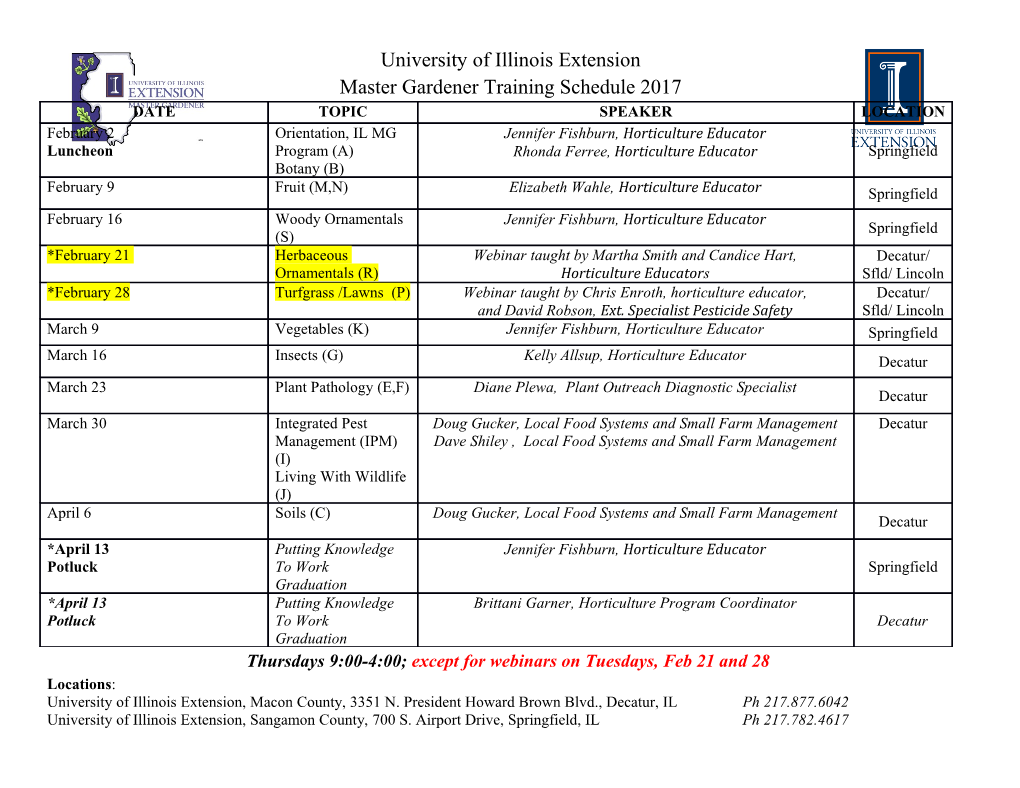
Math 217 x5.5 Professor Karen Smith Definition: An inner product on a vector space V is rule for combining two vectors f; g of V into a scalar hf; gi satisfying the following axioms: 1. hf; gi = hg; fi for all f; g 2 V (symmetric property) 1 2. hf1 + f2; gi = hf1; gi + hf2; gi for all f1; f2; g 2 V (linearity in first argument ) 3. hcf; gi = chf; gi for all f; g 2 V and scalars c (linearity in first argument2) 4. hf; fi ≥ 0 for all f 2 V with hf; fi = 0 if and only if f = 0 (positive definite property). A vector space equipped with an inner product is called an inner product space. Task: Your group is given one vector space, together with a rule for combining two vectors into a scalar. Investigate the following questions. 1. Is it an inner product space? Prove it, or adapt it to make it an inner product space. 2. Pick any non-zero vector in your inner product space. Find its length. 3. Find a pair of orthonormal vectors ~u1; ~u2 in your space. Let W be the space that they span. What is dim W ? 2 4. Compute the distance between ~u1 and ~u2 in your inner product. Also compute jj~u1 − ~u2jj . 5. What is the dimension of W ?? Find an element ~z in W ?. What is the closest vector in W to ~z? What is the projection of ~z onto W ? 6. Find an element ~q not in W [ W ?. Compute the projection of ~q to W . What is the closest vector in W to ~q? Z 1 A. Let C0 be the space of continuous functions. Let hf; gi be defined by fg dx: −1 2×2 T B. Let R be the space of 2 × 2 matrices. Define hA; Bi by trace (A B): 3 T C. Let V be R . Define h~x;~yi as the matrix product ~x A ~y where A is a diagonal matrix with 21 0 03 positive entries on the diagonal. For problems 2-6, take A = 40 2 05. 0 0 3 D. Let P2 be the space of polynomials of degree ≤ 2. Define hf; gi by f(−1)g(−1) + f(0)g(0) + f(1)g(1). 1 We also have linearity in the second argument: hf; g1 + g2i = hf; g1i + hf; g2i 8f; g1; g2 2 V but this follows from symmetry. 2We also have linearity in the second argument: hf; cgi = chf; gi 8f; g 2 V and scalars c but this follows from symmetry. Solution note: A. 1). Yes, this is an inner product. We check the four properties: Z 1 Z 1 1. Symmetry: hf; gi = hg; fi because fg dx = gf dx: −1 −1 Z 1 Z 1 Z 1 2. Linearity: hf1 + f2; gi = hf1; gi + hf2; gi because (f1 + f2)g dx = f1g dx + f2g dx: −1 −1 −1 Z 1 Z 1 3. Linearity: hcf; gi = chf; gi because (cf)g dx = c fg dx: −1 −1 Z 1 4. Positive definite: hf; fi = f 2 dx ≥ 0 because f 2 ≥ 0 everywhere on the interval [−1; 1]. −1 The only way for this integral to be zero is for f = 0|this uses the fact that f is CONTIN- UOUS, so there must be some interval where f is strictly positive if it is non-zero. You do not have to prove this fact|you will learn how to do so in Math 451. s Z 1 p 2). jj1jj = 1 dx = 2. −1 Z 1 3). Let f = 1 and g = x. Then fg dx = 0 since x is odd. So this is almost an −1 1=2 orthonormal basis: we need only scale each by its length. Computep jjxjj = hx; xi = 1 p R 2 1=2 1=2 x p3 x [ −1 x dx] = [2=3] . So let ~u1 = 1= 2 and ~u2 = jjxjj = : The dimension of p 2 1 3 x W is 2. It has orthonormal basis is (~u1; ~u2) = ( p ; p ): p 2 2 4). The distance between is 2. 5). The dimension of W ? is (very!) infinite. To find a vector z 2 W ?, we need a R 1 R 1 function z satisfying −1 zdx = 0 and −1 xzdx = 0. One way to do this is to take ANY vector, then subtract its projection to W . Take g = 3x2. The component in p 1 p p1 R 2 p2 the ~u1 direction has length hg; 1= 2i = −1 3x dx = = 2: The length of the 2 p 2 3 R 1 3 3 component in the ~u2 direction has hg; ~u2i = p 3x dx = 0 since x is odd. So p 2 −1 jj ? 2 ? g = 2~u1 + 0~u2 = 1. So g = g − 1 = 3x − 1 is vector in W . For any vector in W ?; including g = 3x2 − 1, the projection to W is zero. So the closest vector in W is zero as well. 6). For q, we can take the function g, it is neither in W nor W ?: Its projection onto W is hg; ~u i~u + hg; ~u i~u = [R 1 g~u dx]~u + [R 1 g~u dx]~u . In this case, for g = 3x2, p 1 1 2 2 −1 1 1 −1 2 2 we get 2~u1 + 0~u2 = 1. Solution note: B. 1.) This is an inner product. Check the properties. T T Symmetry: trace(A B) = trace(B A) because if we write A = [~a1 ~a2] and B = T ~ ~ T ~a1 ~ ~ ~ ~ [b1 b2], then A B = T [b1 b2] which has diagonal entries ~a1 · b1 and ~a2 · b2. Simi- ~a2 T larly, B A has diagonal entries ~a1 ·~b1 and ~a2 ·~b2, so the trace is the same. T T T T T Linearity: (A1 + A2) B = (A1 + A2 )B = A1 B + A2 B, so these have the same trace. SImilarly, (cA)T B = cAT B so the traces are the same. T 2 2 Positive definite: A A has trace ~a1 · ~a1 + ~a2 · ~a2 = jj~a1jj + jj~a2jj > 0 unless both ~a1 and ~a2 = 0.p 2. jjI2jj = 2. 3. E11;E22 is orthonormal. W has dimension 2. 0 1 p 2). The length of is p(trace(AT A)) = p(trace(I )) = 2: 1 0 2 3). Let vectors E11 and E22 are orthonormal, as you can easily check by computing hE11;E11i = 1; hE11;E22i = 0 and hE22;E22i = 1. The space they span has dimen- sion 2. s 1 0 p 4). jju − u jj = trace( )2 = 2. 1 2 0 −1 ? 5). The element E12 is in W . It's projection to W is zero. Thus zero is the closest ? vector in W to E12. The dimension of W is the dimension of the ambient space 2×2 R minus the dimension of W , or 4 − 2 = 2. 0 1 0 0 6). Let ~q = : The projection to W is : 1 1 0 1 Solution note: C. 1. For this we need A to have strictly positive entries on the diag- onal. Otherwise, it is not an inner product because positive definitivity fails. Symmetry: ~xT A~y = ~yT A~x because transposing, (~yT A~x)T = ~xT AT~(yT )T = ~xT A~y. Linearity follows from basic properties of matrix arithmetic. T 2 2 2 T Positive Definite: ~x A~x = x1d1 + x2d2 + : : : xndn where ~x = [x1 x2 : : : xn] . This is non-negative because the di are all positive. It is 0 if and only if all xi = 0. 2 3 1 0 0 p T 1=2 2. jj~e3jj = (~e3 40 2 05~e3) = 3: 0 0 3 3. ~e ; p1 ~e . W has dimension 2. 1 2 2 p 4). The distance between is 2. ? ? 5). The vector ~e3 2 W . For any vector in W ; the projection to W is zero. So the closest vector in W is zero as well. W ? has dimension 1, since dim W + dim W ? = 3; 3 which is the dimension of the ambient space R . 6). Let q = I2. Then the projection onto W is E11. Solution note: D. 1. This is obviously symmetric: hf; gi = hg; fi: To check linearlity: hf + h; gi = (f + h)(−1)g(−1) + (f + h)(0)g(0) + (f + h)(1)g(1) = f(−1)g(−1) + f(0)g(0) + f(1)g(1) + h(−1)g(−1) + h(0)g(0) + h(1)g(1) = hf; gi + hh; gi. To check positive definiteness, compute: hf; fi = (f(−1))2 + (f(0)2 + (f(1))20, which is non-negative, since it is a sum of squares. If hf; fi = 0 for some f = a + bx + cx2, then hf; fi = (c − b + a)2 + (a)+(a + b + c)2 = 0. Since squares are all positive, we conclude that (c − b + a) = a = (a + b + c). This means that a = 0; b + c = 0 and c − b = 0, so a = b − c = 0. So it is positive definite. p 2. The length of the polynomial x is hx; xi1=2 = ((−1)2 + 02 + (1)2)1=2 = 2: 3. Note that h1; xi = (1)(−1) + (1)(0) + (1)(−1)2 = 0, so that 1 and x are perpendicular. So we can getp an orthonormal basis by scaling each to be a unit vector. We already checked that jjxjj = 2, so px is a unit vector. Note also h1; 1i = 12 + 12 + 1=3, so p1 is a unit vector.
Details
-
File Typepdf
-
Upload Time-
-
Content LanguagesEnglish
-
Upload UserAnonymous/Not logged-in
-
File Pages4 Page
-
File Size-