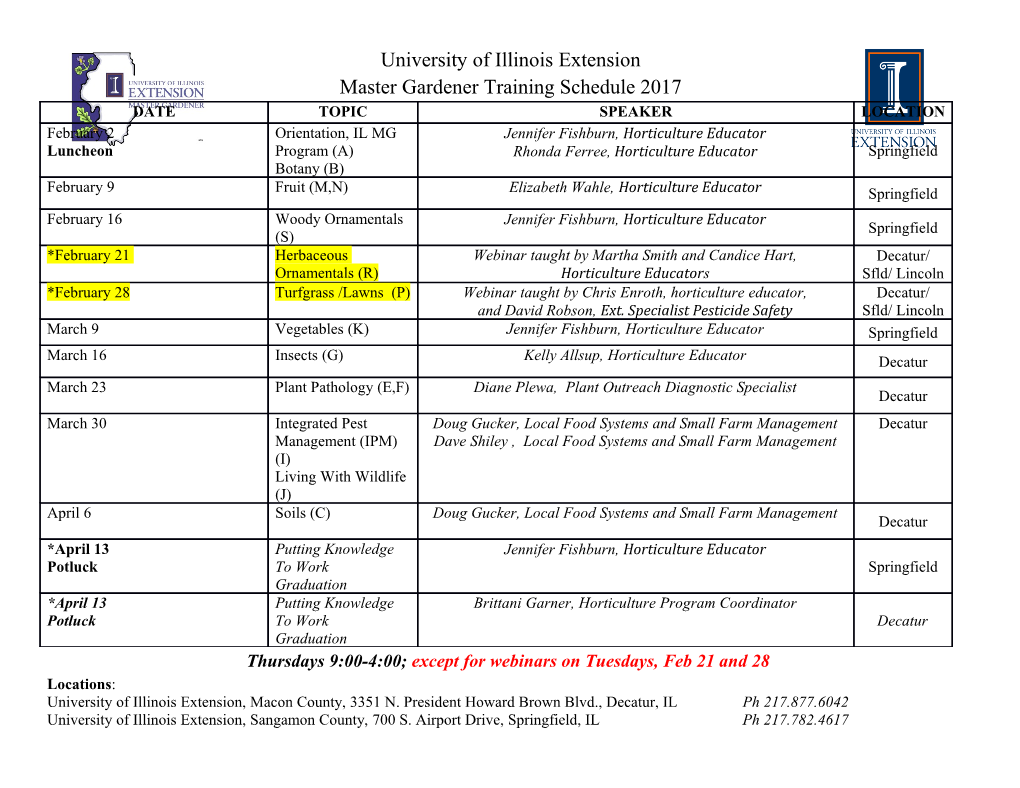
CHAPTER V DUAL SPACES DEFINITION Let (X, T ) be a (real) locally convex topological vector space. By the dual space X∗, or (X, T )∗, of X we mean the set of all continuous linear functionals on X. By the weak topology on X we mean the weakest topology W on X for which each f ∈ X∗ is continuous. In this context, the topology T is called the strong topology or original topology on X. EXERCISE 5.1. (a) Prove that X∗ is a vector space under pointwise operations. (b) Show that W ⊆ T . Show also that (X, W) is a locally convex topological vector space. (c) Show that if X is infinite dimensional then every weak neigh- borhood of 0 contains a nontrivial subspace M of X. HINT: If V = n −1 n ∩i=1fi (Ui), and if M = ∩i=1 ker(fi), then M ⊆ V. (d) Show that a linear functional f on X is strongly continuous if and only if it is weakly continuous; i.e., prove that (X, T )∗ = (X, W)∗. (e) Prove that X is finite dimensional if and only if X∗ is finite di- mensional, in which case X and X∗ have the same dimension. EXERCISE 5.2. (a) For 1 < p < ∞, let X be the normed linear 0 space Lp(R). For each g ∈ Lp (R) (1/p + 1/p0 = 1), define a linear functional φg on X by Z φg(f) = f(x)g(x) dx. 81 82 CHAPTER V p0 Prove that the map g → φg is a vector space isomorphism of L (R) onto X∗. (b) By analogy to part a, show that L∞(R) is isomorphic as a vector space to L1(R)∗. (c) Let c0 be the normed linear space of real sequences {a0, a1,... } for which lim an = 0 with respect to the norm defined by k{an}k = max |an|. ∗ 1 1 Show that c0 is algebraically isomorphic to l , where l is the linear space ∗ of all absolutely summable sequences {b0, b1,... }. HINT: If f ∈ c0, n n define bn to be f(e ), where e is the element of c0 that is 1 in the nth position and 0 elsewhere. (d) In each of parts a through c, show that the weak and strong topologies are different. Exhibit, in fact, nets (sequences) which converge weakly but not strongly. ∞ 1 (e) Let X = L (R). For each function g ∈ L (R), define φg on X by R ∗ φg(f) = fg. Show that φg is an element of X . Next, for each finite R Borel measure µ on R, define φµ on X by φµ(f) = f dµ. Show that ∗ 1 φµ is an element of X . Conclude that, in this sense, L (R) is a proper subset of (L∞)∗. (f) Let ∆ be a second countable locally compact Hausdorff space, and let X be the normed linear space C0(∆) equipped with the supremum norm. Identify X∗. (g) Let X1,...,Xn be locally convex topological vector spaces. If Ln ∗ Ln ∗ X = i=1 Xi, show that X is isomorphic to i=1 Xi . THEOREM 5.1. (Relation between the Weak and Strong Topolo- gies) Let (X, T ) be a locally convex topological vector space. (1) Let A be a convex subset of X. Then A is strongly closed if and only if it is weakly closed. (2) If A is a convex subset of X, then the weak closure of A equals the strong closure of A. (3) If {xα} is a net in X that converges weakly to an element x, then there exists a net {yβ}, for which each yβ is a (finite) con- vex combination of some of the xα’s, such that {yβ} converges strongly to x. If T is metrizable, then the net {yβ} can be chosen to be a sequence. PROOF. If A is a weakly closed subset, then it is strongly closed since W ⊆ T . Conversely, suppose that A is a strongly closed convex set and let x ∈ X be an element not in A. Then, by the Separation Theorem, there exists a continuous linear functional φ on X, and a real number s, such that φ(y) ≤ s for all y ∈ A and φ(x) > s. But then DUAL SPACES 83 the set φ−1(s, ∞) is a weakly open subset of X that contains x and is disjoint from A, proving that A is weakly closed, as desired. If A is a convex subset of X, and if B is the weak closure and C is the strong closure, then clearly A ⊆ C ⊆ B. On the other hand, C is convex and strongly closed, hence C is weakly closed. Therefore, B = C, and part 2 is proved. Now let {xα} be a weakly convergent net in X, and let A be the convex hull of the xα’s. If x = limW xα, then x belongs to the weak closure of A, whence to the strong closure of A. Let {yβ} be a net (sequence if T is metrizable) of elements of A that converges strongly to x. Then each yβ is a finite convex combination of certain of the xα’s, and part 3 is proved. DEFINITION. Let X be a locally convex topological vector space, and let X∗ be its dual space. For each x ∈ X, define a functionx ˆ on X∗ byx ˆ(f) = f(x). By the weak∗ topology on X∗, we mean the weakest topology W∗ on X∗ for which each functionx, ˆ for x ∈ X, is continuous. THEOREM 5.2. (Duality Theorem) Let (X, T ) be a locally convex topological vector space, and let X∗ be its dual space. Then: (1) Each function xˆ is a linear functional on X∗. (2) (X∗, W∗) is a locally convex topological vector space. (Each xˆ is continuous on (X∗, W∗).) (3) If φ is a continuous linear functional on (X∗, W∗), then there exists an x ∈ X such that φ =x ˆ; i.e., the map x → xˆ is a linear transformation of X onto (X∗, W∗)∗. (4) The map x → xˆ is a topological isomorphism between (X, W) and ((X∗, W∗)∗, W∗). PROOF. If x ∈ X, then xˆ(af + bg) = (af + bg)(x) = af(x) + bg(x) = axˆ(f) + bxˆ(g), proving part 1. By the definition of the topology W∗, we see that eachx ˆ is continuous. Also, the set of all functions {xˆ} separate the points of X∗, for if f, g ∈ X∗, with f 6= g, then f −g is not the 0 functional. Hence there exists an x ∈ X for which (f −g)(x), which isx ˆ(f)−xˆ(g), is not 0. Therefore, the weak topology on X∗, generated by thex ˆ’s, is a locally convex topology. See part c of Exercise 3.11. 84 CHAPTER V Now suppose φ is a continuous linear functional on (X∗, W∗), and let M be the kernel of φ. If M = X∗, then φ is the 0 functional, which is 0ˆ. Assume then that there exists an f ∈ X∗, for which φ(f) = 1, whence f∈ / M. Since φ is continuous, M is a closed subset in X∗, and there exists a weak* neighborhood V of f which is disjoint from M. Therefore, ∗ by the definition of the topology W , there exists a finite set x1, . , xn of elements of X and a finite set 1, . , n of positive real numbers such that ∗ V = {g ∈ X : |xˆi(g) − xˆi(f)| < i, 1 ≤ i ≤ n}. Define a map R : X∗ → Rn by R(g) = (x ˆ1(g),..., xˆn(g)). Clearly R is a continuous linear transformation of X∗ into Rn. Now R(f) ∈/ R(M), for otherwise there would exist a g ∈ M such thatx ˆi(g) = xˆi(f) for all i. But this would imply that g ∈ V ∩ M, contradicting the choice of the neighborhood V. Also, R(M) is a subspace of Rn, so contains 0, implying then that R(f) 6= 0. Suppose R(M) is of dimension n j < n. Let α1, . , αn be a basis for R , such that α1 = R(f) and n αi ∈ R(M) for 2 ≤ i ≤ j + 1. We define a linear functional p on R by setting p(α1) = 1 and p(αi) = 0 for 2 ≤ i ≤ n. Now, p ◦ R is a continuous linear functional on X∗. If g ∈ M, then (p ◦ R)(g) = p(R(g)) = 0, since R(g) ∈ R(M), which is in the span of α2, . , αn. Also, (p◦R)(f) = p(R(f)) = 1, since R(f) = α1. So, p◦R is a linear functional on X∗ which has the same kernel M as φ and agrees with φ on f. Therefore, φ − p ◦ R = 0 everywhere, and φ = p ◦ R. n Let e1, . , en denote the standard basis for R , and let A be the n × n matrix relating the bases e1, . , en and α1, . , αn. That is, ei = Pn Pn j=1 Aijαj. Then, if α = (a1, . , an) = i=1 aiei, we have n X p(α) = aip(ei) i=1 n n X X = ai Aijp(αj) i=1 j=1 n X = aiAi1. i=1 DUAL SPACES 85 Therefore, φ(g) = p ◦ R(g) = p(R(g)) = p((xc1(g),..., xcn(g))) n X = Ai1xbi(g) i=1 n X = ( Ai1xbi)(g) i=1 n X\ = Ai1xi(g) i=1 =x ˆ(g), Pn where x = i=1 Ai1xi, and this proves part 3. We leave the proof of part 4 to the exercises. EXERCISE 5.3. Prove part 4 of the preceding theorem.
Details
-
File Typepdf
-
Upload Time-
-
Content LanguagesEnglish
-
Upload UserAnonymous/Not logged-in
-
File Pages18 Page
-
File Size-