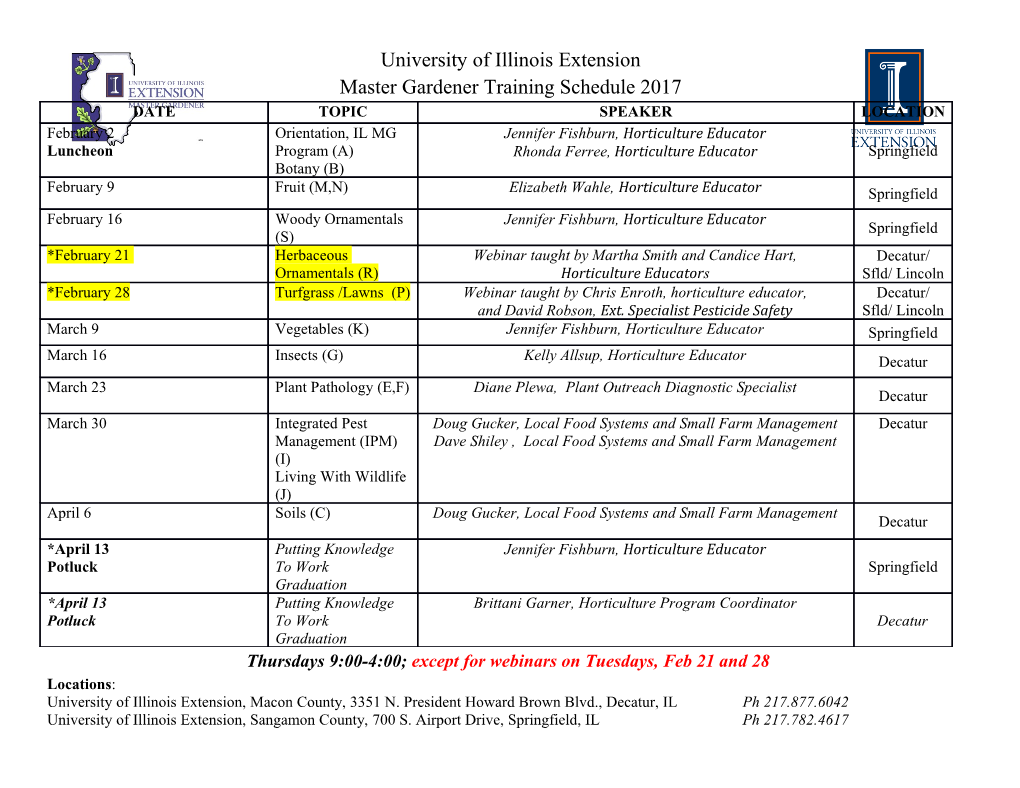
KTH ROYAL INSTITUTE OF TECHNOLOGY Eigenvalue Perturbation Results, Motivation Lecture 7 We know from a previous lecture thatρ(A) A for any ≤ ||| ||| � Chapter 6 + Appendix D: Location and perturbation of matrix norm. That is, we know that all eigenvalues are in a eigenvalues circular disk with radius upper bounded by any matrix norm. � Some other results on perturbed eigenvalue problems More precise results? � Chapter 8: Nonnegative matrices What can be said about the eigenvalues and eigenvectors of Magnus Jansson/Mats Bengtsson, May 11, 2016 A+�B when� is small? 2 / 29 Geršgorin circles Geršgorin circles, cont. Geršgorin’s Thm: LetA=D+B, whereD= diag(d 1,...,d n), IfG(A) contains a region ofk circles that are disjoint from the andB=[b ] M has zeros on the diagonal. Define rest, then there arek eigenvalues in that region. ij ∈ n n 0.6 r �(B)= b i | ij | j=1 0.4 �j=i � 0.2 ( , )= : �( ) ] C D B z C z d r B λ i { ∈ | − i |≤ i } Then, all eigenvalues ofA are located in imag[ 0 n λ (A) G(A) = C (D,B) k -0.2 k ∈ i ∀ i=1 � -0.4 TheC i (D,B) are called Geršgorin circles. 0 0.2 0.4 0.6 0.8 1 1.2 1.4 real[ λ] 3 / 29 4 / 29 Geršgorin, Improvements Invertibility and stability SinceA T has the same eigenvalues asA, we can do the same IfA M is strictly diagonally dominant such that ∈ n but summing over columns instead of rows. We conclude that n T aii > aij i λi (A) G(A) G(A ) i | | | |∀ ∈ ∩ ∀ j=1 �j=i � 1 SinceS − AS has the same eigenvalues asA, the above can be then “improved” by 1. A is invertible. 1 1 T 2. If all main diagonal elements are real and positive then all λi (A) G(S − AS) G (S− AS) i ∈ ∩ ∀ eigenvalues are in the right half plane. for any choice ofS. For it to be useful,� S should� be “simple”, 3. IfA is Hermitian with all diagonal elements positive, then e.g., diagonal (see e.g. Corollaries 6.1.6 and 6.1.8). all eigenvalues are real and positive. 5 / 29 6 / 29 Reducible matrices Irreducibly diagonally dominant A matrixA M is called reducible if IfA M is called irreducibly diagonally dominant if ∈ n ∈ n � n= 1 andA= 0 or i) A is irreducible (=A has the SC property). � n 2 and there is a permutation matrixP M such ii) A is diagonally dominant, ≥ ∈ n that n B C r aii aij i PT AP= } | |≥ | |∀ 0 D n r j=1 �j=i � �} − � r n r − iii) For at least one row,i, for some integer 1 r n 1. n ≤ ≤ −���� ���� A matrixA M that is not reducible is called irreducible. aii > aij ∈ n | | | | A matrix is irreducible iff it describes a strongly connected j=1 �j=i directed graph, “A has the SC property”. � 7 / 29 8 / 29 Invertibility and stability, stronger result Perturbation theorems IfA M n is irreducibly diagonally dominant, then Thm: LetA,E M n and letA be diagonalizable, ∈ ∈1 ˆ 1. A is invertible. A=SΛS − . Further, let λ be an eigenvalue ofA+E. 2. If all main diagonal elements are real and positive then all Then there is some eigenvalueλ i ofA such that 1 eigenvalues are in the right half plane. λˆ λ S S − E =κ(S) E | − i |≤ ||| ||| ||| ||| ||| ||| ||| ||| 3. IfA is Hermitian with all diagonal elements positive, then for some particular matrix norms (e.g., all eigenvalues are real and positive. 1, 2, ). ||| · ||| ||| · ||| ||| · |||∞ Cor: IfA is a normal matrix,S is unitary = S = S 1 = 1. This gives ⇒ ||| |||2 ||| − |||2 λˆ λ E | − i |≤ ||| ||| 2 indicating that normal matrices are perfectly conditioned for eigenvalue computations. 9 / 29 10 / 29 Perturbation cont’d Perturbation of a simple eigenvalue If bothA andE are Hermitian, we can use Weyl’s theorem Letλ be a simple eigenvalue ofA M and lety andx be ∈ n (here we assume the eigenvalues are indexed in non-decreasing the corresponding left and right eigenvectors. Theny x= 0. ∗ � order): Thm: LetA(t) M n be differentiable att= 0 and assumeλ λ (E) λ (A+E) λ (A) λ (E) k ∈ 1 ≤ k − k ≤ n ∀ is a simple eigenvalue ofA(0) with left and right We also have for this case eigenvectorsy andx. Ifλ(t) is an eigenvalue ofA(t) for n 1/2 smallt such thatλ(0) =λ then 2 λk (A+E) λ k (A) E 2 y ∗A�(0)x | − | ≤ || || λ�( ) = �k=1 � 0 � y ∗x where 2 is the Frobenius norm. y ∗Ex Example: A(t)=A+ tE givesλ �(0) = . || · || y ∗x 11 / 29 12 / 29 Perturbation of eigenvalues cont’d Literature with perturbation results Errors in eigenvalues may also be related to the residual J. R. Magnus and H. Neudecker. r=A xˆ λˆxˆ. Assume for example thatA is diagonalizable Matrix Differential Calculus with Applications in Statistics and − 1 A=SΛS − and letˆx and λˆ be a given complex vector and Econometrics. scalar, respectively. Then there is some eigenvalue ofA such John Wiley & Sons Ltd., 1988, rev. 1999. that H. Krim and P. Forster. Projections on unstructured subspaces. ˆ r IEEE Trans. SP, 44(10):2634–2637, Oct. 1996. λ λ i κ(S) || || | − |≤ xˆ J. Moro, J. V. Burke, and M. L. Overton. || || (for details and conditions see book). On the Lidskii-Vishik- Lyusternik perturbation theory for eigenvalues of matrices with arbitrary Jordan structure. We conclude that a small residual implies a good SIAM Journ. Matrix Anal. and Appl., 18(4):793–817, 1997. approximation of the eigenvalue. F. Rellich. Perturbation Theory of Eigenvalue Problems. Gordon & Breach, 1969. J. Wilkinson. The Algebraic Eigenvalue Problem. Clarendon Press, 1965. 13 / 29 14 / 29 Perturbation of eigenvectors with simple eigenva- Perturbation of eigenvectors with simple eigenva- lues lues: The real symmetric case Assume thatA M (R) is real symmetric matrix with ∈ n Thm: LetA(t) M n be differentiable att= 0 and assume normalized eigenvectorsx i and eigenvaluesλ i . Further assume ∈ ˆ λ0 is a simple eigenvalue ofA(0) with left and right thatλ 1 is a simple distinct eigenvalue. Let A=A+�B where eigenvectorsy 0 andx 0. Ifλ(t) is an eigenvalue ofA(t), it � is a small scalar,B is real symmetric and let xˆ1 be an has a right eigenvectorx(t) for smallt normalized such that eigenvector of Aˆ that approachesx as� 0. Then afirst 1 → order approximation (in�) is x0∗x(t)= 1, with derivative n T x0y0∗ xk Bx1 x�(0) = (λ0I A(0)) † I A�(0)x0 ˆ1x x 1 =� xk − − y0∗x0 − λ1 λ k � � �k=2 − B† denotes the Moore-Penrose pseudo inverse of a Warning: Non-unique derivative in the complex valued matrixB. case! See, e.g., J. R. Magnus and H. Neudecker. Matrix Differential Warning, Warning Warning: No extension to multiple Calculus with Applications in Statistics and Econometrics. eigenvalues! 15 / 29 16 / 29 Chapter 8: Element-wise nonnegative matrices Nonnegative matrices: Some properties Def: A matrixA=[a ] M is nonnegative ifa 0 for all LetA,B M andx C n. Then ij ∈ n,r ij ≥ ∈ n ∈ i,j, and we write this asA 0. (Note that this should not � Ax A x ≥ | |≤| || | be confused with the matrix being nonnegative definite!) � AB A B | |≤| || | Ifa > 0 for alli,j, we say thatA is positive and write this � IfA 0, thenA m 0; ifA> 0, thenA m > 0. ij ≥ ≥ asA> 0. (We writeA>B to meanA B> 0 etc.) � IfA 0,x> 0, and Ax= 0 thenA= 0. − ≥ =[ ] � If A B , then A B , for any absolute norm ; We also define A a ij . | |≤| | � � ≤ � � �·� | | | | that is, a norm for which A = A . Typical applications where nonnegative or positive matrices � � �| |� occur are problems in which we have matrices where the elements correspond to � probabilities (e.g., Markov chains) � power levels or power gain factors (e.g., in power control for wireless systems). � Non-negative weights/costs in graphs. 17 / 29 18 / 29 Nonnegative matrices: Spectral radius Nonnegative matrices: Spectral radius Lemma: IfA M ,A 0, and if the row sums ofA are Thm: LetA M andA 0. Then ∈ n ≥ ∈ n ≥ constant, thenρ(A) = A . If the column sums are n n ||| ||| ∞ constant, thenρ(A) = A 1. min aij ρ(A) max aij ||| ||| i ≤ ≤ i �j=1 �j=1 n n min aij ρ(A) max aij The following theorem can be used to give upper and lower j ≤ ≤ j = = bounds on the spectral radius of arbitrary matrices. �i 1 �i 1 , ρ( ) ρ( ) ρ( ) Thm: LetA M n andA 0. If Ax=λx andx> 0, then Thm: LetA B M n. If A B, then A A B . ∈ ≥ ∈ | |≤ ≤ | | ≤ λ=ρ(A). 19 / 29 20 / 29 Positive matrices Nonnegative matrices For positive matrices we can say a little more. Generalization of Perron’s theorem to general non-negative matrices? Perron’s theorem: IfA M n andA> 0, then ∈ Thm: IfA M andA 0, then 1. ρ(A)>0 ∈ n ≥ 2. ρ(A) is an eigenvalue ofA 1. ρ(A) is an eigenvalue ofA n 3. There is anx R n withx> 0 such that Ax=ρ(A)x 2. There is a non-zerox R withx 0 such that ∈ ∈ ≥ 4. ρ(A) is an algebraically (and geometrically) simple Ax=ρ(A)x eigenvalue ofA For stronger results, we need a stronger assumption onA. 5. λ <ρ(A) for every eigenvalueλ=ρ(A) ofA | | � 6. [A/ρ(A)]m L asm , whereL= xy T , Ax=ρ(A)x, → →∞ y T A=ρ(A)y T ,x> 0,y> 0, andx T y= 1.
Details
-
File Typepdf
-
Upload Time-
-
Content LanguagesEnglish
-
Upload UserAnonymous/Not logged-in
-
File Pages8 Page
-
File Size-