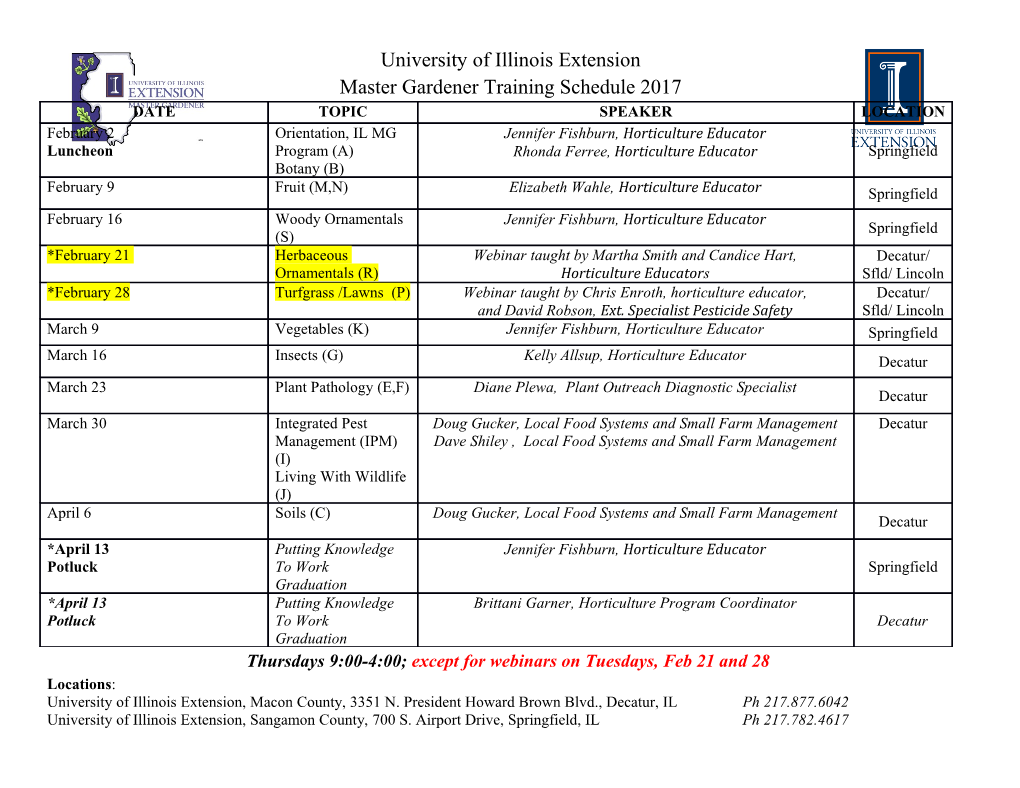
Ryan Tibshirani Carnegie Mellon University http://www.stat.cmu.edu/∼ryantibs/ Depts. of Statistics and Machine Learning [email protected] 229B Baker Hall 412.268.1884 Pittsburgh, PA 15213 Academic Positions Associate Professor (Tenured), Depts. of Statistics and Machine Learning, July 2016 { present Carnegie Mellon University Joint Appointment, Dept. of Machine Learning, Carnegie Mellon University Oct 2013 { present Assistant Professor, Dept. of Statistics, Carnegie Mellon University Aug 2011 { June 2016 Education Ph.D. in Statistics, Stanford University. Sept 2007 { Aug 2011 Thesis: \The Solution Path of the Generalized Lasso". Advisor: Jonathan Taylor. B.S. in Mathematics, Stanford University. Sept 2003 { June 2007 Minor in Computer Science. Grants, Awards, Honors \Theoretical Foundations of Deep Learning", Department of Defense (DoD) May 2020 { Apr 2025 Multidisciplinary University Research Initiative (MURI) grant. (co-PI, Rich Baraniuk is PI) \Delphi Influenza Forecasting Center of Excellence", Centers for Disease Sept 2019 | Aug 2024 Control and Prevention (CDC) grant no. U01IP001121, total award amount $3,000,000 (co-PI, Roni Rosenfeld is PI) \Improved Nowcasting via Adaptive Boosting of Highly Variable Biosurveil- Nov 2017 { May 2020 lance Data Sources", Defense Threat Reduction Agency (DTRA) grant no. HDTRA1-18-C-0008, total award amount $1,016,057 (co-PI, Roni Rosenfeld is PI) Teaching Innovation Award from Carnegie Mellon University Apr 2017 \Locally Adaptive Nonparametric Estimation for the Modern Age | New July 2016 { June 2021 Insights, Extensions, and Inference Tools", National Science Foundation (NSF) Division of Mathematical Sciences (DMS) CAREER grant no. 1554123, total award amount $400,000 (PI) \Graph Trend Filtering for Recommender Systems", Adobe Digital Marketing Sept 2014 { Sept 2015 Research Awards, total award amount $50,000, (co-PI, Alex Smola is PI) 1 \Advancing Theory and Computation in Statistical Learning Problems", July 2013 { June 2016 National Science Foundation (NSF) Division of Mathematical Sciences (DMS) grant no. 1309174, total award amount $150,000 (PI) Yahoo! Key Scientific Challenges Winner in Statistics and Machine Learning Sept 2010 Statistics Dept. Teaching Assistant Award June 2010 National Science Foundation (NSF) VIGRE Fellowship June 2007 { Aug 2010 Phi Beta Kappa June 2007 Departmental Honors in Mathematics June 2007 Distinction from Stanford University June 2007 Professional Service Committee Service Steering Commitee for Association for Computing Machinery-Institute of 2020 { present Mathematical Statistics (ACM-IMS) Foundations of Data Science Conference Institute for Pure and Applied Mathematics (IPAM) Scientific Advisory 2019 { present Board Steering Commitee for Association for Computing Machinery-Institute of 2019 Mathematical Statistics (ACM-IMS) Interdisciplinary Summit on Founda- tions of Data Science Associate Chair for Joint Statistical Meetings (JSM) 2018 Editorial Service Associate Editor for Journal of the American Statistical Association (JASA) 2019 { present Editor for Springer Series in the Data Sciences 2018 { present Associate Editor for Journal of Machine Learning Research (JMLR) 2018 { present Associate Editor for Annals of Statistics 2016 { present Area Chair for the ML Conferences: Artificial Intelligence and Statistics 2014 { present (AISTATS), International Conference on Machine Learning (ICML), and Neural Information Processing Systems (NIPS) (usually just one conference per year) Associate Editor for Biometrika 2013 { 2016 Associate Editor for Statistical Analysis and Data Mining 2013 { 2016 2 Referee Service Referee for Annals of Statistics, Bernoulli, Journal of the Royal Statistical So- 2010 { present ciety: Series B (JRSS-B), Journal of the American Statistical Society (JASA), Journal of Computational and Graphics Statistics (JCGS), Statistica Sinica, Biometrics, Statistics in Medicine, Journal of Machine Learning Research (JMLR), IEEE Transactions on Information Theory, IEEE Transactions on Pattern Analysis and Machine Intelligence Operations Research, Proceedings of the National Academy of Sciences (PNAS) Referee for Neural Information Processing Systems (NIPS) and International 2013 { 2017 Conference on Machine Learning (ICML) Panelist for National Science Foundation (NSF) Division of Mathematical 2015 Sciences (DMS) Grant Program Referee for National Security Agency and American Mathematical Society 2013 (NSA-AMS) Grant Program Published Articles David Farrow, Maria Jahja, Roni Rosenfeld, and Ryan Tibshirani. \Kalman Filter, Sensor Fusion, and Constrained Regression: Equivalences and Insights". Neural Information Processing Systems, 2019. Rina Foygel Barber, Emmanuel Candes, Aaditya Ramdas, and Ryan Tibshirani. \Conformal Prediction Under Covariate Shift". Neural Information Processing Systems, 2019. Trevor Hastie, Robert Tibshirani, and Ryan Tibshirani. \Best Subset, Forward Stepwise, or Lasso? Analysis and Recommendations Based on Extensive Comparisons". To appear, Statistical Science, 2020. Saharon Rosset and Ryan Tibshirani. \From Fixed-X to Random-X Regression: Bias-Variance Decomposi- tions, Covariance Penalties, and Prediction Error Estimation". To appear, Journal of the American Statistical Association, 2020. Veeranjaneyulu Sadhanala and Ryan Tibshirani. \Additive Models with Trend Filtering". Vol. 47, No. 6, 3032{3068, 2019. Veeranjaneyulu Sadhanala, Yu-Xiang Wang, Aaditya Ramdas, and Ryan Tibshirani. \A Higher-Order Kolmogorov Smirnov Test". International Conference on Artificial Intelligence and Statistics, 2019. Alnur Ali, Zico Kolter, and Ryan Tibshirani. \A Continuous-Time View of Early Stopping for Least Squares Regression". International Conference on Artificial Intelligence and Statistics, 2019. Alnur Ali and Ryan Tibshirani. \The Generalized Lasso Problem and Uniqueness." Electronic Journal of Statistics, Vol. 13, No. 2, 2307{2347, 2019. Logan Brooks, David Farrow, Sangwon Hyun, Ryan Tibshirani, and Roni Rosenfeld. \Nonmechanistic Forecasts of Seasonal Influenza with Iterative One-Week-Ahead Distributions". PLOS Computational Biology, Vol. 14, No. 6, 1{29, 2018. Ryan Tibshirani and Saharon Rosset. \Excess Optimism: How Biased is the Apparent Error of an Estimator Tuned by SURE?". Journal of the American Statistical Association, Vol. 114, No. 526, 697{712, 2019. Oscar Hernan Madrid Padilla, James Sharpnack, James Scott, and Ryan Tibshirani. \The DFS Fused Lasso: Linear-Time Denoising over General Graphs". Journal of Machine Learning Research, Vol. 18, No. 176, 1{36, 2018. 3 Jing Lei, Max G'Sell, Alessandro Rinaldo, Ryan Tibshirani, and Larry Wasserman. \Distribution-Free Predictive Inference for Regression". Journal of the American Statistical Association, Vol. 113, No. 523, 1094{1111, 2018. Sangwon Hyun, Max G'Sell, and Ryan Tibshirani. \Exact Post-Selection Inference for the Generalized Lasso Path." Electronic Journal of Statistics, Vol. 12, 1053-1097, 2018. Ryan Tibshirani, Alessandro Rinaldo, Robert Tibshirani, Larry Wasserman. \Uniform Asymptotic Inference and the Bootstrap After Model Selection". Annals of Statistics, Vol. 46, No. 3, 1255-1287, 2018. Veeranjaneyulu Sadhanala, Yu-Xiang Wang, James Sharpnack, and Ryan Tibshirani. \Higher-Order Total Variation Classes on Grids: Minimax Theory and Trend Filtering Methods". Neural Information Processing Systems, 2017. Kevin Lin, James Sharpnack, Alessandro Rinaldo, and Ryan Tibshirani. \A Sharp Error Analysis for the Fused Lasso, with Application to Approximate Changepoint Screening". Neural Information Processing Systems, 2017. Ryan Tibshirani. \Dykstra's Algorithm, ADMM, and Coordinate Descent: Connections, Insights, and Extensions". Neural Information Processing Systems, 2017. David Farrow, Logan Brooks, Sangwon Hyun, Ryan Tibshirani, Donald Burke, and Roni Rosenfeld. \A Human Judgment Approach to Epidemiological Forecasting". PLOS Computational Biology, Vol. 13, No. 3, 1-19, 2017. Alnur Ali, Zico Kolter, and Ryan Tibshirani. \The Multiple Quantile Graphical Model". Advances in Neural Information Processing Systems, 2016. Veeranjaneyulu Sadhanala, Yu-Xiang Wang, and Ryan Tibshirani. \Total Variation Classes Beyond 1d: Minimax Rates, and the Limitations of Linear Smoothers". Advances in Neural Information Processing Systems, 2016. Veeranjaneyulu Sadhanala, Yu-Xiang Wang, Alex Smola, and Ryan Tibshirani. \Graph Sparsification Approaches for Laplacian Smoothing". International Conference on Artificial Intelligence and Statistics, 2016. Yu-Xiang Wang, James Sharpnack, Alex Smola, and Ryan Tibshirani. \Trend Filtering on Graphs". Journal of Machine Learning Research, Vol. 17, 1-41, 2016. Ryan Tibshirani, Jonathan Taylor, Richard, Lockhart, and Robert Tibshirani. \Exact Post-selection Inference for Sequential Regression Procedures". Journal of the American Statistical Association, Vol. 111, No. 514, 600-620, 2016. Jonathan Taylor, Joshua Loftus, and Ryan Tibshirani. \Inference in Adaptive Regression via the Kac-Rice Formula". Annals of Statistics, Vol. 44, No. 2, 743-770, 2016. Yen-Chi Chen, Christopher Genovese, Ryan Tibshirani, and Larry Wasserman. \Nonparametric Modal Regression". Annals of Statistics, Annals of Statistics, Vol. 44, No. 2, 489-514, 2016. Aaditya Ramdas and Ryan Tibshirani. \Fast and Flexible ADMM Algorithms for Trend Filtering". Journal of Computational and Graphical Statistics, Vol. 25, No. 3, 839-858, 2016. Taylor Arnold and Ryan Tibshirani. “Efficient Implementations of the Generalized Lasso Dual Path Algorithm". Journal of Computational and Graphical Statistics,
Details
-
File Typepdf
-
Upload Time-
-
Content LanguagesEnglish
-
Upload UserAnonymous/Not logged-in
-
File Pages11 Page
-
File Size-