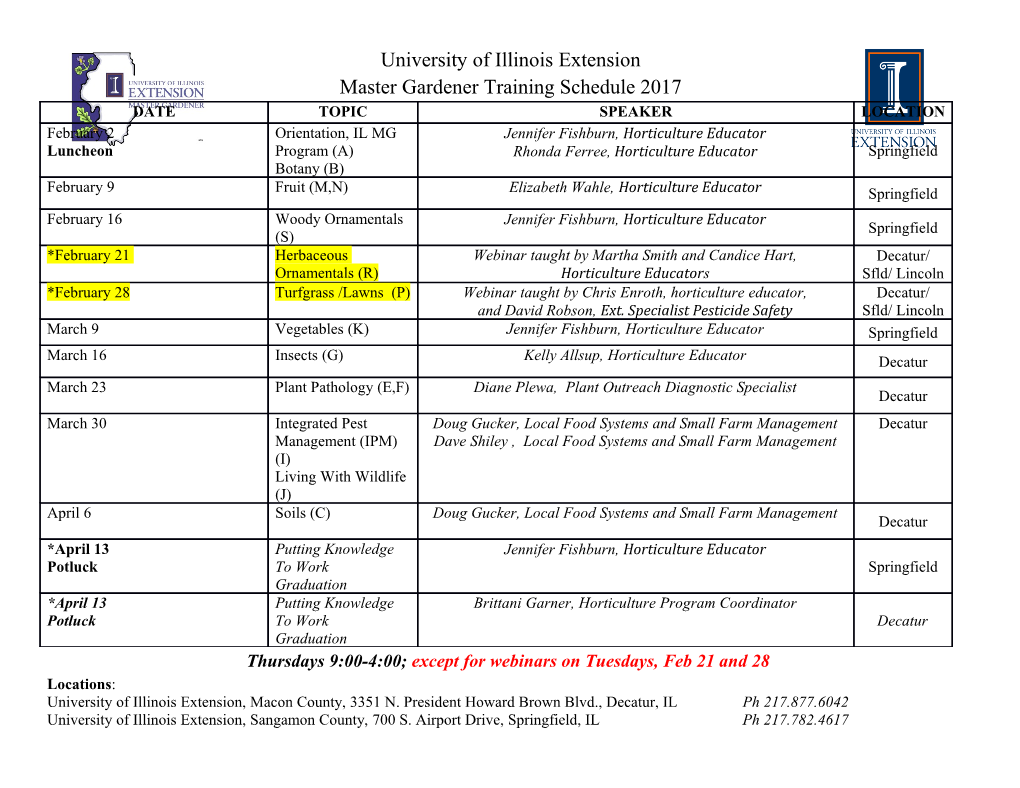
Chapter 4 SIMD or MIMD? Data-Level Parallelism ● Based on past history, the book predicts that for x86 in – We will get two additional cores per chip per year ● Linear growth Vector, SIMD, and GPU – We will get twice as wide SIMD instructions every 4 years ● Exponential growth – Potential speedup from SIMD to be twice that from MIMD! Architectures 1 4 Introduction: Focusing on SIMD Vector Architecture Basics ● SIMD architectures can exploit significant data- level parallelism for: ● The underlying idea: – matrix-oriented scientific computing – The processor does everything on a set of numbers at a time – media-oriented image and sound processors ● Read sets of data elements into “vector registers” ● SIMD is more energy efficient than MIMD ● Operate on those vector registers – Only needs to fetch one instruction per data operation ● Disperse the results back into memory in sets – Makes SIMD attractive for personal mobile devices ● Registers are controlled by compiler to... ● SIMD allows programmer to continue to think sequentially – ...hide memory latency – “Backwards compatibility” ● The price of latency paid only once for the first element of the vector ● Three major implementations ● No need for sophisticated prefetcher – Vector architectures – Always load consecutive elements from memory (unless gather-scatter) – SIMD extensions – ...leverage memory bandwidth – Graphics Processor Units (GPUs) 2 5 ILP vs. SIMD vs. MIMD Example Architecture: VMIPS ... ● Loosely based on Cray-1 ... ● Vector registers – Each register holds a 64-element, 64 bits/element vector – Register file has 16 read ports and 8 write ports ILP ... ● Vector functional units – Fully pipelined: new instruction every cycle ... – Data and control hazards are detected ... ● Vector load-store unit MIMD – Fully pipelined – One word per clock cycle after initial latency ● Scalar registers SIMD – 32 general-purpose registers of MIPS – 32 floating-point registers of MIPS 3 6 How Vector Processors Work: Y=a*X+Y Example Calculation of Execution Time LV V1,Rx ;load vector X L.D F0, a L.D F0, a MULVS.D V2,V1,F0 ;vector-scalar multiply DADDIU R4, Rx, #512 LV V1, Rx LV V3,Ry ;load vector Y Loop: MULVS.D V2, V1, F0 ADDVV.D V4,V2,V3 ;add two vectors L.D F2, 0(Rx) LV V3, Ry SV Ry,V4 ;store the sum MUL.D F2, F2, F0 ADDVV.DV4, V2, V3 L.D F4, 0(Ry) SV V4, Ry Convoys: ADD.D F4, F4, F2 1 LV MULVS.D S.D F4, 9(Ry) DADDIU Rx, Rx, #8 2 LV ADDVV.D DADDIU Ry, Ry, #8 3 SV DSUB R20, R4, Rx BNEZ Loop 3 chimes, 2 FP ops per result, cycles per FLOP = 1.5 600 instructions 6 instructions, no loop For 64 element vectors, requires 64 x 3 = 192 clock cycles 7 10 Vector Execution Time Vector Execution Challenges ● Execution time depends on three factors: ● Needed improvements – Length of operand vectors ● Start up time – > 1 element per clock cycle – Structural hazards ● Compare with CPI < 1 – Data dependencies – Latency of vector functional unit – Non-64 wide vectors ● In VMIPS: – Assume the same as Cray-1 – IF statements in vector code – Functional units consume one element per clock cycle ● Floating-point add → 6 – Memory system – Execution time is approximately the vector length cycles optimizations to support ● Floating-point multiply → 7 vector processors ● Convoy cycles – Multiple dimensional – Set of vector instructions that could potentially execute together ● Floating-point divide → 20 matrices ● cycles No structural hazards – Sparse matrices ● ● RAW hazards are avoided through chaining Vector load → 12 cycles – Programming a vector – In superscalar RISC it is called forwarding computer – Number of convoys gives an estimate of execution time 8 11 Chaining and Chimes Vector Optimizations: Multiple Lanes pipe 0 pipe 1 pipe 2 pipe 3 + + + A[9] B[9] + ● Chaining A[8] B[8] – Like forwarding in superscalar RISC Lane 0 Lane 1 Lane 2 Lane 3 A[7] B[7] – Allows a vector operation to start as soon as the individual V[1], V[5], V[2], V[6], V[3], V[7], V[4], V[8], elements of its vector source operand become available V[9], … V[10], … V[11], … V[12], … A[6] B[6] – Flexible chaining chaining with any other instruction in execution, not just the once sharing a register A[5] B[5] ● Chime A[4] B[4] x x x x – Unit of time to execute one convoy A[3] B[3] A[9] B[9] – m convoys executes in m chimes A[2] B[2] A[5] B[5] A[6] B[6] A[7] B[7] A[8] B[8] – For vector length of n, it is required m*n clock cycles A[1] B[1] A[1] B[1] A[2] B[2] A[3] B[3] A[4] B[4] – Ignores some processor specific overheads ● Better at estimating time for longer vector processors + + + + + – Chime is an underestimate Element C[1] C[1] C[2] group C[3] C[4] 9 12 Arbitrary Length Loops with Vector-Length Register Example: Vector Mask Register ● for (i=0; i < N; ++i) Y[i] = a * X[i] + Y[i] ● What if N < 64 (=Maximum Vector Length)? ● for (i=0 ; i<64; ++i) ● Use a Vector-Length Registers if (X[i] != 0) X[i] = X[i] – Y[i] ● LV V1, Rx – Load N into VLR LV V2, Ry – Vector instructions (loads, stores, arithmetic, …) use VLR for the L.D F0, #0 maximum number of iterations SNEVS.DV1, F0 – By default, VLR=64 ● Vector register utilization SUBVV.DV1, V1, V2 SV V1, Rx – If VLR<64 then the registers are not fully utilized drops with the dropping count of 1's in mask register ● Vector startup time is a greater portion of the instruction ● Use Amdahl's law to calculate the effect of diminishing vector length 13 16 Arbitrary Length Loops with Strip Mining Strided Access and Multidimensional Arrays ● ● for (i=0; i < N; ++i) Y[i] = a * X[i] + Y[i] Matrix-matrix multiply C= alpha * C + beta * A * B: for (i = 0; i < 64; ++i) ● What if N > 64? for (j = 0; j < 64; ++j) { C[i, j] = alpha * C[i, j] ● Use strip mining to split the loop into smaller chunks for (k = 0; k < 64; ++k) C[i,j] = C[i,j] + beta * A[i, k] * B[k, j] for (j = 0; j < (N/64)*64; j += 64) {//N - N%64 } for (i=0 ; i < 64; ++i) ● C[0,0] = A[0,0]*B[0,0]+A[0,1]*B[1,0]+A[0,2]*B[2,0]+... Y[j+i] = a * X[j+i] + Y[i] ● A is accessed by rows and B is accessed by columns } ● Two most common storage formats: ● Clean-up code for N not divisible by 64 1. C: row-major (store matrix by rows) if (N % 64) 2. F: column-major (store matrix by columns) ● There is a need for non-unit stride for accessing memory for (i = 0; i < N%64; ++i) // use VLR here – Best handled by memories with multiple banks Y[j+i] = a * X[j+i] + Y[i] – Stride: 64 elements * 8 bytes = 512 bytes 14 17 Handling Conditional Statements with Mask Regs. Strided Access and Memory Banks ● for (i=0 ; i < 64; ++i) if (X[i] != 0) X[i] = X[i] – Y[i] ● Special load instructions just for accessing strided data ● Values of X are not known until runtime – LVWS=load vector with stride and SVWS ● The loop cannot be analyzed at compile time – The stride (distance between elements) is loaded in GPR (general purpose register) just like vector address ● Conditional is the inherent part of the program logic ● Multiple banks help with fast data access ● Scientific codes are full of conditionals and hardware support is a must – Multiple loads and/or store can be active at the same time – Critical metric: bank busy time (minimum period of time between ● Vector mask register is used as to prohibit execution of vector successive accesses to the same bank) instructions on some elements of the vector for (i = 0; i < 64; ++i) ● For strided accesses, compute LCM (least common multiple) of VectorMask[i] = (X[i] == 0) stride and number of banks to see if bank conflict occurs for (i = 0; i < 64; ++i) X[i] = X[i] – Y[i] only if VectorMask[i] != 0 #banks < bank busy time cycles LCM(stride ,#banks) 15 18 Strided Access Patterns Vector Processors and Compilers 0 1 2 3 4 5 6 7 Stride = 1 1 2 3 4 1 CPU cycle ● Vector machine vendors supplied good compilers to take advantage of the hardware 0 1 2 3 4 5 6 7 Stride = 9 – High profit margins allowed investment in compilers 1 – Without well vectorized code, hardware cannot deliver high 2 Gflop/s rates 3 ● Vector compilers were... 1 CPU cycle 4 – Good at recognizing vectorization opportunities 0 1 2 3 4 5 6 7 Stride = 8 – Good at reporting vectorization process failures and successes 1 – Good at taking hints from the programmer in form of vendor- specific language extensions such as pragmas 2 3 1 bank cycle) max(1 CPU cycle, 4 19 22 Example: Memory banks for Cray T90 SIMD Extensions for Multimedia Processing ● Design parameters ● Modern SIMD extensions in x86 date back to early desktop – 32 processors processing of multimedia – Each processor: 4 loads and 2 stores per cycle ● Typical case of Amdahl's law: – Processor cycle time: 2.167 ns – Speedup the common case or the slowest component – Cycle time for SRAMs: 15 ns ● Image processing: operates on 8 bit colors, 3 components, 1 alpha ● How many memory banks required to keep up with the transparency processors? – Typical pixel length: 32 bits – Typical operations: additions – 32 processors * 6 references = 192 references per cycle ● Audio processing: 8 bit or 16 bit samples (Compact Disc) – SRAM busy time: 15/2.167 = 6.92 ~ 7 CPU cycles ● SIMD extensions versus vector processing – Required cycles: 192 * 7 = 1344 memory banks – Fixed vector length encoded in instruction opcode for SIMD – Original design had only 1024 memory banks.
Details
-
File Typepdf
-
Upload Time-
-
Content LanguagesEnglish
-
Upload UserAnonymous/Not logged-in
-
File Pages9 Page
-
File Size-