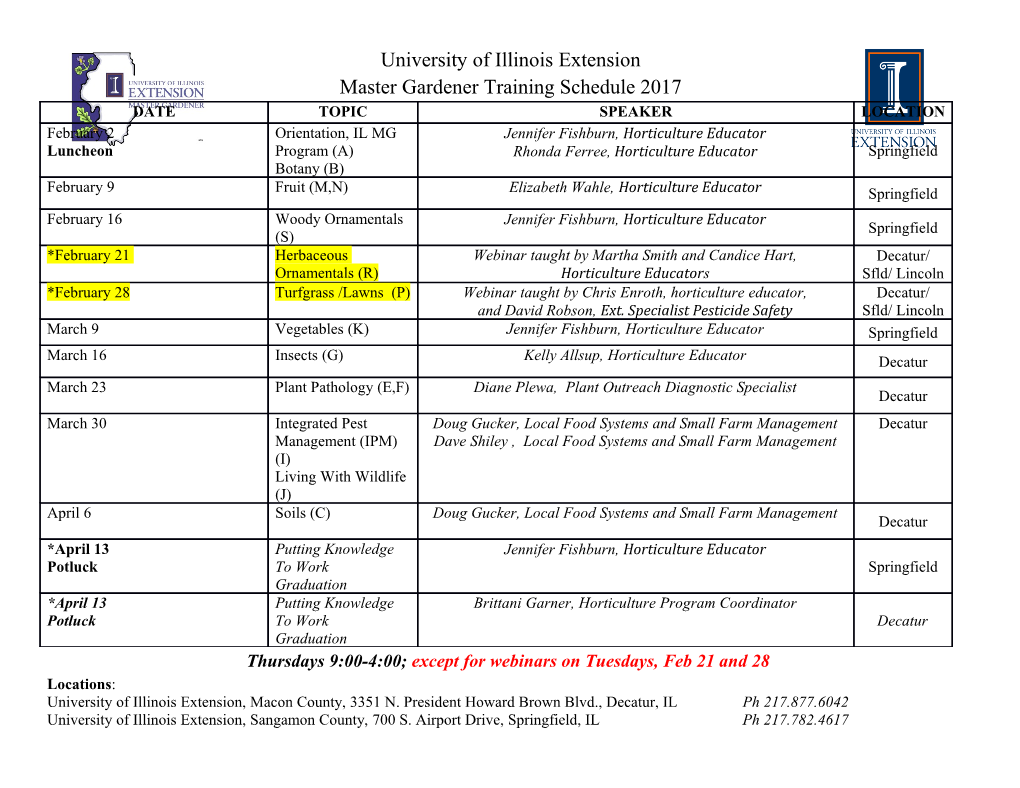
i “book” — 2017/4/1 — 12:47 — page 91 — #93 i i i Embree – draft – 1 April 2017 3 Singular Value Decomposition · Thus far we have focused on matrix factorizations that reveal the eigenval- ues of a square matrix A n n,suchastheSchur factorization and the 2 ⇥ Jordan canonical form. Eigenvalue-based decompositions are ideal for ana- lyzing the behavior of dynamical systems likes x0(t)=Ax(t) or xk+1 = Axk. When it comes to solving linear systems of equations or tackling more general problems in data science, eigenvalue-based factorizations are often not so il- luminating. In this chapter we develop another decomposition that provides deep insight into the rank structure of a matrix, showing the way to solving all variety of linear equations and exposing optimal low-rank approximations. 3.1 Singular Value Decomposition The singular value decomposition (SVD) is remarkable factorization that writes a general rectangular matrix A m n in the form 2 ⇥ A = (unitary matrix) (diagonal matrix) (unitary matrix)⇤. ⇥ ⇥ From the unitary matrices we can extract bases for the four fundamental subspaces R(A), N(A), R(A⇤),andN(A⇤), and the diagonal matrix will reveal much about the rank structure of A. We will build up the SVD in a four-step process. For simplicity suppose that A m n with m n.(Ifm<n, apply the arguments below 2 ⇥ ≥ to A n m.) Note that A A n n is always Hermitian positive ⇤ 2 ⇥ ⇤ 2 ⇥ semidefinite. (Clearly (A⇤A)⇤ = A⇤(A⇤)⇤ = A⇤A,soA⇤A is Hermitian. For any x n,notethatx A Ax =(Ax) (Ax)= Ax 2 0,soA A is 2 ⇤ ⇤ ⇤ k k ≥ ⇤ positive semidefinite.) 91 i i i i i “book” — 2017/4/1 — 12:47 — page 92 — #94 i i i 92 Chapter 3. Singular Value Decomposition Step 1. Using the spectral decomposition of a Hermitian matrix discussed in Section 1.5, A A has n eigenpairs (λ , v ) n with orthonormal unit ⇤ { j j }j=1 eigenvectors (v v =1, v v =0when j = k). We are free to pick any j⇤ j j⇤ k 6 convenient indexing for these eigenpairs; label the eigenvalues in decreasing magnitude, λ λ λ 0. 1 ≥ 2 ≥···≥ n ≥ Step 2. Define s := Av . j k jk Note that s2 = Av 2 = v A Av = λ . Since the eigenvalues λ ,...,λ j k jk j⇤ ⇤ j j 1 n are decreasing in magnitude, so are the s values: s s s 0. j 1 ≥ 2 ≥···≥ n ≥ Step 3. Next, we will build a set of related orthonormal vectors in m. Suppose we have already constructed such vectors u1,...,uj 1. − 1 1 If s =0, then define u = s− Av ,sothat u = s− Av =1. j 6 j j j k jk j k jk If sj =0, then pick uj to be any unit vector such that uj span u1,...,uj 1 ?; 2 { − } 1 i.e., ensure uj⇤uk =0for all k<j. By construction, u u =0for j = k if s or s is zero. If both s and s j⇤ k 6 j k j k are nonzero, then 1 1 λk uj⇤uk = (Avj)⇤(Avk)= vj⇤A⇤Avk = vj⇤vk, sjsk sjsk sjsk where we used the fact that v is an eigenvector of A A.Nowifj = k, j ⇤ 6 then vj⇤vk =0, and hence uj⇤uk =0. On the other hand, j = k implies that 2 vj⇤vk =1,souj⇤uk = λj/sj =1. In conclusion, we have constructed a set of orthonormal vectors u n { j}j=1 with u m. j 2 Step 4. For all j =1,...,n, Avj = sjuj, regardless of whether sj =0or not. We can stack these n vector equations as columns of a single matrix equation, || | || | Av Av Av = s u s u s u . 2 1 2 ··· n 3 2 1 1 2 2 ··· n n 3 || | || | 4 5 4 5 1 If sj =0,thenλj =0,andsoA⇤A has a zero eigenvalue; i.e., this matrix is singular. Embree – draft – 1 April 2017 i i i i i “book” — 2017/4/1 — 12:47 — page 93 — #95 i i i 3.1. Singular Value Decomposition 93 Note that both matrices in this equation can be factored into the product of simpler matrices: s1 s || | || |2 2 3 A v1 v2 vn = u1 u2 un . 2 ··· 3 2 ··· 3 .. 6 7 || | || |6 s 7 4 5 4 5 6 n 7 4 5 Denote these matrices as AV = U⌃, where A m n, V n n, U 2 ⇥ 2 ⇥ 2 m n,and⌃ n n. ⇥ 2 ⇥ The (j, k) entry of V⇤V is simplyb b vj⇤vk,andsoV⇤V = I. Since Vbis a square matrix,b we have just proved that it is unitary, and hence, VV⇤ = I as well. We conclude that A = U⌃V⇤. This matrix factorization is known asb theb reduced singular value decomposi- tion or the economy-sized singular value decomposition (or, informally, the skinny SVD). It can be obtained via the MATLAB command [Uhat, Sighat, V] = svd(A,’econ’); The Reduced Singular Value Decomposition Theorem 3.1. Any matrix A m n with m n can be written as 2 ⇥ ≥ A = U⌃V⇤, m n n n where U ⇥ has orthonormalb columns,b V ⇥ is unitary, and 2 2 ⌃ = diag(s ,...,s ) n n has real nonnegative decreasing entries. 1 n 2 ⇥ The columnsb of U are left singular vectors,thecolumnsofV are right singularb vectors,andthevaluess1,...,sn are the singular values. While the matrix U has orthonormal columns, it is not a unitary matrix when m>n. In particular, we have U U = I n n,but ⇤ 2 ⇥ b m m UU⇤ b b ⇥ 2 cannot be the identity unless mb b= n. (To see this, note that UU⇤ is an orthogonal projection onto R(U) = span u ,...,u . Since dim(R(U)) = { 1 n} n, this projection cannot equal the m-by-m identity matrix whenbm>nb .) b b Embree – draft – 1 April 2017 i i i i i “book” — 2017/4/1 — 12:47 — page 94 — #96 i i i 94 Chapter 3. Singular Value Decomposition Though U is not unitary, it is subunitary. We can construct m n − additional column vectors to append to U to make it unitary. Here is the recipe: For jb= n +1,...,m,pick b uj span u1,...,uj 1 ? 2 { − } with uj⇤uj =1. Then define || | U = u u u . 2 1 2 ··· m 3 || | 4 5 Confirm that U U = UU = I m m, showing that U is unitary. ⇤ ⇤ 2 ⇥ We wish to replace the U in the reduced SVD with the unitary matrix U. To do so, we also need to replace ⌃ by some ⌃ in such a way that U⌃ = U⌃. The simplest approachb constructs ⌃ by appending zeros to the end of ⌃, thus ensuring there is no contributionb when the new entries of U multiplyb b against the new entries of ⌃: b ⌃ m n ⌃ = ⇥ . 0 2 b The factorization A = U⌃V⇤ is called the full singular value decomposition. The Full Singular Value Decomposition Theorem 3.2. Any matrix A m n can be written in the form 2 ⇥ A = U⌃V⇤, where U m m and V n n are unitary matrices and ⌃ m n 2 ⇥ 2 ⇥ 2 ⇥ is zero except for the main diagonal; The columns of U are left singular vectors,thecolumnsofV are right singular vectors,andthevaluess1,...,smin m,n are the singular values. { } A third version of the singular value decomposition is often very helpful. Start with the reduced SVD A = U⌃V⇤ in Theorem 3.1 Multiply U⌃ together to get b b b b v1⇤ n || . A = U⌃V⇤ = s u s u . = s u v⇤, 2 1 1 ··· n n 3 2 . 3 j j j v j=1 ||6 n⇤ 7 X b b 4 5 4 5 Embree – draft – 1 April 2017 i i i i i “book” — 2017/4/1 — 12:47 — page 95 — #97 i i i 3.1. Singular Value Decomposition 95 which renders A as the sum of outer products u v m n,weightedby j j⇤ 2 ⇥ nonnegative numbers sj. Let r denote the number of nonzero singular values, so that if r<n, then s = = s =0. r+1 ··· n Thus A can be written as r A = sjujvj⇤, (3.1) Xj=1 known as the dyadic form of the SVD. The Dyadic Form of the Singular Value Decomposition Theorem 3.3. For any matrix A m n,thereexistssomer 2 ⇥ 2 1,...,n such that { } r A = sjujvj⇤, Xj=1 where s s s > 0 and u ,...,u m are orthonormal, and 1 ≥ 2 ≥···≥ r 1 r 2 v ,...,v n are orthonormal. 1 r 2 Corollary 3.4. The rank of a matrix equals its number of nonzero singular values. The singular value decomposition also gives an immediate formula for the 2-norm of a matrix. Theorem 3.5. Let A m n have singular value decomposition (3.1). 2 ⇥ Then Ax A = max k k = s1. k k x=0 x 6 k k Proof. The proof follows from the construction of the SVD at the start of this chapter. Note that Ax 2 x A Ax A 2 = max k k = max ⇤ ⇤ , k k x=0 x 2 x=0 x x 6 k k 6 ⇤ and so A 2 is the maximum Rayleigh quotient of the Hermitian matrix k k A⇤A. By Theorem 2.2, this maximum value is the largest eigenvalue of Embree – draft – 1 April 2017 i i i i i “book” — 2017/4/1 — 12:47 — page 96 — #98 i i i 96 Chapter 3.
Details
-
File Typepdf
-
Upload Time-
-
Content LanguagesEnglish
-
Upload UserAnonymous/Not logged-in
-
File Pages22 Page
-
File Size-