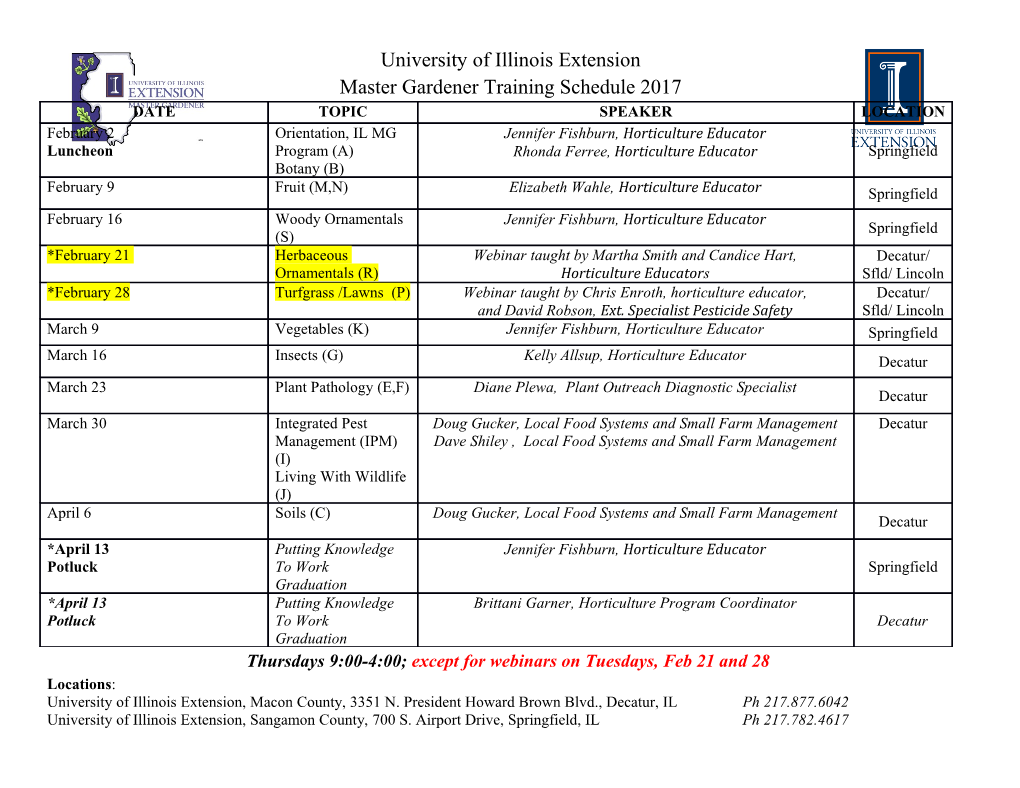
Usage of WordNet in Natural Language Generation Hongyan Jing Department of Computer Science Columbia University New York, NY 10027, USA [email protected] Abstract 2 Why a valuable resource for generation? WordNet has rarely been applied to natural lan- WordNet is a potentially valuable resource for guage generation, despite of its wide applica- generation for four reasons. First, Synonym tion in other fields. In this paper, we address sets in WordNet (synsets) can possibly provide three issues in the usage of WordNet in gener- large amount of lexical paraphrases. One ma- ation: adapting a general lexicon like WordNet jor shortcoming of current generation systems is to a specific application domain, how the infor- its poor expressive capability. Usually none or mation in WordNet can be used in generation, very limited paraphrases are provided by a gen- and augmenting WordNet with other types of eration system due to the cost of hand-coding in knowledge that are helpful for generation. We the lexicon. Synsets, however, provide the pos- propose a three step procedure to tailor Word- sibility to generate lexical paraphrases without Net to a specific domain, and carried out ex- tedious hand-coding in individual systems. For periments on a basketball corpus (1,015 game example, for the output sentence \Jordan hit a reports, 1.7MB). jumper", we can generate the paraphrase \Jor- dan hit a jump shot" simply by replacing the word jumper in the sentence with its synonym 1 Introduction jump shot listed in WordNet synset. Whereas, such replacements are not always appropriate. WordNet (Miller et al., 1990) has been success- For example, tally and rack up are listed as syn- fully applied in many human language related onyms of the word score, although the sentence applications, such as word sense disambigua- like \Jordan scored 22 points" are common in tion, information retrieval, and text categoriza- newspaper sport reports, sentences like \Jor- tion; yet generation is among the fields in which dan tallied 22 points" or \Jordan racked up 22 the application of WordNet has rarely been ex- points" seldomly occur. To successfully apply plored. We demonstrate in this paper that, as a WordNet for paraphrasing, we need to develop rich semantic net, WordNet is indeed a valuable techniques which can correctly identify inter- resource for generation. We propose a corpus changeability of synonyms in a certain context. based technique to adapt WordNet to a specific Secondly, as a semantic net linked by lexi- domain and present experiments in the basket- cal relations, WordNet can be used for lexical- ball domain. We also discuss possible ways to ization in generation. Lexicalization maps the use WordNet knowledge in the generation task semantic concepts to be conveyed to appropri- and to augment WordNet with other types of ate words. Usually it is achieved by step-wise knowledge. refinements based on syntactic, semantic, and In Section 2, we answer the question why pragmatic constraints while traversing a seman- WordNet is useful for generation. In Section tic net (Danlos, 1987). Currently most genera- 3, we discuss problems to be solved to success- tion systems acquire their semantic net for lexi- fully apply WordNet to generation. In Section calization by building their own, while WordNet 4, we present techniques to solve the problems. provides the possibility to acquire such knowl- Finally, we present future work and conclude. edge automatically from an existing resource. Next, WordNet ontology can be used for Once WordNet is tailored to the domain, the building domain ontology. Most current genera- main problem is how to use its knowledge in the tion systems manually build their domain ontol- generation process. As we mentioned in section ogy from scratch. The process is time and labor 2, WordNet can potentially benefit generation intensive, and introduction of errors is likely. in three aspects: producing large amount of lex- WordNet ontology has a wide coverage, so can ical paraphrases, providing the semantic net for possibly be used as a basis for building domain lexicalization, and providing a basis for building ontology. The problem to be solved is how to domain ontology. A number of problems to be adapt it to a specific domain. solved at this stage, including: (a)while using Finally, WordNet is indexed by concepts synset for producing paraphrases, how to de- rather than merely by words makes it especially termine whether two synonyms are interchange- desirable for the generation task. Unlike lan- able in a particular context? (b)while WordNet guage interpretation, generation has as inputs can provide the semantic net for lexicalization, the semantic concepts to be conveyed and maps the constraints to choose a particular node dur- them to appropriate words. Thus an ideal gen- ing lexical choice still need to be established. eration lexicon should be indexed by semantic (c) How to use the WordNet ontology? concepts rather than words. Most available lin- The last problem is relevant to augmenting guistic resources are not suitable to use in gen- WordNet with other types of information. Al- eration directly due to their lack of mapping be- though WordNet is a rich lexical database, it tween concepts and words. WordNet is by far can not contain all types of information that the richest and largest database among all re- are needed for generation, for example, syntac- sources that are indexed by concepts. Other rel- tic information in WordNet is weak. It is then atively large and concept-based resources such worthwhile to investigate the possibility to com- as PENMAN ontology (Bateman et al., 1990) bine it with other resources. usually include only hyponymy relations com- In the following section, we address the above pared to the rich types of lexical relations pre- issues in order and present our experiment re- sented in WordNet. sults in the basketball domain. 3 Problems to be solved 4 Solutions Despite the above advantages, there are some 4.1 Adapting WordNet to a domain problems to be solved for the application of We propose a corpus based method to automat- WordNet in a generation system to be success- ically adapt a general resource like WordNet to ful. a domain. Most generation systems still use The first problem is how to adapt WordNet hand-coded lexicons and ontologies, however, to a particular domain. With 121,962 unique corpus based automatic techniques are in de- words, 99,642 synsets, and 173,941 senses of mand as natural language generation is used in words as of version 1.6, WordNet represents the more ambitious applications and large corpora largest publically available lexical resource to in various domains are becoming available. The date. The wide coverage on one hand is benefi- proposed method involves three steps of pro- cial, since as a general resource, wide coverage cessing. allows it to provide information for different ap- plications. On the other hand, this can also be Step 1: Prune unused words and quite problematic since it is very difficult for synsets an application to efficiently handle such a large We first prune words and synsets that are database. Therefore, the first step towards uti- listed in WordNet but not used in the domain. lizing WordNet in generation is to prune unre- This is accomplished by tagging the domain cor- lated information in the general database so as pus with part of speech information, then for to tailor it to the domain. On the other hand, each word in WordNet, if it appears in the do- domain specific knowledge that is not covered main corpus and its part of speech is the same by the general database needs to be added to as that in the corpus, the word is kept in the re- the database. sult, otherwise it is eliminated; for each synset in WordNet, if none of the words in the synset { A } appears in the domain corpus, the synset as a / \ { A } whole is deleted. The only exception is that if { B } ... ===> / \ a synset is the closest common ancestor of two / \ { D } ... synsets in the domain corpus, the synset is al- { C } { F } ways kept in the result. The reason to keep this / \ kind of synsets is to generalize the semantic cat- { D }{ E } egory of verb arguments, as we illustrate in step 2. The frequency of words in such synsets will before after be marked zero so that they will not be used in output. Figure 1 shows two example prun- (A) Synset A and D appear in the corpus, ing operations: (A) is a general case, and (B) while B, C, E, and F do not. is the case involving ancestor synset. In this step, words are not yet disambiguated, so all the senses of a word remain in the result; the prun- { A } ing of unlikely senses is achieved in step 2, when / \ { A } verb argument clusters are utilized. Words that { B } { C } ===> / \ are in the corpus but not covered by WordNet / \ { B }{ D } are also identified in this stage, and later at step { D }{ E} 3, we guess the meanings of these known words and place them into domain ontology. before after A total of 1,015 news reports on basketball games (1.7MB, Clarinet news, 1990-1991) were (B) Synset B and D appear in the corpus, collected. The frequency count reported totally A, C, and E do not. Note Synset A is not 1,414 unique nouns (proper names excluded) removed since it's the closest ancestor and 993 unique verbs in the corpus. Compared of B and D. to 94,473 nouns and 10,318 verbs in WordNet 1.6, only 1.5% of nouns and 9.6% of verbs are Figure 1: Examples for corpus based pruning used in the domain.
Details
-
File Typepdf
-
Upload Time-
-
Content LanguagesEnglish
-
Upload UserAnonymous/Not logged-in
-
File Pages7 Page
-
File Size-