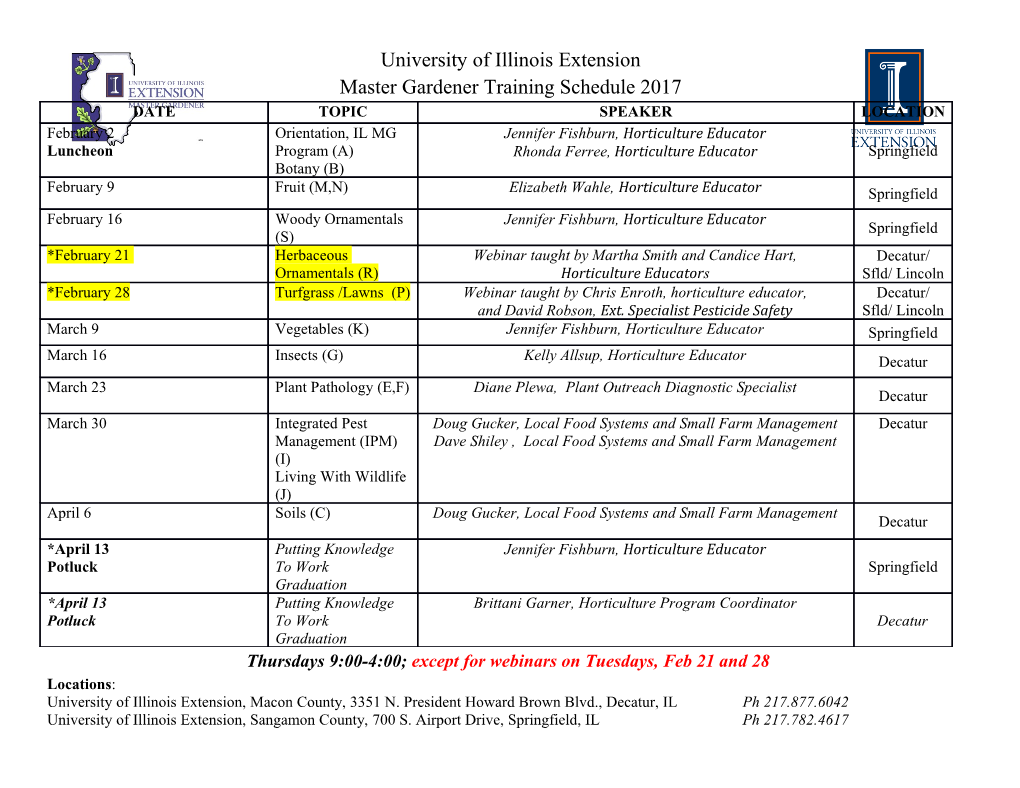
Learning the Countability of English Nouns from Corpus Data Timothy Baldwin Francis Bond CSLI NTT Communication Science Laboratories Stanford University Nippon Telegraph and Telephone Corporation Stanford, CA, 94305 Kyoto, Japan [email protected] [email protected] Abstract ence. Knowledge of countability preferences is im- portant both for the analysis and generation of En- This paper describes a method for learn- glish. In analysis, it helps to constrain the inter- ing the countability preferences of English pretations of parses. In generation, the countabil- nouns from raw text corpora. The method ity preference determines whether a noun can be- maps the corpus-attested lexico-syntactic come plural, and the range of possible determin- properties of each noun onto a feature ers. Knowledge of countability is particularly im- vector, and uses a suite of memory-based portant in machine translation, because the closest classifiers to predict membership in 4 translation equivalent may have different countabil- countability classes. We were able to as- ity from the source noun. Many languages, such sign countability to English nouns with a as Chinese and Japanese, do not mark countability, precision of 94.6%. which means that the choice of countability will be largely the responsibility of the generation compo- nent (Bond, 2001). In addition, knowledge of count- 1 Introduction ability obtained from examples of use is an impor- tant resource for dictionary construction. This paper is concerned with the task of knowledge- In this paper, we learn the countability prefer- rich lexical acquisition from unannotated corpora, ences of English nouns from unannotated corpora. focusing on the case of countability in English. We first annotate them automatically, and then train Knowledge-rich lexical acquisition takes unstruc- classifiers using a set of gold standard data, taken tured text and extracts out linguistically-precise cat- from COMLEX (Grishman et al., 1998) and the trans- egorisations of word and expression types. By fer dictionaries used by the machine translation sys- combining this with a grammar, we can build tem ALT-J/E (Ikehara et al., 1991). The classifiers broad-coverage deep-processing tools with a min- and their training are described in more detail in imum of human effort. This research is close Baldwin and Bond (2003). These are then run over in spirit to the work of Light (1996) on classi- the corpus to extract nouns as members of four fying the semantics of derivational affixes, and classes — countable: dog; uncountable: furniture; bi- Siegel and McKeown (2000) on learning verb as- partite: [pair of] scissors and plural only: clothes. pect. We first discuss countability in more detail (x 2). In English, nouns heading noun phrases are typ- Then we present the lexical resources used in our ex- ically either countable or uncountable (also called periment (x 3). Next, we describe the learning pro- count and mass). Countable nouns can be modi- cess (x 4). We then present our results and evalu- fied by denumerators, prototypically numbers, and ation (x 5). Finally, we discuss the theoretical and have a morphologically marked plural form: one practical implications (x 6). dog, two dogs. Uncountable nouns cannot be modi- fied by denumerators, but can be modified by unspe- 2 Background cific quantifiers such as much, and do not show any number distinction (prototypically being singular): Grammatical countability is motivated by the se- *one equipment, some equipment, *two equipments. mantic distinction between object and substance Many nouns can be used in countable or uncountable reference (also known as bounded/non-bounded or environments, with differences in interpretation. individuated/non-individuated). It is a subject of We call the lexical property that determines which contention among linguists as to how far grammat- uses a noun can have the noun’s countability prefer- ical countability is semantically motivated and how much it is arbitrary (Wierzbicka, 1988). ers will dispute the validity of the new usage. We The prevailing position in the natural language do not necessarily wish to learn such rare examples, processing community is effectively to treat count- and may not need to learn more common conver- ability as though it were arbitrary and encode it as sions either, as they can be handled by regular lexi- a lexical property of nouns. The study of countabil- cal rules (Copestake and Briscoe, 1995). The second ity is complicated by the fact that most nouns can problem is that some constructions affect the appar- have their countability changed: either converted by ent countability of their head: for example, nouns a lexical rule or embedded in another noun phrase. denoting a role, which are typically countable, can An example of conversion is the so-called universal appear without an article in some constructions (e.g. packager, a rule which takes an uncountable noun We elected him treasurer). The third is that different with an interpretation as a substance, and returns a senses of a word may have different countabilities: countable noun interpreted as a portion of the sub- interest “a sense of concern with and curiosity” is stance: I would like two beers. An example of em- normally countable, whereas interest “fixed charge bedding is the use of a classifier, e.g. uncountable for borrowing money” is uncountable. nouns can be embedded in countable noun phrases There have been at several earlier approaches as complements of classifiers: one piece of equip- to the automatic determination of countabil- ment. ity. Bond and Vatikiotis-Bateson (2002) determine Bond et al. (1994) suggested a division of count- a noun’s countability preferences from its seman- ability into five major types, based on Allan (1980)’s tic class, and show that semantics predicts (5-way) noun countability preferences (NCPs). Nouns which countability 78% of the time with their ontology. rarely undergo conversion are marked as either fully O’Hara et al. (2003) get better results (89.5%) using countable, uncountable or plural only. Fully countable the much larger Cyc ontology, although they only nouns have both singular and plural forms, and can- distinguish between countable and uncountable. not be used with determiners such as much, little, a Schwartz (2002) created an automatic countabil- little, less and overmuch. Uncountable nouns, such ity tagger (ACT) to learn noun countabilities from as furniture, have no plural form, and can be used the British National Corpus. ACT looks at deter- with much. Plural only nouns never head a singular miner co-occurrence in singular noun chunks, and noun phrase: goods, scissors. classifies the noun if and only if it occurs with a de- Nouns that are readily converted are marked as ei- terminer which can modify only countable or un- ther strongly countable (for countable nouns that can countable nouns. The method has a coverage of be converted to uncountable, such as cake) or weakly around 50%, and agrees with COMLEX for 68% of countable (for uncountable nouns that are readily the nouns marked countable and with the ALT-J/E convertible to countable, such as beer). lexicon for 88%. Agreement was worse for uncount- NLP systems must list countability for at least able nouns (6% and 44% respectively). some nouns, because full knowledge of the refer- ent of a noun phrase is not enough to predict count- 3 Resources ability. There is also a language-specific knowl- edge requirement. This can be shown most sim- Information about noun countability was obtained ply by comparing languages: different languages en- from two sources. One was COMLEX 3.0 (Grish- code the countability of the same referent in dif- man et al., 1998), which has around 22,000 noun ferent ways. There is nothing about the concept entries. Of these, 12,922 are marked as being count- denoted by lightning, e.g., that rules out *a light- able (COUNTABLE) and 4,976 as being uncountable ning being interpreted as a flash of lightning. In- (NCOLLECTIVE or :PLURAL *NONE*). The remainder deed, the German and French translation equivalents are unmarked for countability. are fully countable (ein Blitz and un eclair´ respec- The other was the common noun part of ALT- tively). Even within the same language, the same J/E’s Japanese-to-English semantic transfer dictio- referent can be encoded countably or uncountably: nary (Bond, 2001). It contains 71,833 linked clothes/clothing, things/stuff , jobs/work. Japanese-English pairs, each of which has a value Therefore, we must learn countability classes for the noun countability preference of the English from usage examples in corpora. There are several noun. Considering only unique English entries with impediments to this approach. The first is that words different countability and ignoring all other informa- are frequently converted to different countabilities, tion gave 56,245 entries. Nouns in the ALT-J/E dic- sometimes in such a way that other native speak- tionary are marked with one of the five major count- ability preference classes described in Section 2. In of nouns countability-classified in both lexicons, the addition to countability, default values for number mean was 93.8%. Almost half of the disagreements and classifier (e.g. blade for grass: blade of grass) came from words with two countabilities in ALT-J/E are also part of the lexicon. but only one in COMLEX. We classify words into four possible classes, with some words belonging to multiple classes. The first 4 Learning Countability class is countable: COMLEX’s COUNTABLE and ALT- J/E’s fully, strongly and weakly countable. The sec- The basic methodology employed in this research is ond class is uncountable: COMLEX’s NCOLLECTIVE or to identify lexical and/or constructional features as- :PLURAL *NONE* and ALT-J/E’s strongly and weakly sociated with the countability classes, and determine countable and uncountable.
Details
-
File Typepdf
-
Upload Time-
-
Content LanguagesEnglish
-
Upload UserAnonymous/Not logged-in
-
File Pages8 Page
-
File Size-