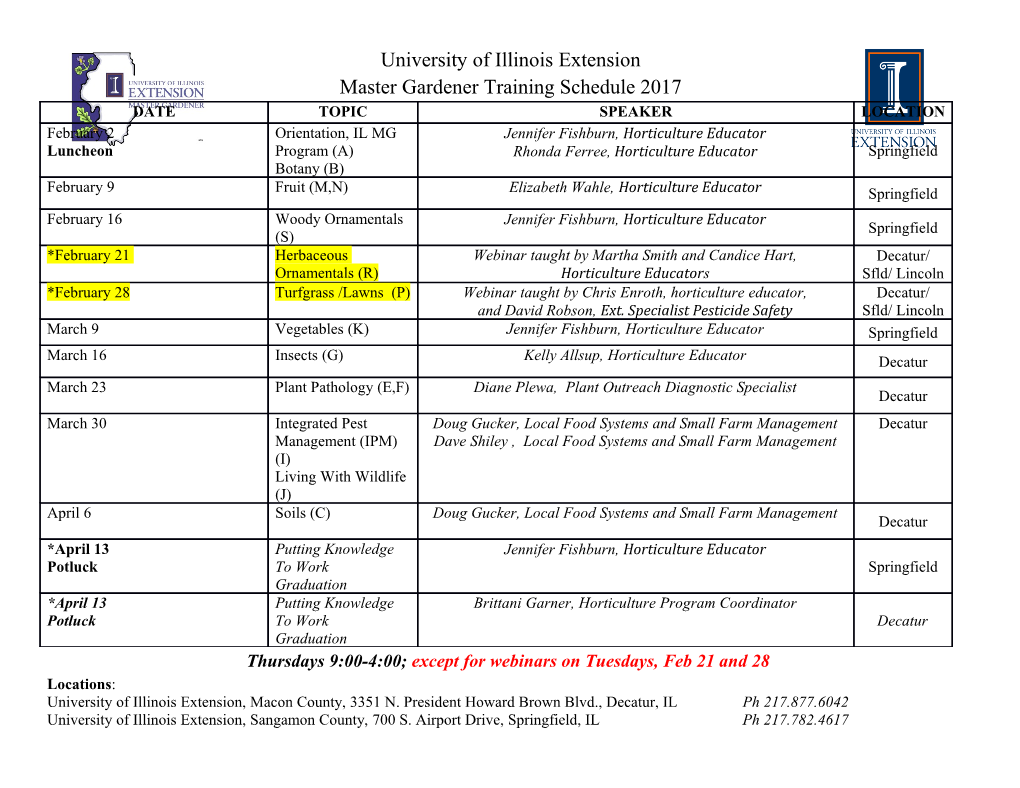
JP1.8 WAVELET ANALYSIS ON VARIABILITY, TELECONNECTIVITY AND PREDICTABILITY OF EAST AFRICA RAINFALL Davison Mwalea, Thian Yew Gana* and Samuel Shenb a Department of Civil and Environment Engineering, University of Alberta, Alberta, Canada b Department of Mathematics, University of Alberta, Alberta, Canada 1. INTRODUCTION In investigating EA rainfall variability and its relationship to other climatic Problems of quantifying rainfall elements it has become imperative to variability in space with respect to climatic analyze the irregularly distributed events in elements such as SST, winds, air time that depict non-stationary power at temperature and specific humidity have many different frequencies. been a subject of numerous studies for To meet this requirement, this study Eastern Africa, (EA) (e.g., Mutai et al. 1998; used wavelet analysis and wavelet based 2002; Ntale et al. 2003), and other studies empirical orthogonal function analysis as summarized in Beltrando (1990). (WEOF), also known as wavelet principal Following some of these studies, EA has component analysis (WLPCA) and wavelet been divided into between 6 and 20 zones independent component analysis (WLICA) of homogenous rainfall variability, found to to study the variability, teleconnectivity and teleconnect to SST variability in the Atlantic, predictability of EA rainfall. In this study, two Indian and Pacific oceans, (Mutai et al. sets of information from the wavelet spectra 1998; Ntale et al. 2003) and atmospheric are used. These are (1) energy coefficients signals, Philippon et al. (2002). Many of (also known as power) of individual scales of these studies applied a combination of time the wavelet spectra, and (2) the average domain multivariate statistics such as wavelet spectra energy over some harmonic analysis, Fourier analysis, linear significant scales (called scale averaged regressions and cross correlations to climate wavelet power, SAWP). Combinations of data to determine relationships between and empirical orthogonal function analysis and among the climate elements. A common and wavelets analysis have previously been well-recognized shortcoming of such applied to multivariate statistical process approaches has been the assumption that monitoring (e.g., Bakshi 1998). climate data is stationary and linear, a criterion few data sets from natural 2. DATA AND METHODS. phenomena satisfy (Huang et al., 1998). With unstable relationships between EA has two rainy seasons, the rainfall in EA and SST in the oceans and September-November (SON) and March- atmospheric circulation fields highlighted by May (MAM). Monthly rainfall data (1950- erratic rainfall between 1965 and 1997, one 1995) from 21 grid locations at a resolution of the major challenges for meteorologists of 2.5°×3.75° latitude and longitude was has been to predict the nature of this extracted for EA (4ºS-12ºS, 28ºE-44ºE), see variability in the rainfall of EA over different Fig 1.The rainfall data was provided by the spatial and temporal scales. Potts (1971), UK meteorological office. and Rodhe and Virji (1996) showed that The monthly rainfall data was oscillatory peaks of 2-2.5, 3.5 and 5.6 years transformed into seasonal data by summing in addition to other peaks associated with EL the monthly values for each grid location. Nino (e.g., Ropelewski and Halpert 1987) Monthly SST anomaly grid data (1950-1995) exist in EA rainfall. However, it has never at 5o x 5o latitude and longitude resolution been clear as to when these oscillations was extracted from the Indian (20ºN-40ºS, occurred in the rainfall. 40ºE-105ºE) and Atlantic (10ºN-30ºS, 50ºW- 10ºE) Ocean. This data was transformed *Corresponding Author address: Thian Yew Gan, Univ. into seasonal and annual data by computing of Alberta, Dept of Civil and Environmental Engineering, 3-month averages for JFM, AMJ, JAS and Edmonton, Alberta AB T6G 2G7, Canada; Email: [email protected] SON and annual averages, respectively. The SST dataset is part of MOHSST6 and 1 was provided by the UK meteorological continuous or a discrete values. The office. quantity a −1/ 2 in Equation (2) is an energy normalization term, which ensures that energy of the mother, and daughter wavelets remain the same over all scales and making it possible to directly compare wavelet transforms of one time series with another (Torrence and Compo, 1998). The wavelet transform of a real signal X (t) with respect to the mother wavelet is a convolution integral given as 1 T t − b W ()b,a = X ()t Ψ * dt (3) a ∫0 a where ψ* is the complex conjugate of ψ. In Fig. 1. (a) Study location (b) detailed this equation, W (b,a) is a wavelet location and description of EA (Uganda, spectrum, a matrix of energy coefficients of Kenya and Tanzania) the decomposed time series X (t). A faster and much more efficient way to compute the wavelet transform is done in the Fourier 2.2 Wavelet Analysis space using the Fourier transform of a discrete time series, X (t), as Wavelets are a set of limited duration waves, also called daughter T ∩ ∩ * wavelets, because they are formed by iω nδt k (4) dilations and translations of a single Wt ()a = ∑ X Ψ (sω k )e prototype wavelet function ψ(t), where t is k =0 real valued, called the basic or mother where the caret symbolizes Fourier wavelet (Castleman, 1996). The mother Transform, k is the frequency index (0,…T) wavelet designed to oscillate like a wave, is and Ψ (sω ) is the Fourier transform of the required to span an area that sums to zero, k wavelet function. The wavelet spectrum was and die out rapidly to zero as t tends to computed using a discrete set of 20 scales infinity to satisfy the so called “admissibility” starting at 2 years in fractional power of two condition. using Ψ()t dt = 0 (1) jδj ∫ s j = so 2 (5) A set of wavelets can be generated by where s0 is twice the sampling rate,j translating and scaling the basic wavelet as =0,1,….20, and δj = 0.25, thus giving follows scales(periods) ranging from 2 to 64 years. The wavelet transform of a time series 1 t − b contains a wealth of information, which can Ψa,b ()t = Ψ (2) a a be condensed over a range of scales to construct the scale averaged wavelet power (SAWP) and used in multivariate analysis. where the scale (width) of the wavelet and 2 translated position along the t-axis are a and j2 W a 2 δ jδ t t ( j ) (6) b respectively. By continuously varying a Wt = ∑ long b, a picture is constructed depicting Cδ j= j1 a j how the energy over various frequencies where Cδ is the reconstruction factor that varies with time. The parameters a and b takes on values depending on the mother are real and a, always positive, may take 2 wavelet used, δj is a factor for scale makes the extracted components as averaging and δt is the sampling period. The independent as possible. To obtain the initial global wavelet spectrum shows dominant most important independent components, oscillations present in a time series. The pre-whitening, a process of projecting the local wavelet power shows how the original data into a subspace spanned by dominant oscillations vary with time. To the first few PCs is employed. Using these examine whether two remotely located projections optimization algorithms are used multivariate time series are related to each to estimate the independent components. other or if one modulates the other over a ICA is considered an extension to PCA and number of scales, the individual scale power used in this study as a complementary tool or the SAWP can be constructed by varying to EOF analysis, to allow the underlying the scale, a, and computing the weighted structure of the data to be more readily sum of the wavelet power over those scales. observed. Using the results of global spectra computed for some selected SST fields located in the 2.5 Artificial Neural Network driven Indian and Atlantic Oceans and rainfall fields by Genetic Algorithm (ANN-GA) located in EA, we extracted energy of some for rainfall prediction individual scale and SAWP from the 2-8 year range. To compute the wavelet power The Genetic algorithm calibrated for this study, the Morlet wavelet (k = 6), neural network used in this study (Fig not was used because its structure resembles shown) consists of a population of feed that of a rainfall time series. forward neural networks embedded in a Genetic algorithm routine. The ANN Ψ (t) = π-1/4ei6te-t2/2 (7) parameters are iteratively improved via genetic evolution (selection, crossover and 2.3 Wavelet Empirical Orthogonal mutation) to more accurately model the joint Function Analysis (WEOF). SST-rainfall variability. The objective function used is a combination of the Empirical Orthogonal Functions Pearson correlation and the root mean analysis (EOF) has been widely used (e.g. square error (RMSE). For each neural Kutzbach, 1967) for analyzing spatial and network of the population, the predictand, y, temporal variability of physical fields to is obtained as a nonlinear translation of the objectively identify the spatially uncorrelated weighted average of the PCs of raw data, x modes of variability of a given field. In this study EOF is used on individual scale power 2 1 1 2 y = f w f w x + b + b (8) or SAWP. Since the WLPCs are obtained 2 ∑ ( 1 ∑ ( ) ) from SAWP, they are interpreted as ‘frequency compacted’ energy variability. To where x is the standardized anomaly and w1 identify and delineate temporally and and w2 are weights for each solution to the spatially uncorrelated patterns at regional hidden and the output layers respectively, scale, we applied the WEOF analysis on and b1 and b2 are the bias vectors SAWP of the SST of the South Atlantic and associated with the hidden and output Indian Ocean and rainfall time series of EA.
Details
-
File Typepdf
-
Upload Time-
-
Content LanguagesEnglish
-
Upload UserAnonymous/Not logged-in
-
File Pages6 Page
-
File Size-