proposed method can consistently able gap in the literature on the extension of aforemen- detect the outliers. In addition, we obtain tioned methods to nonparametric regression problems, minimax-optimal convergence rates for both in which identifying outliers may be more challenging the regression function and the mean shift because outliers can be more easily associated with the parameter under regularity conditions. The majority of data via a nonparametric function than a estimation procedure is shown to enjoy the linear curve. There are a few robust nonparametric oracle property in the sense that the conver- estimation methods such as Cleveland (1979), Brown, gence rates agree with the minimax-optimal Cai, and Zhou (2008) and Cai and Zhou (2009). How- rates when the outliers (or regression func- ever, these methods can only estimate the nonpara- tion) are known in advance. Numerical re- metric function, and none of them can be applied to sults demonstrate that the proposed method outlier detection. has desired performance in identifying out- liers under different scenarios. In this paper, we fill in this gap by considering out- lier detection and robust estimation simultaneously for nonparametric regression problems. We use univariate 1 Introduction regression yi = f(xi) + i as an illustrative example and propose to include a subject-specific mean shift Outliers are observations that deviate markedly from parameter in the model. In particular, we add an ad- the majority of data. They are commonly encountered ditional subject-specific term into the nonparametric in real data applications such as genomics, biomed- regression model, i.e. yi = f(xi) + γi + i, where a nonzero mean shift parameter γi indicates that the ith Proceedings of the 21st International Conference on Ar- observation is an outlier. Then the problem becomes tificial Intelligence and Statistics (AISTATS) 2018, Lan- estimation of the regression function, f and mean shift zarote, Spain. PMLR: Volume 84. Copyright 2018 by the parameters, γi's. This idea originates from Gannaz author(s). (2006); McCann and Welsch (2007); She and Owen Outlier Detection and Robust Estimation in Nonparametric Regression (2011) in the context of linear models, however, the bust estimation and outlier detection. In this pa- extension from linear model to nonparametric mod- per, we adopt a general penalized regression frame- els requires nontrivial effort and the results are much work by considering popular penalty functions such as more flexible and useful in practice. The proposed LASSO (Tibshirani, 1996) and smoothly clipped abso- method is not restricted to particular domains, but lute deviation (SCAD) (Fan and Li, 2001). The pro- in general applicable for a wide range of domains in- posed method also applies to multivariate nonpara- cluding univariate and multivariate data. The exten- metric regression and semi-parametric models (e.g., sion from univariate data to the multi-dimensional and partial linear models). A major challenge in extending high-dimensional data is discussed in Section 6. Ma- the previous work in linear models lies in accurate and teos and Giannakis (2012) proposed a robust estima- efficient estimation of both the nonparametric func- tion procedure based on a similar model as ours, how- tion and the mean shift parameters at the same time. ever, there are several key differences between our pa- In the literature, nonparametric estimation is usu- per and this reference. First, our algorithm is different ally achieved via a smoothing procedure, such as local and much faster. We only need to update γi's itera- polynomial smoothing (Fan and Gijbels, 1996), poly- tively, while Mateos and Giannakis (2012) need to iter- nomial splines (Hastie and Tibshirani, 1990), regres- atively update γi's and f. Second, outlier detection is sion splines (Agarwal and Studden, 1980) and smooth- an important goal of our paper, and we study the per- ing splines (Wahba, 1990; Gu, 2013). In this paper, we formance of the method in terms of outlier detection, adopt smoothing splines for the nonparametric func- while the reference only focused on robust function tion estimates, and propose an efficient algorithm to estimation. Third, we have a better tuning method. solve an optimization problem that involves selecting The tuning method in Mateos and Giannakis (2012) two different tuning parameters simultaneously. depends on an initial function fit, which is computa- The rest of the paper is organized as follows. Section 2 tionally much slower. More severely, their initial func- describes our methodology including the problem for- tion fit may not be robust and may result in a bad mulation, computational algorithm and tuning param- estimate of the error variance. As their tuning crite- eter selection. Section 3 discusses the convergence rate rion is based on the estimate of the error variance, the for our nonparametric estimates. We present some tuning parameters selected could be completely mis- simulation results to evaluate the finite-sample perfor- leading. Finally, we have investigated the asymptotic mance of the proposed method in Section 4. In Section theory of our method, which is not included in that 5, we apply our method to the baseball data. We dis- reference. cuss some extensions to multi-dimensional and high- Our theoretical studies are concerned with the con- dimensional models in Section 6 and conclude with sistency of outlier detection and the so-called \oracle some remarks in Section 7. A proof sketch of the the- property" of the estimators. Specifically, we define the orems is given in Section 8. \oracle estimate" of f as the one obtained given all the outliers are known. Then an estimator of f is said 2 Methodology to satisfy the oracle property if it possesses the same minimax-optimal convergence rate as the oracle esti- We consider a univariate nonparametric mean shift mate. The oracle property for mean shift parameter model as follows, estimators can be defined in a similar way. A major contribution of our paper is that we derive sufficient yi = f(xi) + γi + i; (1) conditions on the tuning parameters such that the esti- mators of f and γ satisfy the oracle property. In other where the covariate xi, lies in a bounded closed inter- words, our estimation procedure is not affected by the val on the real line, and i's are i.i.d random errors additional step of identifying the outliers. The main with mean 0 and finite second moment. We are inter- technique we use here is based on M¨ullerand van de ested in using mean shift parameters γi's as indicators Geer (2015) with modifications to accommodate the of the outliers in the nonparametric regression of yi mean shift parameter component in our case. given xi. More precisely, if γi 6= 0, then its corre- sponding subject i is an outlier. Similarly, if γi = 0, For mean shift regression models, regularization meth- subject i is a normal data point. Suppose we have n ods are commonly used to detect the outliers. For samples (xi; yi), and we assume the number of outliers example, McCann and Welsch (2007) considered an is less than dn=2e. In other words, less than half of L1 regularization. She and Owen (2011) imposed a the γi's are nonzero, and this assumption guarantees nonconvex penalty function on γi's to obtain a sparse identifiability of our model. solution. Kong et al. (2018) imposed an adaptive penalty function on γi's to obtain fully efficient ro- We consider a shrinkage approach for outlier detec- tion by pushing most of the γi's toward zero. Clearly, Dehan Kong, Howard Bondell, Weining Shen those nonzero estimates will represent the outliers we Notice that for a fixed γ, we have detect under the regression model. In addition to out- ^ T −1 T lier detection, we are also interested in robust esti- θ = (N N + λL) N (y − γ): (5) mation of the nonparametric function f(·). This is Thus, we can use the profiling idea to express the opti- achieved by adopting a smoothing spline technique mization problem strictly as a function of γ. In partic- with a roughness penalty, which is based on the second T −1 T ular, denote Hλ = N(N N + λL) N .
Details
-
File Typepdf
-
Upload Time-
-
Content LanguagesEnglish
-
Upload UserAnonymous/Not logged-in
-
File Pages9 Page
-
File Size-