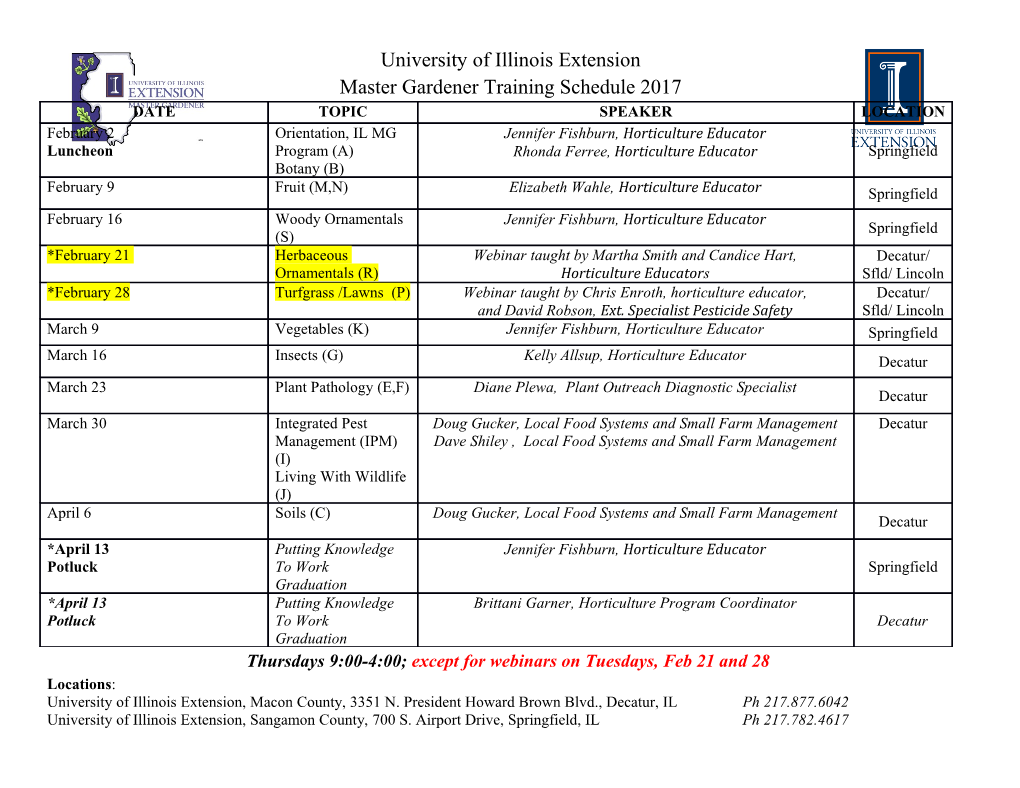
Maximum Likelihood Inference in Weakly Identied DSGE Models. By Isaiah Andrews1 and Anna Mikusheva 2 3 First version: April 14, 2011 This version: May 21, 2012 Abstract This paper examines the issue of weak identication in maximum likelihood, motivated by problems with estimation and inference in a multi-dimensional, non-linear DSGE model. We suggest a test for a simple hypothesis concerning the full parameter vector which is robust to weak identication. We also suggest a test for a composite hypothesis regarding a sub-vector of parameters. The suggested subset test is shown to be asymptotically exact when the nuisance parameter is strongly identied, and in some cases when the nuisance parameter is weakly iden- tied. We pay particular attention to the question of how to estimate Fisher information, and make extensive use of martingale theory. Key words: weak identification, maximum likelihood, score test, C(®)¡ test 1 Introduction In recent years we have witnessed the rapid growth of the empirical literature on the highly parameterized, micro-founded macro models known as Dynamic Stochastic Gen- eral Equilibrium (DSGE) models. A number of papers in this literature have considered estimating these models by maximum likelihood (see for example Ingram, Kocherlakota and Savin (1994), Ireland (2004), Lindé (2005), and McGrattan, Rogerson and Wright (1997)). More recently, Bayesian estimation has become increasingly popular, due in large part to the diculty of maximum likelihood estimation in many DSGE models. As 1Department of Economics, M.I.T., 50 Memorial Drive, Building E52, Cambridge, MA, 02142. Email: [email protected]. Financial support from the Ford Foundation and the NSF is gratefully acknowledged. 2Department of Economics, M.I.T., 50 Memorial Drive, Building E52, Cambridge, MA, 02142. Email: [email protected]. Financial support from the Castle-Krob Career Development Chair is gratefully acknowledged. 3We would like to thank Patrik Guggenberger, Ulrich Muller, Whitney Newey, Zhongjun Qu, Jim Stock, and seminar participants at the Winter Econometric Society Meeting in Chicago, Boston College, Canadian Econometric Study Group, Columbia, Harvard-MIT, NBER summer institute, Rice, Texas A& M, UC Berkeley, UC San Diego, UPenn and U Virginia for helpful comments. We would also like to thank Frank Kleibergen and Sophocles Mavroeidis for informative discussion of their approach in GMM. We are grateful to Lynda Khalaf for guidance on implementing the procedure of Dufour, Khalaf and Kichian (2009). 1 Fernández-Villaverde (2010) points out in his survey of DSGE estimation, "likelihoods of DSGE models are full of local maxima and minima and of nearly at surfaces... the standard errors of the estimates are notoriously dicult to compute and their asymptotic distribution a poor approximation to the small sample one." The poor performance of maximum likelihood estimation has fueled growing concerns about poor identication in many DSGE models (see Canova and Sala (2009), Guerron-Quintana, Inoue and Kilian (2009), Iskrev (2010), and Mavroeidis (2005)). In this paper, we consider the problem of weak identication in models estimated by maximum likelihood, taking DSGE models as a leading empirical example. Weak identi- cation arises when the amount of information in the data about some parameter or group of parameters is small and is generally modeled in such a way that information about parameters accumulates slowly along some dimensions. This leads to the breakdown of the usual asymptotics for maximum likelihood, with the asymptotic distributions for the maximum likelihood estimator and the standard LR, LM, and Wald statistics providing a poor approximation to their nite sample behavior. This is distinct from loss of point identication. We assume throughout that the models we consider are point identied, and thus that changing the value of any parameter changes the distribution of the data, though the eect will be small for some parameters. We focus on the problem of testing and condence set construction in this context. In our view there are two main approaches to inference in models where identication may be weak. One is to create a two-step procedure, where one rst dierentiates (via a pre-test) between weakly and strongly identied models and then chooses a procedure based on the test result. We take the other approach. Rather than looking for a test for weak identication as such, we instead attempt to construct a test for parameters that is robust to weak identication. The ideal procedure should satisfy two conditions. First, it should control size well if identication is weak, and second, it should be asymptotically equivalent to the classical ML tests if identication is strong. If such a procedure exists, it renders pretests unnecessary and, in general, inferior given the size problems endemic to multiple testing procedures. We view this approach as analogous to the modern treatment of testing in the presence of potential heteroscedasticity. While in the past it was common to use pretests for heteroscedasticity, current empirical practice is to simply use standard errors (such as those of White (1980)) which are correct asymptotically regardless of whether or not the 2 data is heteroscedastic. Likewise, in weak instrumental variables regression (weak IV) there are tests available that have correct asymptotic size under weak identication and (at least for the case of one endogenous variable) at least as much power as the classical procedures under strong identication. We consider two dierent tasks. First, we examine the problem of testing a simple hypothesis on the full parameter vector. We suggest using a particular form of the classical Lagrange Multiplier (LM) test, which we show is robust to weak identication. The assumptions needed for this result are extremely weak and cover a large number of cases, including weak IV, an ARMA(1,1) with nearly canceling roots, a weakly identied binary choice model and weakly identied exponential family models, for example VARs with weakly identied structural parameters. The proof for this test makes extensive use of martingale theory, particularly the fact that the score (i.e. the gradient of the log likelihood) is a martingale when evaluated at the true parameter value. Second, we turn to the problem of testing a subset of parameters without restrict- ing the remaining parameters. Creation of such tests is critical for the construction of condence sets, given that the common practice in applied work is to report a sepa- rate condence interval for each element of the parameter vector. Constructing a test satisfying our rst requirement, that is, one that controls size well under weak iden- tication, is straightforward using the LM test for the full parameter vector and the projection method. However, simultaneously satisfying the second condition, asymptotic equivalence to the classical tests under strong identication, is a much more challenging problem which has not been fully solved even for many simpler models. The test we suggest for a subset of parameters is a form of Rao's score test and is asymptotically equivalent to Neyman's C(®) test when identication is strong. We show that the suggested test has a Â2 asymptotic distribution as long as the nuisance parameter (i.e. the part of the parameter vector which we are not testing) is strongly identied, without any assumption about the strength of identication of the tested parameter. We also show that the suggested test has correct asymptotic size in some cases where the nuisance parameter is weakly identied. By combining our procedure for concentrating out nuisance parameters that are strongly identied with projection over the remaining nuisance parameters, one obtains a weak identication-robust test more powerful than existing procedures. 3 Relation to the Literature on Weak Identication The literature on weak iden- tication is quite large. The most studied and best understood case is that of weak instrumental variables estimation. For a comprehensive survey of the literature on this topic, see Stock, Wright, and Yogo (2002). The weak identication framework was gen- eralized to GMM by Stock and Wright (2000), who represented weak identication using an asymptotic embedding in which the objective function becomes at along some di- mensions as the sample grows. An alternative embedding assumes a drifting sequence of parameters, as in Andrews and Cheng (2009). While we make use of similar embeddings to demonstrate the applicability of our assumptions in examples, they are in no way necessary for our results, and we remain quite agnostic about the process generating the data. Our paper diers from the existing weak identication-robust literature in several respects. First, we consider maximum likelihood, which has not been well-studied in the theoretical econometric literature on weak identication, with the exception of the recent paper by Andrews and Cheng (2011). The results from the weak GMM literature do not in general apply to composite hypothesis testing in the ML context, as the variance of the moment condition (the score of the log-likelihood) becomes degenerate asymptotically, violating a key assumption in this literature. Second, we point out a previously unno- ticed link between weak identication and the breakdown of the asymptotic equivalence between two common ways of estimating Fisher information. Third, we provide the rst example of a model in which concentrating out a weakly identied nuisance parameter results in an asymptotically similar test. There are several papers in the weak identi- cation literature that show that one can concentrate out a strongly identied nuisance parameter in a particular context and get an asymptotically similar test, as for example in Guggenberger and Smith (2005) and Kleibergen (2005). However, we are not aware of any results that show that one can concentrate out a weakly identied nuisance param- eter and obtain a standard limiting distribution. On the contrary, Andrews and Cheng (2009) show that the presence of a weakly identied nuisance parameter usually leads to non-standard asymptotic behavior, even for strongly identied parameters.
Details
-
File Typepdf
-
Upload Time-
-
Content LanguagesEnglish
-
Upload UserAnonymous/Not logged-in
-
File Pages48 Page
-
File Size-