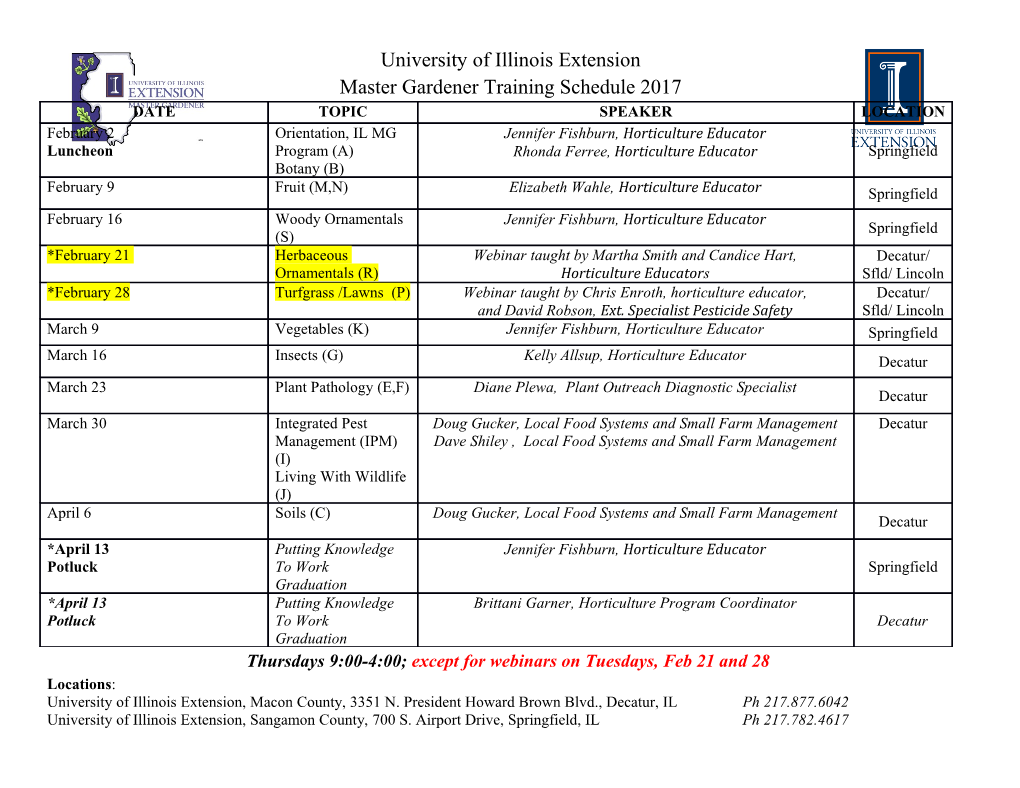
A governance framework for algorithmic accountability and transparency STUDY Panel for the Future of Science and Technology EPRS | European Parliamentary Research Service Scientific Foresight Unit (STOA) PE 624.262 – April 2019 EN A governance framework for algorithmic accountability and transparency Algorithmic systems are increasingly being used as part of decision-making processes in both the public and private sectors, with potentially significant consequences for individuals, organisations and societies as a whole. Algorithmic systems in this context refer to the combination of algorithms, data and the interface process that together determine the outcomes that affect end users. Many types of decisions can be made faster and more efficiently using algorithms. A significant factor in the adoption of algorithmic systems for decision-making is their capacity to process large amounts of varied data sets (i.e. big data), which can be paired with machine learning methods in order to infer statistical models directly from the data. The same properties of scale, complexity and autonomous model inference however are linked to increasing concerns that many of these systems are opaque to the people affected by their use and lack clear explanations for the decisions they make. This lack of transparency risks undermining meaningful scrutiny and accountability, which is a significant concern when these systems are applied as part of decision-making processes that can have a considerable impact on people's human rights (e.g. critical safety decisions in autonomous vehicles; allocation of health and social service resources, etc.). This study develops policy options for the governance of algorithmic transparency and accountability, based on an analysis of the social, technical and regulatory challenges posed by algorithmic systems. Based on a review and analysis of existing proposals for governance of algorithmic systems, a set of four policy options are proposed, each of which addresses a different aspect of algorithmic transparency and accountability: 1. awareness raising: education, watchdogs and whistleblowers; 2. accountability in public-sector use of algorithmic decision-making; 3. regulatory oversight and legal liability; and 4. global coordination for algorithmic governance. EPRS | European Parliamentary Research Service STOA | Panel for the Future of Science and Technology AUTHORS This study has been written by the following authors at the request of the Panel for the Future of Science and Technology (STOA) and managed by the Scientific Foresight Unit, within the Directorate-General for Parliamentary Research Services (EPRS) of the Secretariat of the European Parliament. Ansgar Koene, main author, University of Nottingham Chris Clifton, Purdue University Yohko Hatada, EMLS RI Helena Webb, Menisha Patel, Caio Machado, Jack LaViolette, University of Oxford Rashida Richardson, Dillon Reisman, AI Now Institute ADMINISTRATOR RESPONSIBLE Mihalis Kritikos, Scientific Foresight Unit (STOA) To contact the publisher, please e-mail [email protected] LINGUISTIC VERSION Original: EN Manuscript completed in March 2019. DISCLAIMER AND COPYRIGHT This document is prepared for, and addressed to, the Members and staff of the European Parliament as background material to assist them in their parliamentary work. The content of the document is the sole responsibility of its author(s) and any opinions expressed herein should not be taken to represent an official position of the Parliament. Reproduction and translation for non-commercial purposes are authorised, provided the source is acknowledged and the European Parliament is given prior notice and sent a copy. Brussels © European Union, 2019. PE 624.262 ISBN: 978-92-846-4656-2 doi: 10.2861/59990 QA-03-19-162-EN-N http://www.europarl.europa.eu/stoa (STOA website) http://www.eprs.ep.parl.union.eu (intranet) http://www.europarl.europa.eu/thinktank (internet) http://epthinktank.eu (blog) A governance framework for algorithmic accountability and transparency Executive summary This report presents an analysis of the social, technical and regulatory challenges associated with algorithmic transparency and accountability, including a review of existing proposals for the governance of algorithmic systems and the current state of development of related standards and consideration of the global and human rights dimensions of algorithmic governance. Motivation Algorithmic systems are increasingly being used as part of decision-making processes with potentially significant consequences for individuals, organisations and societies as a whole. When used appropriately, with due care and analysis of its impacts on people's lives, algorithmic systems, including artificial intelligence (AI) and machine learning, have great potential to improve human rights and democratic society. In order to achieve this however it is vitally necessary to establish clear governance frameworks for algorithmic transparency and accountability to make sure that the risk and benefits are equitably distributed in a way that does not unduly burden or benefit particular sectors of society. There is growing concern that unless appropriate governance frameworks are put in place, the opacity of algorithmic systems could lead to situations where individuals are negatively impacted because 'the computer says NO', with no recourse to meaningful explanation, a correction mechanism, or a way to ascertain faults that could bring about compensatory processes. As with the governance of any other aspect of society, the extent of algorithmic accountability required should be considered within the context of the good, harm, and risks these systems present. Background definitions and drivers for algorithmic transparency and accountability The study presents two 'conceptual landscapes' that explore the conceptual roles and uses of transparency and accountability in the context of algorithmic systems. The primary role of transparency is identified as a tool to enable accountability. If it is not known what an organisation is doing, it cannot be held accountable and cannot be regulated. Transparency may relate to the data, algorithms, goals, outcomes, compliance, influence and/or usage of automated decision making systems (i.e. algorithmic systems), and will often require different levels of detail for the general public, regulatory staff, third-party forensic analysts and researchers. The degree of transparency of an algorithmic systems often depends on a combination of governance processes and technical properties of the system. An important difference between transparency and accountability is that accountability is primarily a legal and ethical obligation on an individual or organisation to account for its activities, accept responsibility for them, and to disclose the results in a transparent manner. The challenges for algorithmic accountability arise from: the complex interactions between sub-systems and data sources, which might not all be under the control of the same entity; the impossibility of testing against all possible conditions when there are no formal proofs for the system's performance; difficulties in translating algorithmically derived concepts into human understandable concepts, resulting in incorrect interpretations; information asymmetries arising from algorithmic inferences; accumulation of many small (individually non-significant) algorithmic decisions; difficult to detect injections of adversarial data. When considering the governance of both transparency and accountability it is important to keep in mind the larger motivating drivers that define what is meant to be achieved. While recognising that fairness is an immensely complex concept with different, sometimes competing, definitions it is nevertheless seen as a fundamental component underpinning responsible systems and it is suggested that algorithmic processes should seek to minimise their potential to be unfair and maximise their potential to be fair. Transparency and accountability provide two important ways in which this can be achieved. Fairness is discussed through the lens of social justice, highlighting the I STOA | Panel for the Future of Science and Technology potential for algorithmic systems to systematically disadvantage, or even discriminate against, different social groups and demographics. A series of real life case studies is used to illustrate how this lack of fairness can arise, before exploring the consequences that lack of fairness can have plus the complexities inherent to trying to achieve fairness in any given societal context. The study describes ways in which lack of fairness in the outcomes of algorithmic systems might be caused by developmental decision-making and design features embedded at different points in the lifecycle of an algorithmic decision making model. A connection is made between the problem of fairness and the tools of transparency and accountability, while highlighting the value of responsible research and innovation (RRI) approaches to pursuing fairness in algorithmic systems. Technical challenges and solutions Viewing transparency as 'explaining the steps of the algorithm' is unlikely to lead to an informative outcome. On the one hand, it could result in a description that only captures the general process used to make a decision. At the other extreme would be to provide the complete set of steps taken (e.g. the complete detailed algorithm, or the machine learned model.) While this may enable the outcome to be reconstructed (provided
Details
-
File Typepdf
-
Upload Time-
-
Content LanguagesEnglish
-
Upload UserAnonymous/Not logged-in
-
File Pages124 Page
-
File Size-