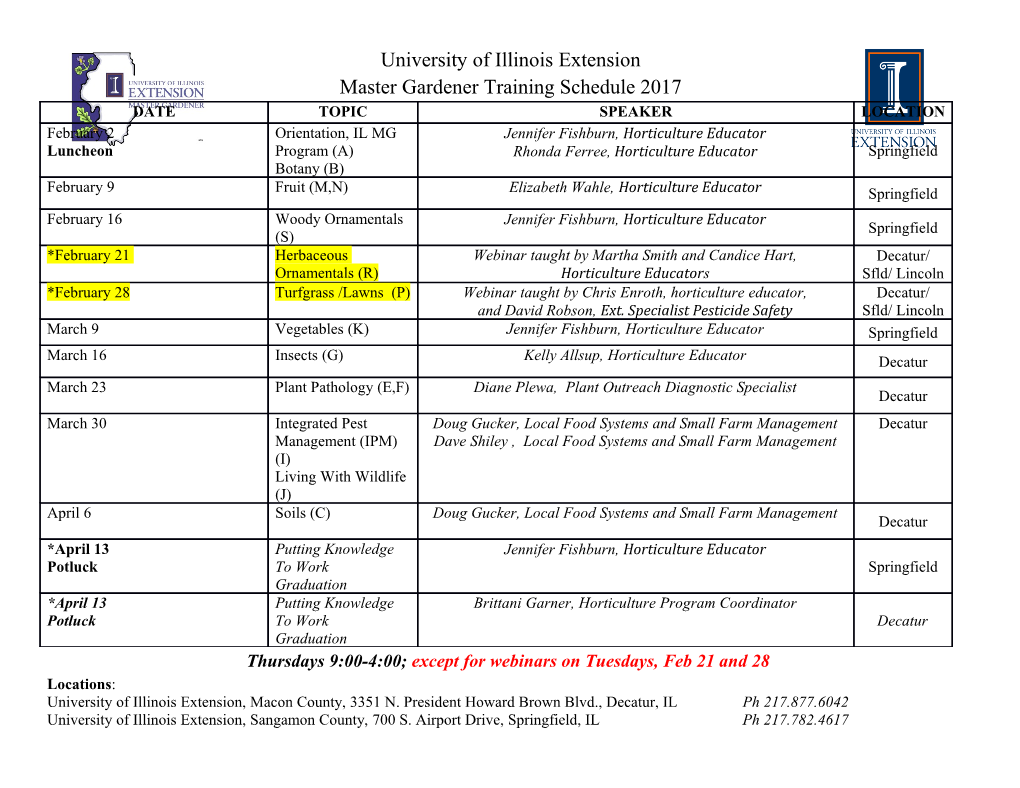
bioRxiv preprint Bioinformatics Analysis of Key Genes and Pathways for Obesity Associated Type 2 Diabetes Mellitus as a Therapeutic Target (which wasnotcertifiedbypeerreview)istheauthor/funder.Allrightsreserved.Noreuseallowedwithoutpermission. doi: https://doi.org/10.1101/2020.12.25.424383 Basavaraj Vastrad1, Anandkumar Tengli2, Chanabasayya Vastrad*3, Iranna Kotturshetti4 1. Department of Biochemistry, Basaveshwar College of Pharmacy, Gadag, Karnataka 582103, India. 2. Department of Pharmaceutical Chemistry, JSS College of Pharmacy, Mysuru and JSS Academy of Higher Education & Research, Mysuru, Karnataka, 570015, India 3. Biostatistics and Bioinformatics, Chanabasava Nilaya, Bharthinagar, Dharwad 580001, Karnataka, India. 4. Department of Ayurveda, Rajiv Gandhi Education Society`s Ayurvedic Medical College, Ron 562209, Karnataka, India. ; this versionpostedDecember27,2020. * Chanabasayya Vastrad The copyrightholderforthispreprint [email protected] Ph: +919480073398 Chanabasava Nilaya, Bharthinagar, Dharwad 580001 , Karanataka, India bioRxiv preprint Abstract (which wasnotcertifiedbypeerreview)istheauthor/funder.Allrightsreserved.Noreuseallowedwithoutpermission. doi: Obesity associated type 2 diabetes mellitus is one of the most common metabolic disorder worldwide. The prognosis of https://doi.org/10.1101/2020.12.25.424383 obesity associated type 2 diabetes mellitus patients has remained poor, though considerable efforts have been made to improve the treatment of this metabolic disorder. Therefore, identifying significant differentially expressed genes (DEGs) associated in metabolic disorder advancement and exploiting them as new biomarkers or potential therapeutic targets for metabolic disorder is highly valuable. Differentially expressed genes (DEGs) were screened out from gene expression omnibus (GEO) dataset (GSE132831) and subjected to GO and REACTOME pathway enrichment analyses. The protein - protein interactions network, module analysis, target gene - miRNA regulatory network and target gene - TF regulatory network were constructed, and the top ten hub genes were selected. The relative expression of hub genes was detected in RT-PCR. Furthermore, diagnostic value of hub genes in obesity associated type 2 diabetes mellitus patients was ; investigated using the receiver operating characteristic (ROC) analysis. Small molecules were predicted for obesity this versionpostedDecember27,2020. associated type 2 diabetes mellitus by using molecular docking studies. A total of 872 DEGs, including 439 up regulated genes and 432 down regulated genes were observed. Second, functional enrichment analysis showed that these DEGs are mainly involved in the axon guidance, neutrophil degranulation, plasma membrane bounded cell projection organization and cell activation. The top ten hub genes (MYH9, FLNA, DCTN1, CLTC, ERBB2, TCF4, VIM, LRRK2, IFI16 and CAV1) could be utilized as potential diagnostic indicators for obesity associated type 2 diabetes mellitus. The hub genes were validated in obesity associated type 2 diabetes mellitus. This investigation found effective and reliable molecular biomarkers for diagnosis and prognosis by integrated bioinformatics analysis, suggesting new and key therapeutic targets for obesity associated type 2 diabetes mellitus. The copyrightholderforthispreprint Keywords: biomarker; GEO; obesity associated type 2 diabetes mellitus; differentially expressed genes; pathways . bioRxiv preprint Introduction Obesity and type 2 diabetes mellitus are a major metabolic or endocrine disorder and are dramatically increasing throughout (which wasnotcertifiedbypeerreview)istheauthor/funder.Allrightsreserved.Noreuseallowedwithoutpermission. the globe [1]. The prevalence of obesity associated type 2 diabetes mellitus is 86% [2]. Obesity associated type 2 diabetes doi: mellitus might be responsible for causing cardiovascular diseases [3], hypertension [4], and neurological and https://doi.org/10.1101/2020.12.25.424383 neuropsychiatric disorders [5] and asthma [6]. Till today, there is no cure for obesity associated type 2 diabetes mellitus, and treatment and mediation tailored to clinical features are endorsed. Genetic and environmental factors are two initial contributors to this disorder [7]. Exploration of the molecular mechanisms of obesity associated type 2 diabetes mellitus will develop the considerate of its pathogenesis and has key implications for designing new therapy. Molecular mechanisms of obesity associated type 2 diabetes mellitus have been increasingly studied. Previous investigations showed that obesity associated type 2 diabetes mellitus in human was related to genes and signaling pathways. Key genes such as ENPP1 [8] and FTO [9] were responsible for development of obesity associated type 2 ; diabetes mellitus. Recent investigations showed that PI3K/AKT pathway [10] and TLR pathway [11] as a potential target this versionpostedDecember27,2020. for obesity associated type 2 diabetes mellitus. However, certain key genes and pathways of obesity associated type 2 diabetes mellitus have not been completely investigated. Further studies are necessary to elucidate these essential genes and pathways to provide novel therapeutic targets for the treatment of obesity associated type 2 diabetes mellitus. Therefore, in this investigation, we downloaded the expression profiling by high throughput sequencing data GSE132831, provided by Osinski et al. [12], from Gene Expression Omnibus (GEO, http:// www.ncbi.nlm.nih.gov/geo/) [13] database to identify the differentially expressed genes (DEGs) between diabetic obese samples and non diabetic obese samples. With the identified DEGs, we performed Gene Ontology (GO) and pathway enrichment analyses to investigate the functions and pathways enriched by the DEGs. Additionally, we constructed a protein–protein interaction (PPI) network and The copyrightholderforthispreprint modules screened out some important gene nodes to perform clustering analysis. Furthermore, we constructed target gene - miRNA regulatory network and target gene - TF regulatory network based on these key genes to investigate the potential relationships between genes and obesity associated type 2 diabetes mellitus. Finally, hub genes were validated by using receiver operating characteristic (ROC) curve analysis and RT-PCR. Materials and Methods bioRxiv preprint RNA sequencing data The expression profiling by high throughput sequencing data GSE132831 was downloaded from the GEO database, which (which wasnotcertifiedbypeerreview)istheauthor/funder.Allrightsreserved.Noreuseallowedwithoutpermission. was based on the platform of GPL1857 Illumina NextSeq 500 (Homo sapiens). This dataset, including 104 diabetic obese doi: samples and 120 non diabetic obese samples, was deposited by Osinski et al. [12]. https://doi.org/10.1101/2020.12.25.424383 Identification of DEGs The limma R/Bioconductor software package was used to perform the identification of DEGs between diabetic obese samples and non diabetic obese samples in R software [14]. The cutoff criteria were |logFC| > 1.112 for up regulated genes, |logFC| < -0.64 for down regulated genes and a P-value <0.05. Gene Ontology (GO) and Reactome pathway enrichment analysis of DEGs The ToppGene (ToppFun) (https://toppgene.cchmc.org/enrichment.jsp) [15] is a gene functional enrichment program, ; this versionpostedDecember27,2020. providing a large series of functional annotation tools for researchers to decipher the biological implications behind huge amounts of genes. To understand the deeper biological meaning of DEGs, GO (http://geneontology.org/) [16] and REACTOME (https://reactome.org/) [17] pathway enrichment analysis of identified DEGs was performed using ToppGene. GO functional analysis consists of three categories: Biological process (BP), cellular component (CC) and molecular function (MF). P<0.05 was set as the threshold value. Protein-protein interaction (PPI) network and module analysis The IID interactome (http://iid.ophid.utoronto.ca/) [18] is an online database containing known and predicted PPI networks. In this investigation, a PPI network of identified DEGs in dataset was identified using the IID interactome database The copyrightholderforthispreprint (combined score >0.4) and subsequently visualized using Cytoscape (http://www.cytoscape.org/) software (version 3.8.2) [19]. The regulatory relationship between genes were analyzed through topological property of computing network including the node degree [20], betweenness centrality [21], stress centrality [22] and closeness centrality [23] by using the Network Analyzer app within Cytoscape. The PEWCC1 (http://apps.cytoscape.org/apps/PEWCC1) [24] program within Cytoscape was used to detect modules of the PPI network. The cut-off criteria were set as follows: Degree, ≥2; node score, bioRxiv preprint ≥0.2; k-score, 0.2; maximum depth, 100. The GO and pathway enrichment analysis of the identified modules was then performed using the ToppGene database. (which wasnotcertifiedbypeerreview)istheauthor/funder.Allrightsreserved.Noreuseallowedwithoutpermission. Target gene - miRNA regulatory network doi: https://doi.org/10.1101/2020.12.25.424383 In the current investigation, the miRNAs associated with DEGs were searched utilizing miRNet database (https://www.mirnet.ca/) [25] online tool, and target gene - miRNA regulatory network was visualized by Cytoscape software. Target gene - TF regulatory network In the current investigation, the TFs associated with DEGs were searched utilizing NetworkAnalyst database
Details
-
File Typepdf
-
Upload Time-
-
Content LanguagesEnglish
-
Upload UserAnonymous/Not logged-in
-
File Pages89 Page
-
File Size-