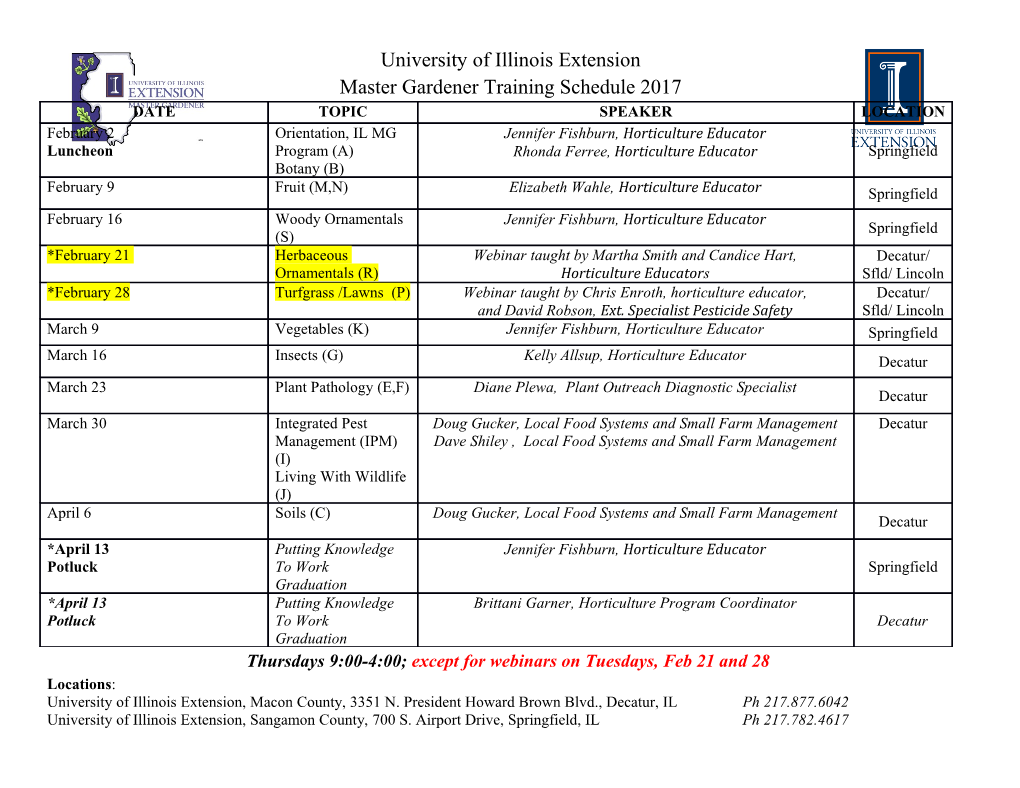
Detecting Non-compositional MWE Components using Wiktionary Bahar Salehi,♣♥ Paul Cook♥ and Timothy Baldwin♣♥ NICTA Victoria Research Laboratory Department♣ of Computing and Information Systems ♥ The University of Melbourne Victoria 3010, Australia [email protected], [email protected], [email protected] Abstract components of a given MWE have a composi- tional usage. Experiments over two widely-used We propose a simple unsupervised ap- datasets show that our approach outperforms state- proach to detecting non-compositional of-the-art methods. components in multiword expressions based on Wiktionary. The approach makes 2 Related Work use of the definitions, synonyms and trans- Previous studies which have considered MWE lations in Wiktionary, and is applicable to compositionality have focused on either the iden- any type of MWE in any language, assum- tification of non-compositional MWE token in- ing the MWE is contained in Wiktionary. stances (Kim and Baldwin, 2007; Fazly et al., Our experiments show that the proposed 2009; Forthergill and Baldwin, 2011; Muzny and approach achieves higher F-score than Zettlemoyer, 2013), or the prediction of the com- state-of-the-art methods. positionality of MWE types (Reddy et al., 2011; 1 Introduction Salehi and Cook, 2013; Salehi et al., 2014). The identification of non-compositional MWE tokens A multiword expression (MWE) is a combina- is an important task when a word combination tion of words with lexical, syntactic or seman- such as kick the bucket or saw logs is ambiguous tic idiosyncrasy (Sag et al., 2002; Baldwin and between a compositional (generally non-MWE) Kim, 2009). An MWE is considered (semanti- and non-compositional MWE usage. Approaches cally) “non-compositional” when its meaning is have ranged from the unsupervised learning of not predictable from the meaning of its compo- type-level preferences (Fazly et al., 2009) to su- nents. Conversely, compositional MWEs are those pervised methods specific to particular MWE con- whose meaning is predictable from the meaning structions (Kim and Baldwin, 2007) or applica- of the components. Based on this definition, a ble across multiple constructions using features component is compositional within an MWE, if its similar to those used in all-words word sense meaning is reflected in the meaning of the MWE, disambiguation (Forthergill and Baldwin, 2011; and it is non-compositional otherwise. Muzny and Zettlemoyer, 2013). The prediction Understanding which components are non- of the compositionality of MWE types has tradi- compositional within an MWE is important in tionally been couched as a binary classification NLP applications in which semantic information task (compositional or non-compositional: Bald- is required. For example, when searching for win et al. (2003), Bannard (2006)), but more re- spelling bee, we may also be interested in docu- cent work has moved towards a regression setup, ments about spelling, but not those which contain where the degree of the compositionality is pre- only bee. For research project, on the other hand, dicted on a continuous scale (Reddy et al., 2011; we are likely to be interested in documents which Salehi and Cook, 2013; Salehi et al., 2014). In ei- contain either research or project in isolation, and ther case, the modelling has been done either over for swan song, we are only going to be interested the whole MWE (Reddy et al., 2011; Salehi and in documents which contain the phrase swan song, Cook, 2013), or relative to each component within and not just swan or song. the MWE (Baldwin et al., 2003; Bannard, 2006). In this paper, we propose an unsupervised ap- In this paper, we focus on the binary classification proach based on Wikitionary for predicting which of MWE types relative to each component of the 1792 Proceedings of the 2014 Conference on Empirical Methods in Natural Language Processing (EMNLP), pages 1792–1797, October 25-29, 2014, Doha, Qatar. c 2014 Association for Computational Linguistics MWE. definition. A given MWE will often have multiple The work that is perhaps most closely related to definitions, however, begging the question of how this paper is that of Salehi and Cook (2013) and to combine across them, for which we propose the Salehi et al. (2014), who use translation data to following three methods. predict the compositionality of a given MWE rel- IRST EF Use only the ative to each of its components, and then combine First Definition (F D ): first-listed Wiktionary definition for the MWE, those scores to derive an overall compositionality based on the assumption that this is the predom- score. In both cases, translations of the MWE and inant sense. its components are sourced from PanLex (Bald- win et al., 2010; Kamholz et al., 2014), and if All Definitions (ALLDEFS): In the case that there is greater similarity between the translated there are multiple definitions for the MWE, cal- components and MWE in a range of languages, culate the lexical overlap for each independently the MWE is predicted to be more compositional. and take a majority vote; in the case of a tie, label The basis of the similarity calculation is unsuper- the component as non-compositional. vised, using either string similarity (Salehi and Cook, 2013) or distributional similarity (Salehi et Idiom Tag (ITAG): In Wiktionary, there is fa- al., 2014). However, the overall method is su- cility for users to tag definitions as idiomatic.1 If, pervised, as training data is used to select the for a given MWE, there are definitions tagged as languages to aggregate scores across for a given idiomatic, use only those definitions; if there are MWE construction. To benchmark our method, no such definitions, use the full set of definitions. we use two of the same datasets as these two pa- pers, and repurpose the best-performing methods 3.2 Synonym-based Definition Expansion of Salehi and Cook (2013) and Salehi et al. (2014) In some cases, a component is not explicitly men- for classification of the compositionality of each tioned in a definition, but a synonym does occur, MWE component. indicating that the definition is compositional in that component. In order to capture synonym- 3 Methodology based matches, we optionally look for synonyms of the component word in the definition,2 and ex- Our basic method relies on analysis of lexical pand our notion of lexical overlap to include these overlap between the component words and the def- synonyms. initions of the MWE in Wiktionary, in the man- For example, for the MWE china clay, the defi- ner of Lesk (1986). That is, if a given component nition is kaolin, which includes neither of the com- can be found in the definition, then it is inferred ponents. However, we find the component word that the MWE carries the meaning of that compo- clay in the definition for kaolin, as shown below. nent. For example, the Wiktionary definition of swimming pool is “An artificially constructed pool A fine clay, rich in kaolinite, used in ce- of water used for swimming”, suggesting that the ramics, paper-making, etc. MWE is compositional relative to both swimming and pool. If the MWE is not found in Wiktionary, This method is compatible with the three we use Wikipedia as a backoff, and use the first definition-based similarity methods described paragraph of the (top-ranked) Wikipedia article as above, and indicated by the +SYN suffix (e.g. a proxy for the definition. FIRSTDEF+SYN is FIRSTDEF with synonym- As detailed below, we further extend the basic based expansion). method to incorporate three types of information found in Wiktionary: (1) definitions of each word 3.3 Translations in the definitions, (2) synonyms of the words in the A third information source in Wiktionary that can definitions, and (3) translations of the MWEs and be used to predict compositionality is sense-level components. translation data. Due to the user-generated na- ture of Wiktionary, the set of languages for which 3.1 Definition-based Similarity 1Although the recall of these tags is low (Muzny and The basic method uses Boolean lexical overlap be- Zettlemoyer, 2013). tween the target component of the MWE and a 2After removing function words, based on a stopword list. 1793 ENC EVPC tionary+Wikipedia for the ENCs, and very high WordNet 91.1% 87.5% coverage for the EVPCs. In both cases, the cov- Wiktionary 96.7% 96.2% erage of WordNet is substantially lower, although Wiktionary+Wikipedia 100.0% 96.2% still respectable, at around 90%. Table 1: Lexical coverage of WordNet, Wik- 4 Datasets tionary and Wiktionary+Wikipedia over our two As mentioned above, we evaluate our method over datasets. the same two datasets as Salehi and Cook (2013) (which were later used, in addition to a third translations are provided varies greatly across lexi- dataset of German noun compounds, in Salehi cal entries. Our approach is to take whatever trans- et al. (2014)): (1) 90 binary English noun com- lations happen to exist in Wiktionary for a given pounds (ENCs, e.g. spelling bee or swimming MWE, and where there are translations in that lan- pool); and (2) 160 English verb particle construc- guage for the component of interest, use the LCS- tions (EVPCs, e.g. stand up and give away). Our based method of Salehi and Cook (2013) to mea- results are not directly comparable with those of sure the string similarity between the translation Salehi and Cook (2013) and Salehi et al. (2014), of the MWE and the translation of the compo- however, who evaluated in terms of a regression nents. Unlike Salehi and Cook (2013), however, task, modelling the overall compositionality of the we do not use development data to select the opti- MWE. In our case, the task setup is a binary clas- mal set of languages in a supervised manner, and sification task relative to each of the two compo- instead simply take the average of the string simi- nents of the MWE.
Details
-
File Typepdf
-
Upload Time-
-
Content LanguagesEnglish
-
Upload UserAnonymous/Not logged-in
-
File Pages6 Page
-
File Size-