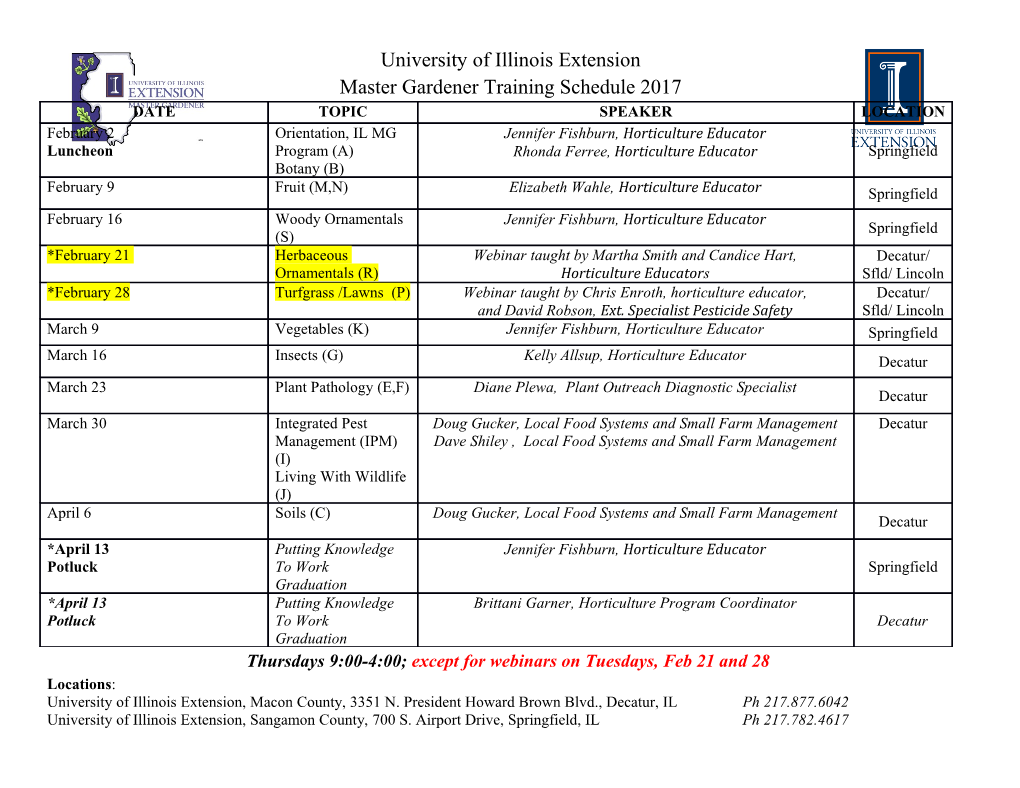
Lecture 9: Percolation and stochastic topology NSF/CBMS Conference Sayan Mukherjee Departments of Statistical Science, Computer Science, Mathematics Duke University www.stat.duke.edu/ sayan ⇠ May 31, 2016 Stochastic topology “I predict a new subject of statistical topology. Rather than count the number of holes, Betti numbers, etc., one will be more interested in the distribution of such objects on noncompact manifolds as one goes out to infinity,” Isadore Singer. Random graphs Erd˝os-R´enyi G G(n, p) is a draw of a graph G from the graph distribution. ⇠ Consider p(n)andn and ask questions about thresholds of !1 graph properties. Erd˝os-R´enyi random graph model G(n, p) is the probability space of graphs on vertex set [n]= 1,...n with the probability of each edge p independently. { } Consider p(n)andn and ask questions about thresholds of !1 graph properties. Erd˝os-R´enyi random graph model G(n, p) is the probability space of graphs on vertex set [n]= 1,...n with the probability of each edge p independently. { } G G(n, p) is a draw of a graph G from the graph distribution. ⇠ Erd˝os-R´enyi random graph model G(n, p) is the probability space of graphs on vertex set [n]= 1,...n with the probability of each edge p independently. { } G G(n, p) is a draw of a graph G from the graph distribution. ⇠ Consider p(n)andn and ask questions about thresholds of !1 graph properties. Matthew Kahle (Ohio State University) Random topology and geometry AMS Short Course Matthew Kahle (Ohio State University) Random topology and geometry AMS Short Course Matthew Kahle (Ohio State University) Random topology and geometry AMS Short Course Matthew Kahle (Ohio State University) Random topology and geometry AMS Short Course Matthew Kahle (Ohio State University) Random topology and geometry AMS Short Course Matthew Kahle (Ohio State University) Random topology and geometry AMS Short Course Matthew Kahle (Ohio State University) Random topology and geometry AMS Short Course Matthew Kahle (Ohio State University) Random topology and geometry AMS Short Course Matthew Kahle (Ohio State University) Random topology and geometry AMS Short Course Matthew Kahle (Ohio State University) Random topology and geometry AMS Short Course Matthew Kahle (Ohio State University) Random topology and geometry AMS Short Course Matthew Kahle (Ohio State University) Random topology and geometry AMS Short Course Matthew Kahle (Ohio State University) Random topology and geometry AMS Short Course Matthew Kahle (Ohio State University) Random topology and geometry AMS Short Course Matthew Kahle (Ohio State University) Random topology and geometry AMS Short Course Matthew Kahle (Ohio State University) Random topology and geometry AMS Short Course Matthew Kahle (Ohio State University) Random topology and geometry AMS Short Course Matthew Kahle (Ohio State University) Random topology and geometry AMS Short Course Matthew Kahle (Ohio State University) Random topology and geometry AMS Short Course Matthew Kahle (Ohio State University) Random topology and geometry AMS Short Course Matthew Kahle (Ohio State University) Random topology and geometry AMS Short Course Theorem (Erd˝os-R´enyi) log n+c Let c IR be fixed and G G(n, p).Ifp = n , then β0(G) is 2 ⇠ c asymptotically Poisson distributed with mean e− and e c lim IP [G is connected ]=e− − . n !1 Erd˝os-R´enyi theorem Theorem (Erd˝os-R´enyi) Let ✏ > 0 be fixed and G G(n, p).Then ⇠ 1:p (1 + ✏) log n/n IP [G is connected ] ≥ ! 0:p (1 + ✏) log n/n. ⇢ Erd˝os-R´enyi theorem Theorem (Erd˝os-R´enyi) Let ✏ > 0 be fixed and G G(n, p).Then ⇠ 1:p (1 + ✏) log n/n IP [G is connected ] ≥ ! 0:p (1 + ✏) log n/n. ⇢ Theorem (Erd˝os-R´enyi) log n+c Let c IR be fixed and G G(n, p).Ifp = n , then β0(G) is 2 ⇠ c asymptotically Poisson distributed with mean e− and e c lim IP [G is connected ]=e− − . n !1 Random simplicial complexes Y Y (n, p) is a draw of a random 2-simplicial complex Y from ⇠ 2 the distribution Y2(n, p) Lineal Meshulam 2-face model Y2(n, p) is the probability space of 2-dimensional simplicial [n] complexes with vertex set [n] and edge set 2 and every 2-dimensional face is included with probability p,independently. Lineal Meshulam 2-face model Y2(n, p) is the probability space of 2-dimensional simplicial [n] complexes with vertex set [n] and edge set 2 and every 2-dimensional face is included with probability p,independently. Y Y (n, p) is a draw of a random 2-simplicial complex Y from ⇠ 2 the distribution Y2(n, p) Matthew Kahle (Ohio State University) Random topology and geometry AMS Short Course Matthew Kahle (Ohio State University) Random topology and geometry AMS Short Course Matthew Kahle (Ohio State University) Random topology and geometry AMS Short Course Matthew Kahle (Ohio State University) Random topology and geometry AMS Short Course Matthew Kahle (Ohio State University) Random topology and geometry AMS Short Course Matthew Kahle (Ohio State University) Random topology and geometry AMS Short Course Matthew Kahle (Ohio State University) Random topology and geometry AMS Short Course Matthew Kahle (Ohio State University) Random topology and geometry AMS Short Course Matthew Kahle (Ohio State University) Random topology and geometry AMS Short Course Matthew Kahle (Ohio State University) Random topology and geometry AMS Short Course Y Y (n, p) is a draw of a random d-simplicial complex Y from ⇠ d the distribution Yd (n, p) Lineal Meshulam model Yd (n, p) is the probability space of d-dimensional simplicial complexes over all simplicial complexes on n vertices with complete d 1 skeleton and every d-dimensional face is included with − probability p,independently. Lineal Meshulam model Yd (n, p) is the probability space of d-dimensional simplicial complexes over all simplicial complexes on n vertices with complete d 1 skeleton and every d-dimensional face is included with − probability p,independently. Y Y (n, p) is a draw of a random d-simplicial complex Y from ⇠ d the distribution Yd (n, p) Theorem (Meshulam, Wallach) Let d 2, ` 2,and✏ > 0 be fixed and Y Y (n, p).Then ≥ ≥ ⇠ d 1:p (d + ✏) log n/n IP [Hd 1(Y , Z/`) = 0] ≥ − ! 0:p (d ✏) log n/n. ⇢ − Percolation on complexes Theorem (Linial,Meshulam) Let ✏ > 0 be fixed and Y Y (n, p).Then ⇠ 2 1:p (2 + ✏) log n/n IP [H1(Y , Z/2) = 0] ≥ ! 0:p (2 ✏) log n/n. ⇢ − Percolation on complexes Theorem (Linial,Meshulam) Let ✏ > 0 be fixed and Y Y (n, p).Then ⇠ 2 1:p (2 + ✏) log n/n IP [H1(Y , Z/2) = 0] ≥ ! 0:p (2 ✏) log n/n. ⇢ − Theorem (Meshulam, Wallach) Let d 2, ` 2,and✏ > 0 be fixed and Y Y (n, p).Then ≥ ≥ ⇠ d 1:p (d + ✏) log n/n IP [Hd 1(Y , Z/`) = 0] ≥ − ! 0:p (d ✏) log n/n. ⇢ − Simple connectivity Theorem (Babson, Ho↵man, Kahle) Let ✏ > 0 be fixed and Y Y (n, p).Then ⇠ 2 n✏ 1:p pn IP [⇡1(Y ) = 0] ≥ ✏ ! 0:p n− ( pn Theorem (Wagner) There exist constants c1, c2 > 0 such that for Y Yd (n, p) ⇠ 2d I if p < c1/n then with high probability Y is embeddable IR , I if p > c2/n then with high probability Y is not embeddable IR 2d . Embedding Every d-dimensional simplicial complex is embeddable in IR 2d+1 but not necessarily in IR 2d . Embedding Every d-dimensional simplicial complex is embeddable in IR 2d+1 but not necessarily in IR 2d . Theorem (Wagner) There exist constants c1, c2 > 0 such that for Y Yd (n, p) ⇠ 2d I if p < c1/n then with high probability Y is embeddable IR , I if p > c2/n then with high probability Y is not embeddable IR 2d . X X (n, p) is a draw of a random d-clique complex X from the ⇠ d distribution Xd (n, p). Random clique complex Xd (n, p) is the probability space of d-dimensional random clique complexes. Given a random graph G G(n, p), the clique ⇠ complex X is generated by including as a faces of the clique complex X (G) complete subgraphs of G. Random clique complex Xd (n, p) is the probability space of d-dimensional random clique complexes. Given a random graph G G(n, p), the clique ⇠ complex X is generated by including as a faces of the clique complex X (G) complete subgraphs of G. X X (n, p) is a draw of a random d-clique complex X from the ⇠ d distribution Xd (n, p). Random clique complex Homology vanishing results Theorem (Kahle) Fix k 1 and X X (n, p) and !(1) a function that tends to ≥ ⇠ d infinity arbitrarily slowly. Then with high probability Hk (X , IR )=0 if 1/(k+1) (k/2 + 1) log n + k log log n + !(1) p 2 ≥ n ! and H (X , IR ) =0if k 6 1/(k+1) (k/2 + 1) log n + k log log n !(1) p 1/nk , 2 − . 2 " n ! # Random flag complex homology # edges Kahle and Nanda: E[χ(X)] in blue for X(25,p)with β1 in green, β2 in red, β3 in cyan, and β5 in purple. Percolation and manifolds Stochastic topology Robert Adler The empirical object is ( , r)= B (p). U P r(n) p [2P Problem statement iid Given points = X , ..., X ⇢ where the support of ⇢ is a P { i n} ⇠ manifold . M Problem statement iid Given points = X , ..., X ⇢ where the support of ⇢ is a P { i n} ⇠ manifold .
Details
-
File Typepdf
-
Upload Time-
-
Content LanguagesEnglish
-
Upload UserAnonymous/Not logged-in
-
File Pages112 Page
-
File Size-