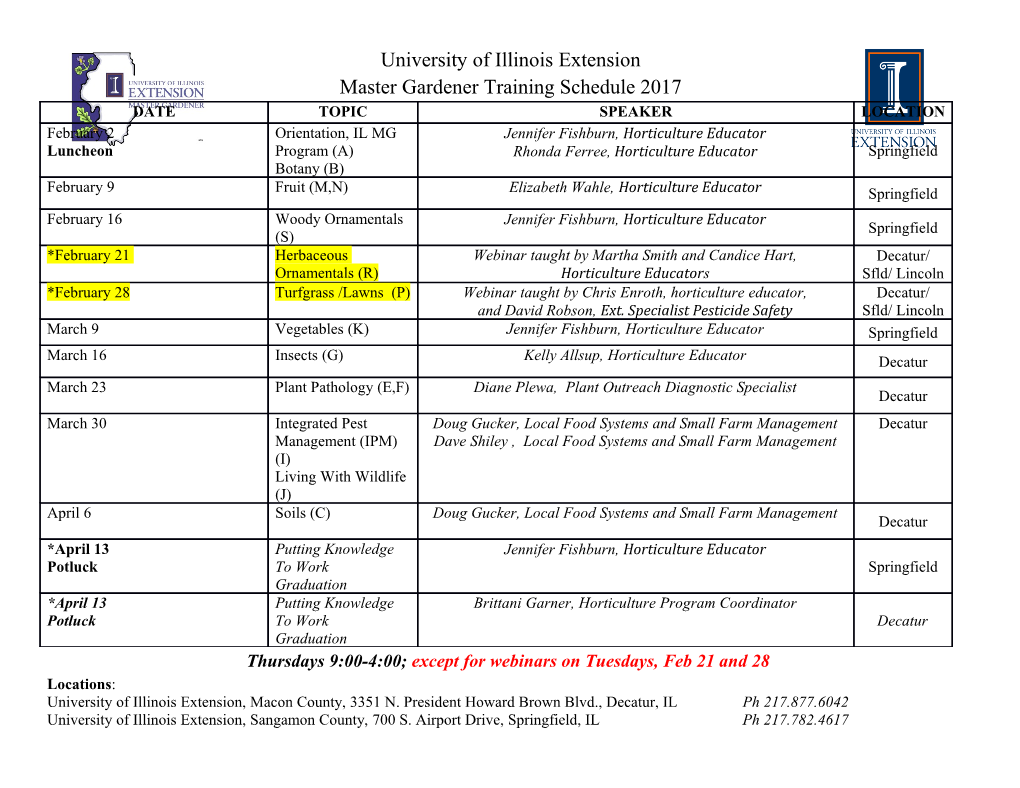
Sorting Algorithms Next to storing and retrieving data, sorting of data is one of the more common algorithmic tasks, with many different ways to perform it. Whenever we perform a web search and/or view statistics at some website, the presented data has most likely been sorted in some way. In this lecture and in the following lectures we will examine several different ways of sorting. The following are some reasons for investigating several of the different algorithms (as opposed to one or two, or the \best" algorithm). • There exist very simply understood algorithms which, although for large data sets behave poorly, perform well for small amounts of data, or when the range of the data is sufficiently small. • There exist sorting algorithms which have shown to be more efficient in practice. • There are still yet other algorithms which work better in specific situations; for example, when the data is mostly sorted, or unsorted data needs to be merged into a sorted list (for example, adding names to a phonebook). 1 Counting Sort Counting sort is primarily used on data that is sorted by integer values which fall into a relatively small range (compared to the amount of random access memory available on a computer). Without loss of generality, we can assume the range of integer values is [0 : m], for some m ≥ 0. Now given array a[0 : n − 1] the idea is to define an array of lists l[0 : m], scan a, and, for i = 0; 1; : : : ; n − 1 store element a[i] in list l[v(a[i])], where v is the function that computes an array element's sorting value. The sorted list can then be obtained by scanning the lists of l one-by-one in increasing order, and placing the encountered objects in a final list. Both steps require Θ(n) steps. Counting Sort is most commonly used on an array a of integers. One reason for this is that objects of a general type are often sorted on very large integers, which makes Counting Sort infeasible. For example, if an array of Employees are sorted based on social security number, then these values range in the tens of millions. When using an array of integers, l can be replaced by an integer array f, where f[i] represents the frequency of the number of elements of a that are equal to i. Example 1. Perform Counting Sort one the elements 9; 3; 3; 10; 5; 10; 3; 4; 9; 10; 1; 3; 5; 2; 4; 9; 9. 2 Radix Sort Radix Sort can be applied to an array of integers for which each integer is represented by k bits, and the time needed to access a single bit is O(1). The algorithm works in k stages. At the beginning of stage i, 1 ≤ i ≤ n, it is assumed that the integers are stored in some array. The elements are then scanned one-by-one, with elements having i th least significant bit equal to j (j = 0; 1) being placed in array bj (keeping the same order as in a). The round ends by rewriting a as the elements of b0 followed by the elements of b1. Assuming that k is held constant, the complexity of radix sort is Θ(kn) = Θ(n). Example 2. Perform Radix Sort on the elements 9; 13; 10; 5; 10; 3; 4; 9; 1; 5; 2. 3 Insertion Sort Insertion Sort represents a doubly iterative way of sorting data in an array. • Step 1. sort the first item in the array. • Step i + 1: assume the first i elements of the array are sorted. Move item i + 1 left to its appropriate location so that the first i + 1 items are sorted. Example 3. Use insertion sort on the array 43; 6; 72; 50; 44; 36; 21; 32; 47. 4 Code for Insertion Sort Applied to an Array of Integers: //Sorting in place the elements a[left:right]. void insertion_sort(int[] array, int left, int right) { int i, j,tmp; //Attempt to move element i to the left. for(i=left+1; i <= right; i++) { //Move left until finding the proper location of a[i]. for(j=i; j > left; j--) { if(a[j] < a[j-1]) { tmp = a[j-1]; a[j-1] = a[j]; a[j] = tmp; } else break; //found the right location for a[i] } } } 5 Average-Case Running Time of an Algorithm When analyzing the running time of an algorithm, sometimes we are interested in its average-case running time, denoted by Tave(n), where Tave(n) is the average of all the algorithm's running times over instances having size n. We now calculate the big-O average-case running time for Insertion Sort. We do this by assuming that each problem of size n is an array of n integers, where the integers are a permutation of the numbers 1; : : : ; n. Recall that an n-permutation is simply an ordered arrangement of the first n positive integers. In other words, if σ is an n-permutation, then we write σ = (σ(1) σ(2) ··· σ(n)); where σ(i) and σ(j) are positive integers less than or equal to n, and distinct if and only if i 6= j. Example 4. Provide the permutation associated with the unsorted array of Example 3. An inversion of a permutation σ is a pair (i; j) such that i < j and σ(j) < σ(i). For example, the permutation (1 4 5 3 6 2) has six inversions. 6 Observation. Every successful comparison of insertion sort reduces the number of inversions by 1. Thus, the number of steps (comparisons) needed for insertion sort is directly proportional to the number of inversions possessed by the array. n(n−1) Theorem 1. The average number of inversions possessed by a random permutation is 4 . n Proof of Theorem 1. Note that there are possible inversions of a permutation over n 2 numbers, and each inversion has a probability of 0:5 of appearing in a randomly generated permuta- n(n−1) tion of the numbers 1; : : : ; n. Thus, we would expect a random permutation to possess 4 such inversions. Corollary 1. The average-case running time for insertion sort is O(n2). n(n−1) Proof of Corollary 1. Since a swap removes only one inversion, and on average there are 4 = O(n2) inversions, it follows that the average-case running is O(n2). 7 Divide and Conquer Algorithms There exist many problems that can be solved using a divide-and-conquer algorithm. A divide-and- conquer algorithm A follows these general guidelines. Divide Algorithm A divides original problem into one or more subproblems of a smaller size. Conquer Each subproblem is solved by making a recursive call to A. Combine Finally, A combines the subproblem solutions into a final solution to the original problem. Some problems that can be solved using a divide-and-conquer algorithm: Binary Search locating an element in a sorted array Quicksort and Mergesort sorting an array Order Statistics finding the k th least or greatest element of an array Geometric Algorithms finding the convex hull of a set of points; finding two points that are closest. Matrix Operations matrix inversion, Fast-Fourier Transform, matrix multiplication, finding the largest submatrix of 1's in a Boolean matrix. Maximum Subsequence Sum finding the maximum sum of any subsequence in a sequence of integers. Minimum Positive Subsequence Sum finding the minimum positive sum of any subsequence in a sequence of integers. Multiplication finding the product of two numbers. 8 Example 5. Using x = 5 and array a = 1; 3; 3; 4; 6; 8:8:9:9:10; 11; 15; demonstrate how the Binary Search algorithm can be viewed as a divide-and-conquer algorithm. 9 Mergesort The Mergesort algorithm is a divide-and-conquer algorithm for sorting an array of comparable elements. The algorithm begins by checking if input array a has two or fewer elements. If so, then the a is sorted in place by making at most one swap. Otherwise, a is divided into two (almost) equal halves aleft and aright. Both of these subarrays are sorted by making recursive calls to Mergesort. Once sorted, a merge operation merges the elements of aleft and aright into an auxiliary array. This sorted auxiliary array is then copied over to the original array. Example 6. Demonstrate the Mergesort algorithm using the array 5; 8; 6; 2; 7; 1; 0; 9; 3; 4; 6. Theorem 2. Mergesort has a running time of T (n) = Θ(n log n). Proof Theorem 2. Let a be an array input of size n. The depth of Mergesort's recursion tree is Θ(log n). Moreover, for each element x of a, and for each depth i of the recursion tree, there is a sub-array a0 at depth i containing x, and for which the merge operation is applied. During this operation, Θ(1) steps are applied to x, For a total of Θ(n) total steps applied to all elements at depth i. Therefore, the algorithm's total steps is Θ(n)Θ(log n) = Θ(n log n). 10 Quicksort Before introducing the Quicksort algorithm, recall that the median of an array a of n numbers a[0]; : : : ; a[n − 1] is the (n + 1)=2 least element of a, if n is odd, and is equal to either the n=2 or n=2 + 1 least element of a if n is even (even-length arrays have two medians). Example 7.
Details
-
File Typepdf
-
Upload Time-
-
Content LanguagesEnglish
-
Upload UserAnonymous/Not logged-in
-
File Pages19 Page
-
File Size-