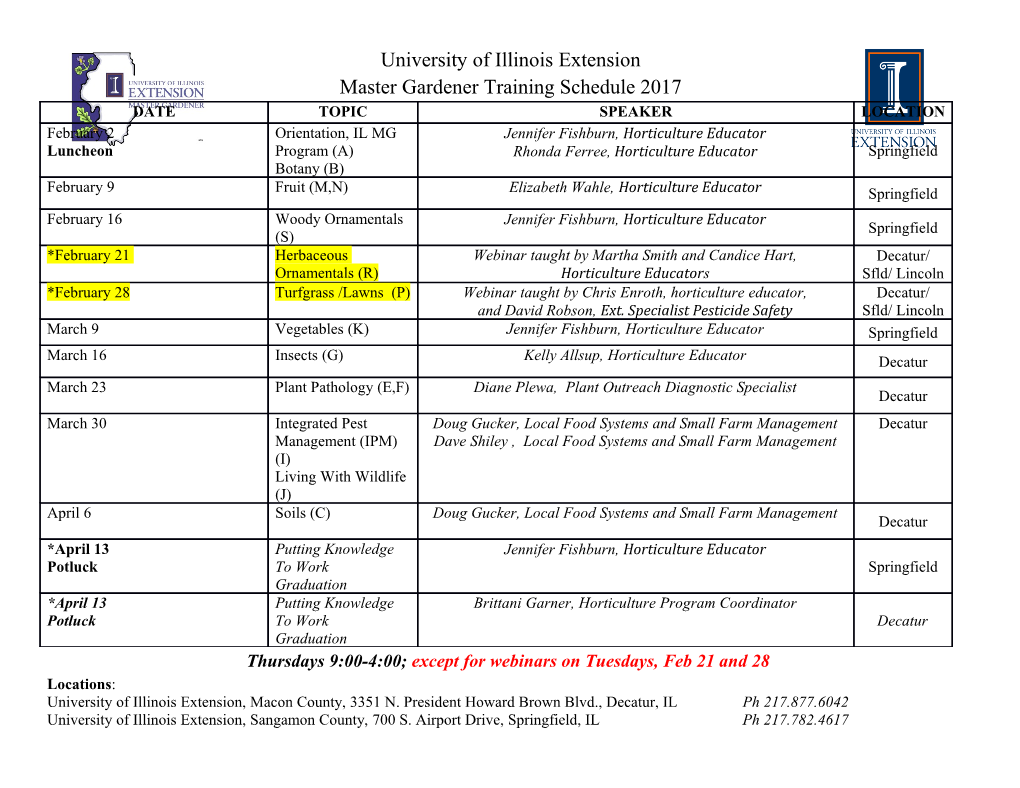
Dai et al. BMC Systems Biology 2012, 6(Suppl 1):S17 http://www.biomedcentral.com/1752-0509/6/S1/S17 RESEARCH Open Access Integrating many co-splicing networks to reconstruct splicing regulatory modules Chao Dai1,2†, Wenyuan Li2†, Juan Liu1, Xianghong Jasmine Zhou2* From The 5th IEEE International Conference on Computational Systems Biology (ISB 2011) Zhuhai, China. 02-04 September 2011 Abstract Background: Alternative splicing is a ubiquitous gene regulatory mechanism that dramatically increases the complexity of the proteome. However, the mechanism for regulating alternative splicing is poorly understood, and study of coordinated splicing regulation has been limited to individual cases. To study genome-wide splicing regulation, we integrate many human RNA-seq datasets to identify splicing module, which we define as a set of cassette exons co-regulated by the same splicing factors. Results: We have designed a tensor-based approach to identify co-splicing clusters that appear frequently across multiple conditions, thus very likely to represent splicing modules - a unit in the splicing regulatory network. In particular, we model each RNA-seq dataset as a co-splicing network, where the nodes represent exons and the edges are weighted by the correlations between exon inclusion rate profiles. We apply our tensor-based method to the 38 co-splicing networks derived from human RNA-seq datasets and indentify an atlas of frequent co-splicing clusters. We demonstrate that these identified clusters represent potential splicing modules by validating against four biological knowledge databases. The likelihood that a frequent co-splicing cluster is biologically meaningful increases with its recurrence across multiple datasets, highlighting the importance of the integrative approach. Conclusions: Co-splicing clusters reveal novel functional groups which cannot be identified by co-expression clusters, particularly they can grant new insights into functions associated with post-transcriptional regulation, and the same exons can dynamically participate in different pathways depending on different conditions and different other exons that are co-spliced. We propose that by identifying splicing module, a unit in the splicing regulatory network can serve as an important step to decipher the splicing code. Background splicing code and understand the mechanism of how an Alternative splicing provides an important means for exon is alternatively spliced in tissue-specific manner. generating proteomic diversity. Recent estimates indicate A central concept in transcription regulation is the that nearly 95% of human multi-exon genes are alterna- transcription module, defined as a set of genes that are tively spliced [1]. The mechanism for regulating alterna- co-regulated by the same transcription factor(s). Analo- tive splicing is still poorly understood, and its gously, such coordinated regulation also occurs at the complexity attributes to the combinatorial regulation of splicing level [4-6]. For example, the splicing factor many factors, e.g. splicing factors, cis-regulatory ele- Nova regulates exon splicing of a set of genes that shape ments, and RNA secondary structure [2,3]. A fundamen- the synapse [6]. However, the study of such coordinated tal task of alternative splicing research is to decipher splicing regulation has thus far been limited to indivi- dual cases [5-9]. In this paper, we define a splicing mod- ule as a set of exons that are regulated by the same * Correspondence: [email protected] splicing factors. The exons in a splicing module can † Contributed equally 2Molecular and Computational Biology, Department of Biological Sciences, belong to different genes, but they exhibit correlated University of Southern California, Los Angeles, CA 90089, USA splicing patterns (in terms of being included or excluded Full list of author information is available at the end of the article © 2012 Dai et al.; licensee BioMed Central Ltd. This is an Open Access article distributed under the terms of the Creative Commons Attribution License (http://creativecommons.org/licenses/by/2.0), which permits unrestricted use, distribution, and reproduction in any medium, provided the original work is properly cited. Dai et al. BMC Systems Biology 2012, 6(Suppl 1):S17 Page 2 of 9 http://www.biomedcentral.com/1752-0509/6/S1/S17 in their respective transcripts) across different condi- j. This weight represents the correlation between the tions, thus form an exon co-splicing cluster. two exons’ inclusion rate profiles. Given m co-splicing The recent development of RNA-seq technology pro- networks with the same n nodes but different edge vides a revolutionary tool to study alternative splicing. weights, we can represent the whole system as a 3rd- From each RNA-seq dataset, we can derive not only the order tensor (or 3-dimensional array) of size n × n × m. expression levels of genes, but also those of exons and An element aijk of the tensor is the weight of the edge transcripts (i.e., splicing isoforms). Given an RNA-seq between nodes i and j in the kth network (Figure 1). A dataset containing a set of samples, we can calculate the co-splicing cluster appears as a heavy subgraph in the inclusion rate of each exon (In this study we only con- co-splicing network, which in turn corresponds to a sider cassette exons, which are common in alternative heavy region in the adjacency matrix. A frequent co-spli- splicing events. Henceforth, the term “exon” always cing cluster is one that appears in multiple datasets, and means “cassette exons”.) In every sample, as the ratio appears as a heavy region of the tensor (Figure 1). Thus, between its expression level and that of the host gene. A the problem of identifying frequent co-splicing clusters recent study provided a nice example of studying spli- can intuitively be formulated as the problem of identify- cing regulatory relationships using a network of exon- ing heavy subtensors in a tensor. By representing net- exon, exon-gene, and gene-gene links [10]. Here, we works and formulating the problem in this tensor form, construct from each RNA-seq dataset a weighted co-spli- we gain access to a wealth of established optimization cing network where the nodes represent exons and the methods for multidimensional arrays. Reformulating a edge weights are correlations between the inclusion discrete graph discovery problem as a continuous opti- ratesoftwoexonsacrossallsamplesinthedataset. mization problem is a longstanding tradition in graph While directly comparing the inclusion rates for the theory. There are many successful examples, such as same exon in different datasets could be biased by plat- using a Hopfield neural network to solve the traveling forms and protocols, the correlations between inclusion salesman problem [13] and applying the Motzkin-Straus rates for a given exon pair are comparable across data- theorem to the clique-finding problem [14]. Moreover, sets. From a series of RNA-seq datasets, we can there- when a graph-based pattern mining problem is trans- fore derive a series of co-splicing networks, which can formed into a continuous optimization problem, it be subjected to comparative network analysis and pro- becomes easy to incorporate constraints representing vide an effective way to integrate a large number of prior knowledge. Finally, advanced continuous optimiza- RNA-seq experiments conducted in different labora- tion techniques require very few ad hoc parameters, in tories and using different technology platforms. contrast with most heuristic graph combinatorial A heavy subgraph in a weighted co-splicing network algorithms. represents a set of exons that are highly correlated in We applied our tensor algorithm to 38 weighted their inclusion rate profiles; i.e., they are co-spliced. A exon co-splicing networks derived from human RNA- set of exons which frequently form a heavy subgraph in seq datasets. We identified an atlas of frequent co- multiple datasets are likely to be regulated by the same splicing clusters and validated them against four biolo- splicing factors, and thus form a splicing module. We gical knowledge bases: Gene Ontology annotations, call such patterns frequent co-splicing clusters.Dueto RNA-binding motif database, 191 ENCODE genome- the enhanced signal to noise separation, frequent clus- wide ChIP-seq profiles, and protein complex database. ters are more robust and are more likely to be regulated We demonstrate that the likelihood for an exon clus- by the same splicing factors (thus more likely to repre- ter to be biologically meaningful increases with its sent splicing modules) than those heavy subgraphs recurrence across multiple datasets, highlighting the derived from a single dataset. In our previous research benefit of the integrative approach. Moreover, we [11], we showed that the likelihood for a gene co- show that co-splicing clusters can reveal novel func- expression cluster to be a transcription module increases tional groups that cannot be identified by co-expres- significantly with the recurrence of clusters in multiple sion clusters. Finally, we show that the same exons datasets. A similar principle applies to splicing modules. can dynamically participate in different pathways, In this paper, we adopt our recently developed tensor- depending on different conditions and different other based approach to find the heavy subgraph that fre- exons that are co-spliced. quently occur in multiple weighted networks [12]. Our goal here is to identify co-spliced exon clusters that fre- Results quently occur across multiple weighted co-splicing net- We identified 38 human RNA-seq datasets from the works. A co-splicing network of n nodes (exons) can be NCBI Sequence Read Archive (http://www.ncbi.nlm. represented as an n × n adjacency matrix A, where ele- nih.gov/sra) (see additional file 1: Section S6 to get ment aij is the weight of the edge between nodes i and details of these datasets), each with at least 6 samples Dai et al. BMC Systems Biology 2012, 6(Suppl 1):S17 Page 3 of 9 http://www.biomedcentral.com/1752-0509/6/S1/S17 Figure 1 Illustration of the 3rd-order tensor representation of a collection of networks.
Details
-
File Typepdf
-
Upload Time-
-
Content LanguagesEnglish
-
Upload UserAnonymous/Not logged-in
-
File Pages9 Page
-
File Size-