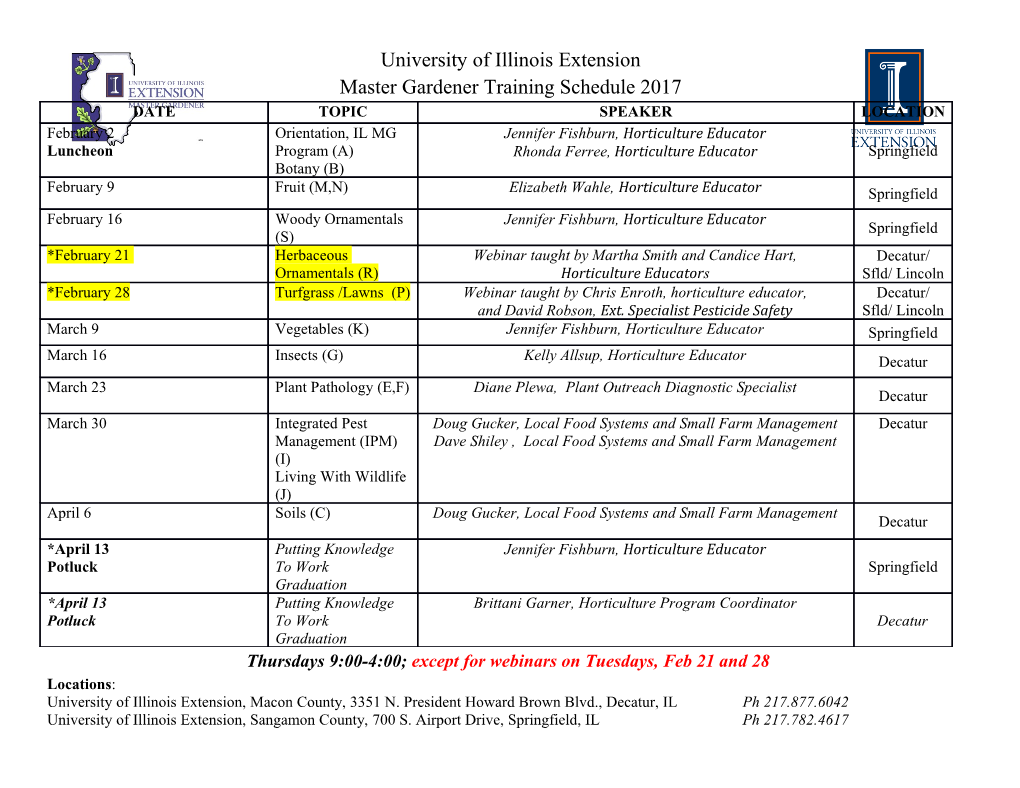
Curriculum Vitae – Wouter Duivesteijn Date of birth December 09, 1984 Place of birth Rotterdam, the Netherlands Nationality Dutch Email address [email protected] Website http://wwwis.win.tue.nl/~wouter/ Academic career September 2016 Assistant Professor Data Mining at Technische Universiteit Eindhoven (the Nether- - now lands). October 2015 Postdoctoraal Bursaal at Universiteit Gent (Belgium), working on the FORSIED project: - August 2016 FORmalising Subjective Interestingness in Exploratory Data mining. August 2015 Honorary Research Associate at University of Bristol (UK), working on the FORSIED - September 2015 project: FORmalising Subjective Interestingness in Exploratory Data mining. August 2013 Wissenschaftlicher Mitarbeiter at Technische Universität Dortmund (Germany), in - July 2015 Sonderforschungsbereich 876: Verfügbarkeit von Information durch Analyse unter Ressourcenbeschränkung (Collaborative Research Center SFB 876: Providing Informa- tion by Resource-Constrained Data Analysis). July 2009 PhD candidate in the Algorithms group at Leiden Institute of Advanced Computer - July 2013 Science, Universiteit Leiden (the Netherlands), under the supervision of Joost N. Kok and Arno Knobbe (graduation date: September 17, 2013). Education 2019 University Teaching Qualification (UTQ), Technische Universiteit Eindhoven. 2009-2013 PhD in Computer Science, Universiteit Leiden (graduated on September 17, 2013). 2008-2009 MSc in History and Philosophy of Science, Universiteit Utrecht (aborted for PhD position). 2005-2008 MSc in Applied Computing Science, Universiteit Utrecht (graduated on October 15, 2008). 2005-2007 MSc in Mathematical Sciences, Universiteit Utrecht (graduated on October 30, 2007). 2002-2005 BSc in Mathematics, Universiteit Utrecht (graduated on July 7, 2005). 2002-2005 BSc in Computer Science, Universiteit Utrecht (graduated on July 7, 2005). 1996-2002 Gymnasium, C.S.G. Blaise Pascal, Spijkenisse. Awards and funding C.J. Kokprijs 2013: the award for the best dissertation of the year in Leiden University’s Faculty of Science. The award has been bestowed annually since 1971; I am the only computer scientist to ever win it. Co-applicant in EDIC: Exceptional and Deep Intelligent Coach, a project funded in the NWO COMMIT2DATA - DATA2PERSON scheme, for a total amount of EUR 1 253 500. KDD 2012 student travel award: USD 1 000 plus free registration (worth USD 380). ICDM 2011 student travel award: CAD 500.ICDM 2010 student travel award: USD 500. 1 Research 1.1 Publications See also Google Scholar Citations: https://scholar.google.nl/citations?user=LmsQAtAAAAAJ. 1.1.1 Journal publications 11. W. Duivesteijn, S. Hess, X. Du: How to Cheat the Page Limit. In WIREs Data Mining and Knowledge Discovery, available online. Impact factor: 2:541 (2018). 10. X. Du, Y. Pei, W. Duivesteijn, M. Pechenizkiy: Exceptional Spatio-Temporal Behavior Mining through Bayesian Non-Parametric Modeling. In Data Mining and Knowledge Discovery, avail- able online. Impact factor: 2:879 (2018). 9. C. Rebelo de Sá, W. Duivesteijn, P. Azevedo, A.M. Jorge, C. Soares, A. Knobbe: Discovering a Taste for the Unusual — Exceptional Models for Preference Mining. In Machine Learning 107 (11), pp. 1775–1807, 2018. Impact factor: 2:809. 8. L. Downar, W. Duivesteijn: Exceptionally Monotone Models — the Rank Correlation Model Class for Exceptional Model Mining. In: Knowledge and Information Systems 51 (2), pp. 369–394, 2017. Impact factor: 2:247. 7. C. Pölitz, W. Duivesteijn, K. Morik: Interpretable Domain Adaptation via Optimization over the Stiefel Manifold. In: Machine Learning 104 (2–3), pp. 315–336, 2016. Impact factor: 1:848. 6. W. Duivesteijn: Correction to Jin-Ting Zhang’s “Approximate and Asymptotic Distributions of Chi-Squared-Type Mixtures with Applications”. In: Journal of the American Statistical Asso- ciation 111 (515), pp. 1370–1371, 2016. Impact factor: 2:016. 5. W. Duivesteijn, A.J. Feelders, A. Knobbe: Exceptional Model Mining — Supervised Descriptive Local Pattern Mining with Complex Target Concepts. In: Data Mining and Knowledge Discov- ery 30 (1), pp. 47–98, 2016. Impact factor: 3:160. 4. R.M. Konijn, W. Duivesteijn, M. Meeng, A. Knobbe: Cost-based Quality Measures in Subgroup Discovery. In: Journal of Intelligent Information Systems 45 (3), pp. 337–355, 2015. Impact factor: 1:000. 3. P. Lohuis, S. Faraj-Hakim, W. Duivesteijn, A. Knobbe, A.-J. Tasman: Benefits of a Short, Practical Questionnaire to Measure Subjective Perception of Nasal Appearance after Aesthetic Rhinoplasty. In: Plastic and Reconstructive Surgery 132 (6), pp. 913e–923e, 2013. Impact factor: 3:328. 2. P.J.F.M. Lohuis, S. Hakim, A. Knobbe, W. Duivesteijn, G.M. Bran: Split hump technique for reduction of the overprojected nasal dorsum – a statistical analysis on subjective body image in relation to nasal appearance and nasal patency in 97 aesthetic rhinoplasty patients. In: Archives of Facial Plastic Surgery, 2012, 14 (5), pp. 346–353. Impact factor: 1:463. 1. S. Hakim, A. Knobbe, W. Duivesteijn, P.J.F.M. Lohuis: Results of a screening questionnaire measuring physical perception of patients undergoing esthetic rhinoplasty: a statistical analysis. In: Nederlands Tijdschrift voor Keel-Neus-Oorheelkunde (Dutch Journal for Otorhinolaryngology), 2010 (2), p. 100. Impact factor: 0. 1.1.2 Conference publications 22. Y. Soons, R. Dijkman, M. Jilderda, W. Duivesteijn: Predicting Remaining Useful Life with Similarity-based Priors. In: Proceedings of the 18th International Symposium on Intelligent Data Analysis (IDA 2020), pp. 483–495. Acceptance rate: 0:3947 (45 out of 114). 21. X. Du, Y. Pei, W. Duivesteijn, M. Pechenizkiy: Fairness in Network Representation by Latent Structural Heterogeneity in Observational Data. In Proceedings of the 34th AAAI Conference on Artificial Intelligence (AAAI 2020), to appear. Acceptance rate: 0:2056 (1591 out of 7737). 20. A. Belfodil, W. Duivesteijn, M. Plantevit, S. Cazalens, P. Lamarre: DEvIANT: Discovering signif- icant exceptional (dis-)agreement within groups. In: Proceedings of the European Conference on Machine Learning and Principles and Practice of Knowledge Discovery in Databases (ECML PKDD 2019), to appear. Acceptance rate: 0:1771 (130 out of 734). 19. S. Hess, W. Duivesteijn: k is the Magic Number — Inferring the Number of Clusters Through Nonparametric Concentration Inequalities. In: Proceedings of the European Conference on Ma- chine Learning and Principles and Practice of Knowledge Discovery in Databases (ECML PKDD 2019), to appear. Acceptance rate: 0:1771 (130 out of 734). 18. S. Hess, W. Duivesteijn, P. Honysz, K. Morik: The SpectACl of Nonconvex Clustering: A Spec- tral Approach to Density-Based Clustering. In: Proceedings of the 33rd AAAI Conference on Artificial Intelligence (AAAI 2019), pp. 3788–3795. Acceptance rate: 0:1621 (1150 out of 7095). 17. X. Du, W. Duivesteijn, M. Klabbers, M. Pechenizkiy: ELBA: Exceptional Learning Behavior Analysis. In: Proceedings of the 11th International Conference on Educational Data Mining (EDM 2018), pp. 312–318. Acceptance rate: 0:4207 (61 out of 145). 16. J. Lijffijt, B. Kang, W. Duivesteijn, K. Puolamäki, E. Oikarinen, T. De Bie: Subjectively Interesting Subgroup Discovery on Real-valued Targets. In: Proceedings of the 34th IEEE International Conference on Data Engineering (ICDE 2018), pp. 1352-1355. Acceptance rate: 0:2311 (98 out of 424). A substantially longer version appeared on arXiv: https://arxiv.org/abs/1710.04521 15. W. Duivesteijn, T. Farzami, T. Putman, E. Peer, H.J.P. Weerts, J.N. Adegeest, G. Foks, M. Pech- enizkiy: Have It Both Ways — from A/B Testing to A&B Testing with Exceptional Model Mining. In: Proceedings of the European Conference on Machine Learning and Principles and Prac- tice of Knowledge Discovery in Databases (ECML PKDD 2017), part III, pp. 114–126. Acceptance rate: 0:2903 (27 out of 93). 14. C. Rebelo de Sá, W. Duivesteijn, C. Soares, A. Knobbe: Exceptional Preferences Mining. In: Proceedings of the 19th International Conference on Discovery Science (DS 2016), pp. 3–18. Acceptance rate: 0:5000 (30 out of 60). 13. L. Downar, W. Duivesteijn: Exceptionally Monotone Models — the Rank Correlation Model Class for Exceptional Model Mining. In: Proceedings of the 15th IEEE International Conference on Data Mining (ICDM 2015), pp. 111–120, 2015. Acceptance rate: 0:0843 (68 out of 807). Including short papers: 0:1821 (147 out of 807). Distilled from Lennart’s Bachelor thesis, available at: http://sfb876.tu-dortmund.de/PublicPublicationFiles/downar_2014a.pdf 12. W. Duivesteijn, J. Thaele: Understanding Where Your Classifier Does (Not) Work. In: Proceedings of the European Conference on Machine Learning and Principles and Practice of Knowledge Discovery in Databases (ECML PKDD 2015) (III), pp. 250–253, 2015. Acceptance rate: 0:4828 (14 out of 29). 11. W. Duivesteijn, J. Thaele: Understanding Where Your Classifier Does (Not) Work — the SCaPE Model Class for EMM. In: Proceedings of the 14th IEEE International Conference on Data Mining (ICDM 2014), pp. 809–814, 2014. Acceptance rate: 0:1953 (142 out of 727). A substantially longer version appeared as Technical Report of the SFB 876 at the TU Dortmund: http://sfb876.tu-dortmund.de/PublicPublicationFiles/duivesteijn_thaele_2014a.pdf 10. J. Witteveen, W. Duivesteijn, A. Knobbe, P. Grünwald: RealKRIMP – Finding Hyperintervals that Compress with MDL for Real-Valued Data. In: Proceedings of the 13th International Symposium on Intelligent Data Analysis (IDA 2014), pp. 368–379, 2014. Acceptance rate: 0:4800 (36 out of 75). Distilled from Jouke’s
Details
-
File Typepdf
-
Upload Time-
-
Content LanguagesEnglish
-
Upload UserAnonymous/Not logged-in
-
File Pages12 Page
-
File Size-