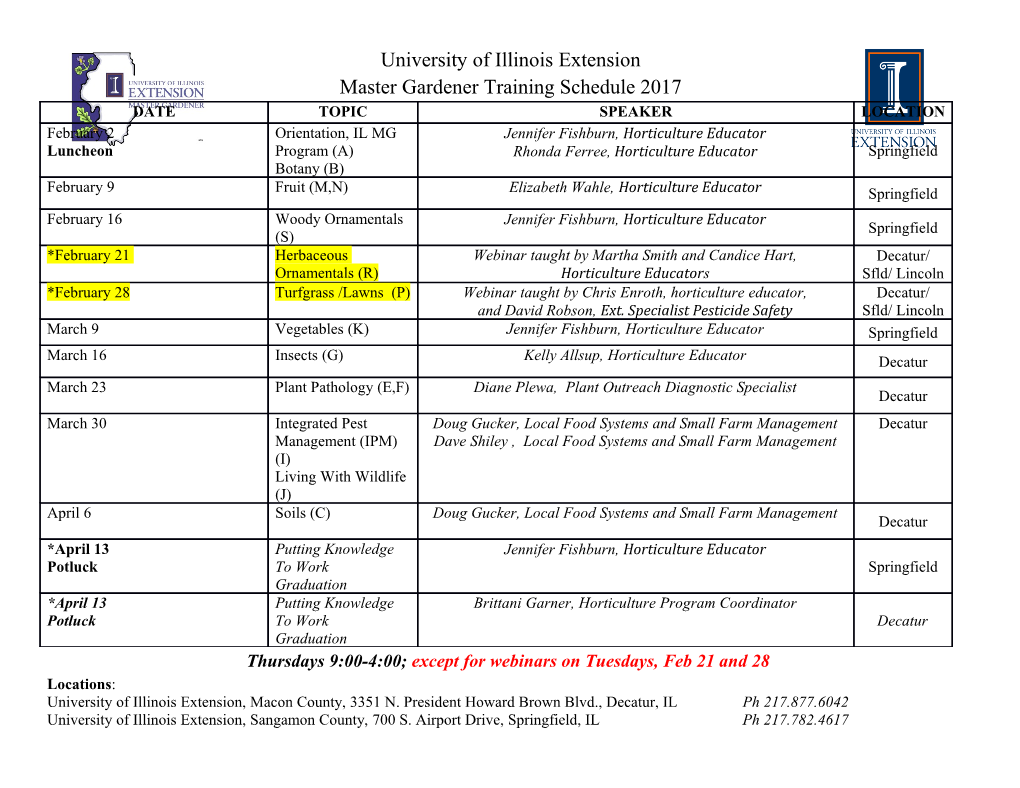
Chapter 7 Assessing and improving convergence of the Markov chain Questions: . Are we getting the right answer? . Can we get the answer quicker? Bayesian Biostatistics - Piracicaba 2014 376 7.1 Introduction • MCMC sampling is powerful, but comes with a cost: dependent sampling + checking convergence is not easy: ◦ Convergence theorems do not tell us when convergence will occur ◦ In this chapter: graphical + formal diagnostics to assess convergence • Acceleration techniques to speed up MCMC sampling procedure • Data augmentation as Bayesian generalization of EM algorithm Bayesian Biostatistics - Piracicaba 2014 377 7.2 Assessing convergence of a Markov chain Bayesian Biostatistics - Piracicaba 2014 378 7.2.1 Definition of convergence for a Markov chain Loose definition: With increasing number of iterations (k −! 1), the distribution of k θ , pk(θ), converges to the target distribution p(θ j y). In practice, convergence means: • Histogram of θk remains the same along the chain • Summary statistics of θk remain the same along the chain Bayesian Biostatistics - Piracicaba 2014 379 Approaches • Theoretical research ◦ Establish conditions that ensure convergence, but in general these theoretical results cannot be used in practice • Two types of practical procedures to check convergence: ◦ Checking stationarity: from which iteration (k0) is the chain sampling from the posterior distribution (assessing burn-in part of the Markov chain). ◦ Checking accuracy: verify that the posterior summary measures are computed with the desired accuracy • Most practical convergence diagnostics (graphical and formal) appeal to the stationarity property of a converged chain • Often done: base the summary measures on the converged part of the chain Bayesian Biostatistics - Piracicaba 2014 380 7.2.2 Checking convergence of the Markov chain Here we look at: • Convergence diagnostics that are implemented in ◦ WinBUGS ◦ CODA, BOA • Graphical and formal tests • Illustrations using the ◦ Osteoporosis study (chain of size 1,500) Bayesian Biostatistics - Piracicaba 2014 381 7.2.3 Graphical approaches to assess convergence Trace plot: • A simple exploration of the trace plot gives a good impression of the convergence • Univariate trace plots (each parameter separately) or multivariate trace plots (evaluating parameters jointly) are useful • Multivariate trace plot: monitor the (log) of likelihood or log of posterior density: ◦ WinBUGS: automatically created variable deviance ◦ Bayesian SASr procedures: default variable LogPost • Thick pen test: trace plot appears as a horizontal strip when stationarity • Trace plot evaluates the mixing rate of the chain Bayesian Biostatistics - Piracicaba 2014 382 7.2.4 Graphical approaches to assess convergence Trace plot: • A simple exploration of the trace plot gives a good impression of the convergence • Univariate trace plots (each parameter separately) or multivariate trace plots (evaluating parameters jointly) are useful • Multivariate trace plot: monitor the (log) of likelihood or log of posterior density: ◦ WinBUGS: automatically created variable deviance ◦ Bayesian SASr procedures: default variable LogPost • Thick pen test: trace plot appears as a horizontal strip when stationarity • Trace plot evaluates the mixing rate of the chain Bayesian Biostatistics - Piracicaba 2014 383 Univariate trace plots on osteoporosis regression analysis (a) 1 β 0.030 0.045 0 500 1000 1500 Iteration (b) 2 σ 0.06 0.080 0.10 500 1000 1500 Iteration Bayesian Biostatistics - Piracicaba 2014 384 Multivariate trace plot on osteoporosis regression analysis Log(posterior) 155 160 165 170 175 0 500 1000 1500 Iterations Bayesian Biostatistics - Piracicaba 2014 385 Autocorrelation plot: k (k+m) • Autocorrelation of lag m (ρm): corr(θ ,θ ) (for k = 1;:::) estimated by ◦ Pearson correlation ◦ Time series approach • Autocorrelation function (ACF): function m 7! ρbm (m = 0; 1;:::) • Autocorrelation plot: graphical representation of ACF and indicates ◦ Mixing rate ◦ Minimum number of iterations for the chain to `forget' its starting position • Autocorrelation plot is a NOT a convergence diagnostic. Except for the burn-in phase, the ACF plot will not change anymore when further sampling Bayesian Biostatistics - Piracicaba 2014 386 Autocorrelation plot on osteoporosis regression analysis 2 LOGPOS β1 σ 0.5 0.0 0.5 1.0 0.5 0.0 0.5 1.0 0.5 0.0 0.5 1.0 Autocorrelation Autocorrelation Autocorrelation % % % 1.0 1.0 1.0 % % % 0 10 20 30 40 50 0 10 20 30 40 50 0 10 20 30 40 50 Lag Lag Lag Bayesian Biostatistics - Piracicaba 2014 387 Running-mean plot: k • Running mean (ergodic mean) θ : mean of sampled values up to iteration k ◦ Checks stationarity for k > k0 of pk(θ) via the mean ◦ Initial variability of the running-mean plot is always relatively high ◦ Stabilizes with increasing k in case of stationarity Bayesian Biostatistics - Piracicaba 2014 388 Running-mean plot on osteoporosis regression analysis 2 LOGPOS β1 σ Autocorrelation Autocorrelation Autocorrelation −1.0 −0.5 0.0 0.5 1.0 −1.0 −0.5 0.0 0.5 1.0 −1.0 −0.5 0.0 0.5 1.0 0 10 20 30 40 50 0 10 20 30 40 50 0 10 20 30 40 50 Lag Lag Lag 2 1 β σ LOGPOS 155 160 165 170 175 0.070 0.080 0.090 0.100 0.035 0.040 0.045 0.050 0 500 1000 1500 0 500 1000 1500 0 500 1000 1500 Iterations Iterations Iterations Bayesian Biostatistics - Piracicaba 2014 389 Q-Q plot: • Stationarity: distribution of the chain is stable irrespective of part • Q-Q plot: 1st half of chain (X-axis) versus 2nd half of chain on (Y -axis) • Non-stationarity: Q-Q graph deviating from the bisecting line Bayesian Biostatistics - Piracicaba 2014 390 Q-Q plot on osteoporosis regression analysis 2 1 β σ 172 174 176 178 0.07 0.08 0.09 0.10 0.11 SECOND HALF OF 0.035 0.040 0.045 0.050 0.055 SECOND HALF OFSECOND SECOND HALF OF LOG(POS) 155 160 165 170 175 0.035 0.045 0.055 0.06 0.07 0.08 0.09 0.10 0.11 2 FIRST HALF OF LOG(POS) FIRST HALF OF β1 FIRST HALF OF σ Bayesian Biostatistics - Piracicaba 2014 391 Cross-correlation plot: • k k Cross-correlation of θ1 with θ2: correlation between θ1 with θ2 (k = 1; : : : ; n) • k k Cross-correlation plot: scatterplot of θ1 versus θ2 • Useful in case of convergence problems to indicate which model parameters are strongly related and thus whether model is overspecified Bayesian Biostatistics - Piracicaba 2014 392 Cross-correlation plot on osteoporosis regression analysis beta1 beta1 0.06 0.06 0.05 0.05 0.04 0.04 0.03 0.03 0.02 0.02 0.4 0.8 0.225 0.275 0.325 beta0 sigma Bayesian Biostatistics - Piracicaba 2014 393 7.2.5 Formal approaches to assess convergence Here: • Four formal convergence diagnostics: ◦ Geweke diagnostic: single chain ◦ Heidelberger-Welch diagnostic: single chain ◦ Raftery-Lewis diagnostic: single chain ◦ Brooks-Gelman-Rubin diagnostic: multiple chains • Illustrations based on the ◦ Osteoporosis study (chain of size 1,500) Bayesian Biostatistics - Piracicaba 2014 394 Geweke diagnostic: k ◦ Acts on a single chain (θ )k (k = 1; : : : ; n) ◦ Checks only stationarity ) looks for k0 ◦ Compares means of (10%) early , (50%) late part of chain using a t-like test • In addition, there is a dynamic version of the test: ◦ Cut off repeatedly x% of the chain, from the beginning ◦ Each time compute Geweke test • Test cannot be done in WinBUGS, but should be done in R accessing the sampled values of the chain produced by WinBUGS Bayesian Biostatistics - Piracicaba 2014 395 Geweke diagnostic on osteoporosis regression analysis • Based on chain of 1,500 iterations • R program geweke.diag (CODA) • With standard settings of 10% (1st part) & 50% (2nd part): numerical problems 2 • Early part = 20%, Geweke diagnostic for β1: Z = 0:057 and for σ : Z = 2:00 • Dynamic version of the Geweke diagnostic (with K = 20) next page, results: ◦ β1: majority of Z-values are outside [-1.96,1.96] ) non-stationarity ◦ log(posterior): non-stationary ◦ σ2: all values except first inside [-1.96,1.96] Bayesian Biostatistics - Piracicaba 2014 396 Geweke diagnostic on osteoporosis regression analysis var1 var1 var1 (a) (b) (c) Z−score Z−score Z−score −10 −5 0 5 10 −4 −2 0 2 4 −2 −1 0 1 2 0 200 400 600 0 200 400 600 0 200 400 600 First iteration in segment First iteration in segment First iteration in segment 2 (a) β1 (b) σ (c) log posterior Bayesian Biostatistics - Piracicaba 2014 397 BGR diagnostic: ◦ Version 1: Gelman and Rubin ANOVA diagnostic ◦ Version 2: Brooks-Gelman-Rubin interval diagnostic ◦ General term: Brooks-Gelman-Rubin (BGR) diagnostic ◦ Global and dynamic diagnostic ◦ Implementations in WinBUGS, CODA and BOA For both versions: ◦ 0 Take M widely dispersed starting points θm (m = 1;:::;M) ◦ k M parallel chains (θm)k (m = 1;:::;M) are run for 2n iterations ◦ The first n iterations are discarded and regarded as burn-in Bayesian Biostatistics - Piracicaba 2014 398 Trace plot of multiple chains on osteoporosis regression analysis beta1 chains 1:8 0.06 0.05 0.04 0.03 0.02 0.01 1 500 1000 1500 iteration Bayesian Biostatistics - Piracicaba 2014 399 Version 1: P n k . Within chains: chain mean θm = (1=n) k=1 θm (m = 1;:::;M) P . Across chains: overall mean θ = (1=M) m θm . ANOVA idea: P P ◦ W = 1 M s2 ; with s2 = 1 n (θk − θ )2 M Pm=1 m m n k=1 m m ◦ n M − 2 B = M−1 m=1(θm θ) . Two estimates for var(θk j y): ◦ b ≡ c k j n−1 1 Under stationarity: W and V var(θ y) = n W + nB good estimates ◦ Non-stationarity: W too small and Vb too large b Vb . Convergence diagnostic: R = W Bayesian Biostatistics - Piracicaba 2014 400 Version 1: .
Details
-
File Typepdf
-
Upload Time-
-
Content LanguagesEnglish
-
Upload UserAnonymous/Not logged-in
-
File Pages274 Page
-
File Size-