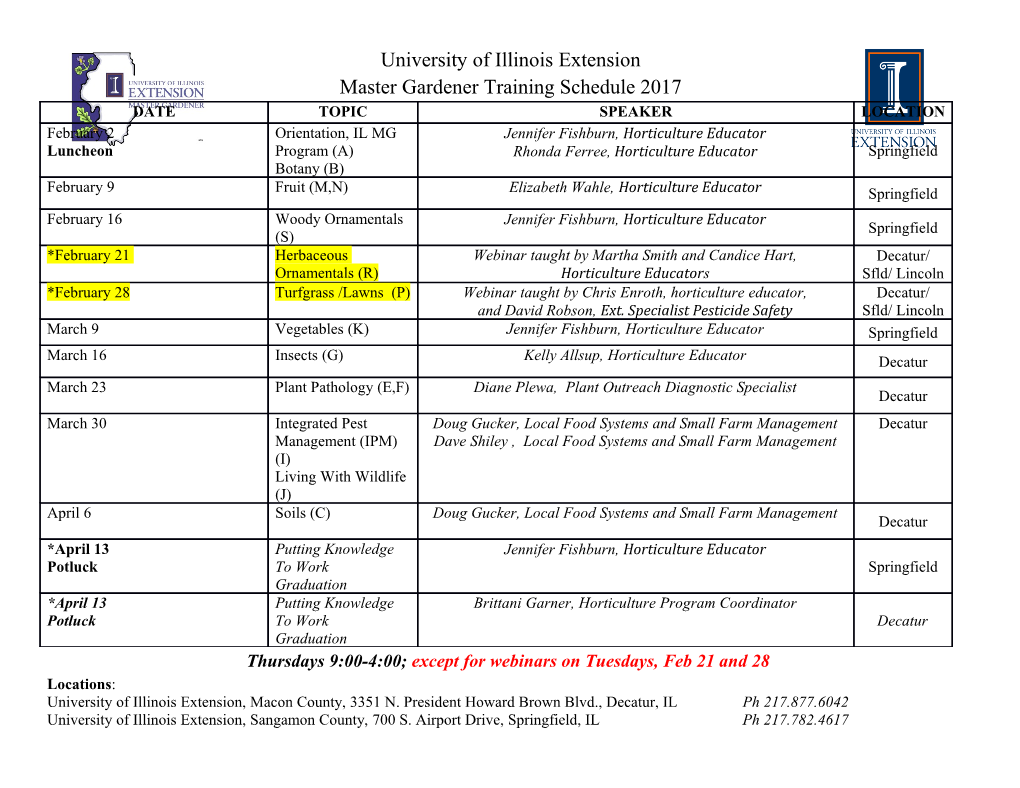
Journal of the American Statistical Association ISSN: 0162-1459 (Print) 1537-274X (Online) Journal homepage: http://www.tandfonline.com/loi/uasa20 Efficient Estimation for Semiparametric Structural Equation Models With Censored Data Kin Yau Wong, Donglin Zeng & D. Y. Lin To cite this article: Kin Yau Wong, Donglin Zeng & D. Y. Lin (2018) Efficient Estimation for Semiparametric Structural Equation Models With Censored Data, Journal of the American Statistical Association, 113:522, 893-905, DOI: 10.1080/01621459.2017.1299626 To link to this article: https://doi.org/10.1080/01621459.2017.1299626 View supplementary material Accepted author version posted online: 03 Mar 2017. Published online: 06 Jun 2018. Submit your article to this journal Article views: 481 View Crossmark data Full Terms & Conditions of access and use can be found at http://www.tandfonline.com/action/journalInformation?journalCode=uasa20 JOURNAL OF THE AMERICAN STATISTICAL ASSOCIATION , VOL. , NO. , –, Theory and Methods https://doi.org/./.. Efficient Estimation for Semiparametric Structural Equation Models With Censored Data Kin Yau Wong, Donglin Zeng, and D. Y. Lin Department of Biostatistics, University of North Carolina, Chapel Hill, NC ABSTRACT ARTICLE HISTORY Structural equation modeling is commonly used to capture complex structures of relationships among Received April multiple variables, both latent and observed. We propose a general class of structural equation models Revised January with a semiparametric component for potentially censored survival times. We consider nonparametric KEYWORDS maximum likelihood estimation and devise a combined expectation-maximization and Newton-Raphson Integrative analysis; Joint algorithm for its implementation. We establish conditions for model identifiability and prove the consis- modeling; Latent variables; tency, asymptotic normality, and semiparametric efficiency of the estimators. Finally, we demonstrate the Model identifiability; satisfactory performance of the proposed methods through simulation studies and provide an application Nonparametric maximum to a motivating cancer study that contains a variety of genomic variables. Supplementary materials for this likelihood estimation; article are available online. Survival analysis 1. Introduction Asparouhov, Masyn, and Muthén (2006) considered a more Structural equation modeling (SEM) is a very general and general formulation of the association among the latent and powerful approach to capture complex relationships among observed variables. SEM with the Cox proportional haz- multiple factors, both observed and latent (Bollen 1989). A typi- ardsmodelforthesurvivalcomponenthasbeenadoptedfor cal SEM framework consists of a structural model that connects more complex settings, such as multivariate survival times latent variables and a measurement model that relates latent (Stoolmiller and Snyder 2006) and competing risks (Stoolmiller variables to observed variables. SEM is extremely popular in the and Snyder 2014). A popular software program, Mplus (Muthén social sciences and psychology, where unmeasured quantities and Muthén 1998–2015), has implemented SEM with survival and psychological constructs, such as human intelligence and data under the proportional hazards model. The estimation creativity, can be related to and investigated through observed of the nonparametric baseline hazard function is based on data. The text of Bollen (1989) has been cited more than 20,000 piecewise-constant splines, and no theoretical justification is times. Recently, SEM has gained popularity in medical and available. In fact, the standard error estimator for the baseline public health research (Dahly, Adair, and Bollen 2009;Naliboff hazard function is incorrect. et al. 2012). In this article, we propose a general SEM framework that Our interest in SEM was motivated by its potential appli- includes a semiparametric component of the measurement cation to integrative analysis in genomic studies. Recent model for potentially censored survival times. Specifically, technologicaladvanceshavemadeitpossibletocollectdifferent we formulate the effects of latent and observed covariates types of genomic data, including DNA copy number, SNP geno- on survival times through a broad class of semiparametric type, DNA methylation level, and expression levels of mRNA, transformation models that includes the proportional hazards microRNA, and protein, on a large number of subjects. There model as a special case. The observed covariates may include is a growing interest in integrating these genomic platforms manifest variables that depend on latent variables. We study so as to understand their biological relationships and predict nonparametric maximum likelihood estimation (NPMLE), disease progression and death, which are considered potentially under which the cumulative hazard functions are estimated by censored survival times (The Cancer Genome Atlas (TCGA); step functions with jumps at observed survival times. https://tcga-data.nci.nih.gov/tcga/). The proposed SEM is reminiscent of joint modeling for sur- SEM with discrete survival times has been studied by vival and longitudinal data (Henderson, Diggle, and Dobson Rabe-Hesketh, Yang, and Pickles (2001), Rabe-Hesketh, Skro- 2000;TsiatisandDavidian2004). With the latter, the observed ndal, and Pickles (2004), Muthén and Masyn (2005), and longitudinal variables are considered error-prone measure- MoustakiandSteele(2005). For continuous survival time, ments of some underlying latent variables, but the measure- Larsen (2004, 2005) adopted the proportional hazards model ments themselves are not causal determinants of the survival (Cox 1972) with a single latent variable to capture the associ- time. By contrast, our SEM framework allows latent variables ation between the survival time and other observed variables; to have direct effects on survival times, as well as indirect effects CONTACT Danyu Lin [email protected] Department of Biostatistics, University of North Carolina, Chapel Hill, NC . Supplementary materials for this article are available online. Please go to www.tandfonline.com/r/JASA. © American Statistical Association 894 K. Y. WONG, D. ZENG, AND D. Y. LIN through other manifest variables. In addition, our framework W, Z, Y,andη as follows: accommodates much more complex relationships among latent η | Z ∼ Fη(·|Z; ν), (1) variables. Y | (Z, η) ∼ (·|Z, η; ψ), A major challenge in our theoretical development is model FY (2) identifiability. Even for an SEM with normally distributed (t | W, Z,Y, η) Tk variables, no single set of conditions exists that is both nec- W Tϑ +ZTβ +Y Tα +ηTφ = G (t) e k k k k , k = 1,...,K, (3) essary and sufficient for model identifiability. Methods that k k deal with special cases of the normal SEM were proposed by where Fη(·|Z, ν) denotes a q-variate normal distribution func- Bollen (1989), Reilly and O’Brien (1996), Vicard (2000), and tion indexed by a parameter vector ν, FY (·|Z, η; ψ) denotes an Bollen and Davis (2009), among others. Most of the methods r-variate parametric distribution function indexed by a param- ψ are based on the fact that identifiability can be established eter vector , Tk isthecumulativehazardfunctionofTk by solving the equations relating the first two model-implied given (W, Z,Y, η), Gk is a known increasing function, k is moments to the sample moments. This approach is not directly an unspecified positive increasing function with k(0) = 0, and (ϑ , β , α , φ ) applicable to models with nonparametric components, as k k k k are unknown regression parameters. infinite-dimensional parameters cannot be identified through Model (1) is the structural model of the latent variables. a finite number of equations. Because the proportional hazards Model (2) is the measurement model of Y. We assume that structure results in a likelihood function that takes the form of Y and η are independent of W given Z.Models(1)and(2) a Laplace transform, however, we are able to develop sufficient represent the existing SEM framework with Y not restricted to conditions under which the identifiability of a semiparamet- be normally distributed. Equation (3) includes the proportional ric SEM can be established by inspecting simpler parametric hazards and proportional odds models as special cases with models. the choices of Gk(x) = x and Gk(x) = log(1 + x),respectively. Another theoretical challenge is the invertibility of the infor- The proportional hazards model has been considered in the mation operator. For the information operator to be invertible, literature. we require that the score statistic along any nontrivial submodel The survival time Tk is subject to right censoring by Ck.It is nonzero. As in the case of model identifiability, general is assumed that (C ,...,C ) are independent of (T ,...,T ) 1 K ˜ 1 K conditions for the invertibility of the information operator and η conditional on Y, Z,andW.DefineTk = min(Tk,Ck ) for semiparametric models do not exist. In the existing work and k = I(Tk ≤ Ck),whereI(·) is the indicator function. involving latent variables for survival times (Kosorok, Lee, and For a sample of size n,theobserveddataconsistofO ≡ ˜ ˜ i Fine 2004;ZengandLin2010), verifying the invertibility of the (T1i,...TKi,1i,...,Ki,Y i, Zi,W i) (i = 1,...,n). information operator involves inspecting the local behavior of Let θ denote the collection of all Euclidean parameters, and the score statistic around the zero survival time. This approach write A = (1,...,K ). The likelihood function for θ and
Details
-
File Typepdf
-
Upload Time-
-
Content LanguagesEnglish
-
Upload UserAnonymous/Not logged-in
-
File Pages14 Page
-
File Size-