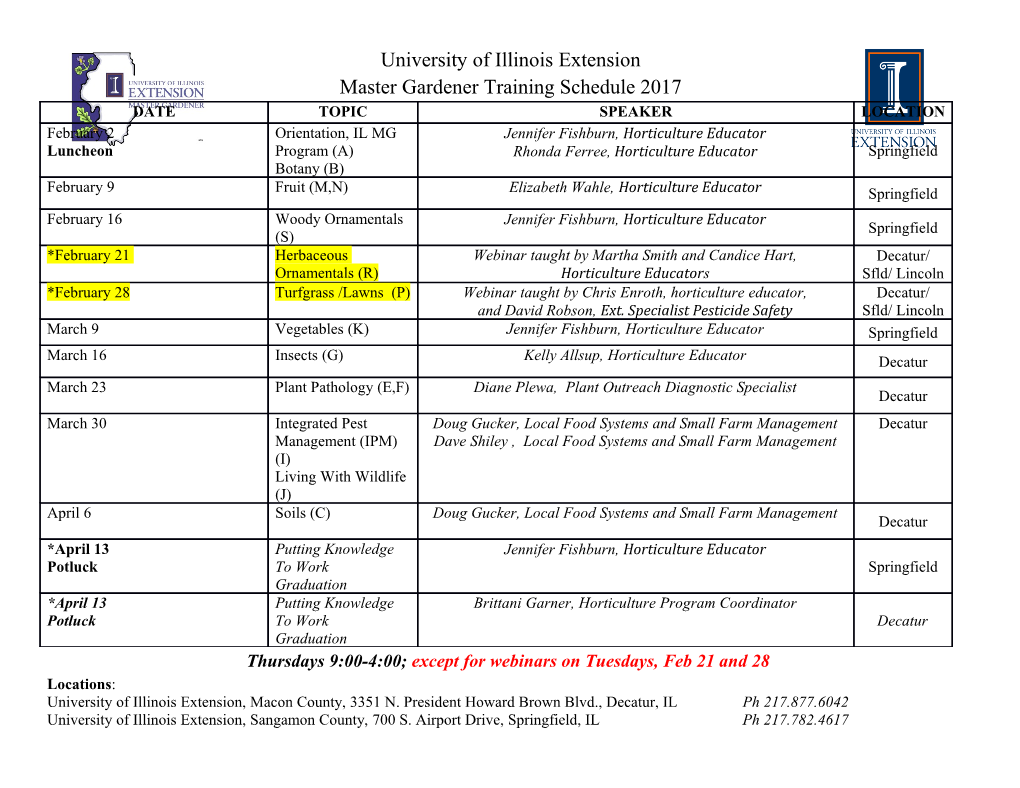
UNIVERSITY OF CALIFORNIA, SAN DIEGO Accurate Prediction of Causative Protein Kinase Polymorphisms in Inherited Disease and Cancer A Dissertation submitted in partial satisfaction of the Requirements for the degree Doctor of Philosophy in Biomedical Sciences by Ali Torkamani Committee in charge: Professor Nicholas Schork, Chair Professor Arshad Desai Professor Gerard Manning Professor Alexandra Newton Professor Susan Taylor Professor Anthony Wynshaw-Boris 2008 Copyright Ali Torkamani, 2008 All rights reserved. The Dissertation of Ali Torkamani is approved and it is acceptable in quality and form for publication on microfilm: Chair University of California, San Diego 2008 iii DEDICATION I dedicate this dissertation to my loving mother, Mitra Moassessi, and father, Naser Torkamani, whose love, support, and encouragement made this work possible. iv EPIGRAPH Dreaming when Dawn's Left Hand was in the Sky I heard a Voice within the Tavern cry, "Awake, my Little ones, and fill the Cup Before Life's Liquor in its Cup be dry." Omar Khayyam v TABLE OF CONTENTS Signature Page………………………………………………………………... iii Dedication…………………………………………………………………...... iv Epigraph……………………………………………………………………..... v Table of Contents……………………………………………………………... vi List of Abbreviations………………………………………………………..... ix List of Figures………………………………………………………………… x List of Tables………………………………………………………………..... xiii Acknowledgements…………………………………………………………… xvi Vita………………………………………………………………………….... xviii Abstract……………………………………………………………………….. xix Introduction………………………………………………………………….... 1 Chapter 1……………………………………………………………………… 7 1.1 Summary……………………………………………………………..... 7 1.2 Introduction……………………………………………………………. 7 1.3 Methodology…………………………………………………………... 9 1.4 Results……………………………………………………………….… 11 1.41 Selection of Prediction Method…………………………………....... 11 1.4.2 Performance and Validation of the Prediction Model……………… 12 1.4.3 Comparison to Previous Methods………………………………....... 16 1.4.4 Contribution of the Attributes………………………………………. 18 1.4.5 Implementation…………………………………………………....... 19 1.5 Conclusions…………………………………………………………… 20 Chapter 2……………………………………………………………………… 27 2.1 Summary…………………………………………………………………. 27 2.2 Introduction………………………………………………………………. 27 2.3 Methodology…………………………………………………………....... 30 2.4 Results……………………………………………………………………. 33 2.4.1 Prediction of Known Drivers…………………………………………... 33 2.4.2 Agreement with Re-sequencing-based Predictions……………………. 35 2.4.3 Analyses of Cosmic Database…………………………………… 36 vi 2.4.4 Sub-domains Analyses…………………………………………………. 37 2.4.5 Predicted Drivers Occur At Sites Enriched in CASMS………………... 41 2.4.6 Driver Hotspots…………………………………………………............ 42 2.4.7 Pathway Analysis………………………………………………………. 45 2.5 Conclusions………………………………………………………………. 49 Chapter 3……………………………………………………………………… 55 3.1 Summary…………………………………………………………………. 55 3.2 Introduction………………………………………………………………. 55 3.3 Methodology…………………………………………………………....... 58 3.4 Results……………………………………………………………………. 61 3.4.1 Distribution of Disease causing vs. Common SNPs…………………… 61 3.4.2 The Substrate Binding C-Lobe is Enriched in Disease SNPs………….. 62 3.4.3 Sub-domain I…………………………………………………………... 66 3.4.4 Sub-domain III-IV……………………………………………………... 67 3.4.5 Sub-domain VII………………………………………………………... 68 3.4.6 Sub-domain VIII……………………………………………………….. 72 3.4.7 Sub-domains IX-XII……………………………………………............ 72 3.4.8 Sub-domain IX…………………………………………………………. 74 3.4.9 Sub-domain X………………………………………………………….. 75 3.4.10 Sub-domains XI-XII………………………………………………….. 76 3.5 Detailed Results………………………………………………………….. 77 3.6 Physiochemical Attributes of Disease Causing Mutations………………. 91 3.7 Protein Flexibility……………………………………………………....... 95 3.8 Solvent Accessibility…………………………………………………….. 99 3.9 Conclusions………………………………………………………………. 101 Chapter 4……………………………………………………………………… 106 4.1 Summary…………………………………………………………………. 106 4.2 Introduction………………………………………………………………. 106 4.3 Methodology…………………………………………………………....... 108 4.4 Results……………………………………………………………………. 111 4.4.1 SNP Identification……………………………………………………... 111 4.4.2 Evolutionary Analysis Via the Panther Database………………............ 113 4.4.3 Group Analysis…………………………………………………............ 114 4.4.4 Domain Analysis………………………………………………............. 115 4.4.5 Amino Acid Analysis………………………………………………….. 117 4.4.6 Amino Acid Changes…………………………………………………... 119 4.4.7 Nucleotide Analysis……………………………………………………. 120 4.4.8 Integrated Analysis…………………………………………………….. 121 4.4.9 Conservation vs. Structural Analysis…………………………………... 123 4.4.10 Comparison with Mouse Kinase nsSNPs…………………………….. 125 4.4.11 Secondary Structure Analyses………………………………………... 125 4.4.12 Solvation Analyses…………………………………………………… 131 vii 4.4.13 Residue Volumen Analyses…………………………………………... 139 4.4.14 Amino Acid – Structural Interaction Analyses……………………….. 142 4.4.15 Integrated Structural Analysis………………………………………... 144 4.5 Conclusions………………………………………………………………. 145 Appendices……………………………………………………………………. 153 Appendix A……………………………………………………………....... 153 Appendix B………………………………………………………………... 160 Appendix C……………………………………………………………....... 243 References…………………………………………………………………….. 258 viii LIST OF ABBREVIATIONS SNPs – single nucleotide polymorphisms nsSNPs – nonsynonymous single nucleotide polymorphisms DC – disease causing uDC – unknown to be disease causing AUC – area under the curve SVM – support vector machine CASM – cancer associated mutation ix LIST OF FIGURES Figure 1.1 Performance of the Prediction Model: ROC curves generated from training and testing using on the natural and experimental set. Corresponding measures of accuracy are presented in Table 1.2, and areas under the curves are presented in Table 1.3. The curves represented are: …. 13 Figure 1.2 Tree Diagram Demonstrating Accuracy of Results: Tree diagram depicting separation of disease and nondisease SNPs. Distances are either unweighted (left) or weighted with the SVM coefficients (Right). Disease SNPs (DC) are shown in red, unknown to cause disease SNPs…………….. 16 Figure 1.3 Comparison of the Model to Previous Methods: Comparison of the Model, SubPSEC, SIFT, and PMUT methods of predicting disease status of mutations. The model outperforms other methods. Corresponding areas under the curve and statistical comparisons are presented in…………. 17 Figure 1.4 Contribution of the Attributes: Comparison of the performance of any SNP characteristic on disease prediction. Corresponding AUCs and statistical comparison are presented in Table 1.4. No single data type performs as well as the combined model. Group shows……………………. 19 Figure 2.1 Sub-domains Mapped to PKA: The sub-domains of PKA (PDB ID 1ATP) are colored and labeled by color-matched roman numerals……... 38 Figure 2.2 CASM and Driver Density Mapped to PKA: The sub-domains of PKA (PDB ID 1ATP) are colored depending on their CASM or Driver Density. CASM density is the ratio of expected to observed CASMs from Table 2.2 (left panel). Driver density is the percentage of CASMs………… 41 Figure 2.3 Position Specific Distribution of CASM and Driver SNPs: The position specific distribution of CASM and driver SNPs mapped to PKA (PDB ID 1ATP). The positions are colored by the number of SNPs per site (either CASMs or drivers). Note the preponderance of green CASM……… 43 Figure 2.4 Sub-domains and Driver Hotspot in EGFR: The sub-domains of EGFR are colored and labeled by color-matched roman numerals. The structure on the left represents EGFR in the active conformation (PDB ID: 2GS6), while the structure on the right represents EGFR in the inactive…... 44 Figure 3.1 Kinase Sub-Domains and SNP Distribution: (A) The sub- domains PKA (PDB ID 1ATP). Grey residues are intervening loops. Sub- domains are numbered by roman numerals and color coded. (B) The distribution of kinase disease SNPs…………………………………….…… 63 x Figure 3.2 of Disease and Common SNPs in N-lobe Sub-domains: The distribution of disease and common SNPs and the degree of conservation per residue in (A) sub-domain I and (B) sub-domain III-IV. Black bars = disease SNPs, Grey bars = Common SNPs. The character height………….. 67 Figure 3.3 Distribution of Disease and Common SNPs in Sub-domains VII and VIII: The distribution of disease and common SNPs and the degree of conservation per residue in (A) sub-domain VII and (B) sub-domain VIII. Black bars = disease SNPs, Grey bars = Common SNPs…………………… 70 Figure 3.4 Distribution of Disease and Common SNPs in C-lobe Sub- domains: The distribution of disease and common SNPs and degree of conservation per residue in (A) sub-domain IX, (B) sub-domain XII and (C) sub-domain X. Black bars = disease SNPs……………………………... 73 Figure 3.5 SNPs and Allostery: The ePK conserved allosteric network of the C-terminal lobe. Red balls = oxygen, blue balls = nitrogen, dashed lines = hydrogen bonds. Zoom box shows the ePK conserved side-chain network……………………………………………………………………… 76 Figure 3.6: Distribution of Disease and Common SNPs in Sub-domains XI: The distribution of disease and common SNPs and the degree of conservation per residue in sub-domain XI. Black bars = disease SNPs, Grey bars = Common SNPs. The character height is proportional…………. 77 Figure 3.7 Distribution of Disease and Common SNPs in Sub-domains II: The distribution of disease and common SNPs and the degree of conservation per residue in sub-domain II. Black bars = disease SNPs, Grey bars = Common SNPs. The character height is proportional……………… 79 Figure 3.8 Distribution of Disease and Common SNPs in Sub-domains V: The distribution of disease and common SNPs and the degree of conservation per residue in sub-domain V. Black bars = disease SNPs, Grey
Details
-
File Typepdf
-
Upload Time-
-
Content LanguagesEnglish
-
Upload UserAnonymous/Not logged-in
-
File Pages293 Page
-
File Size-