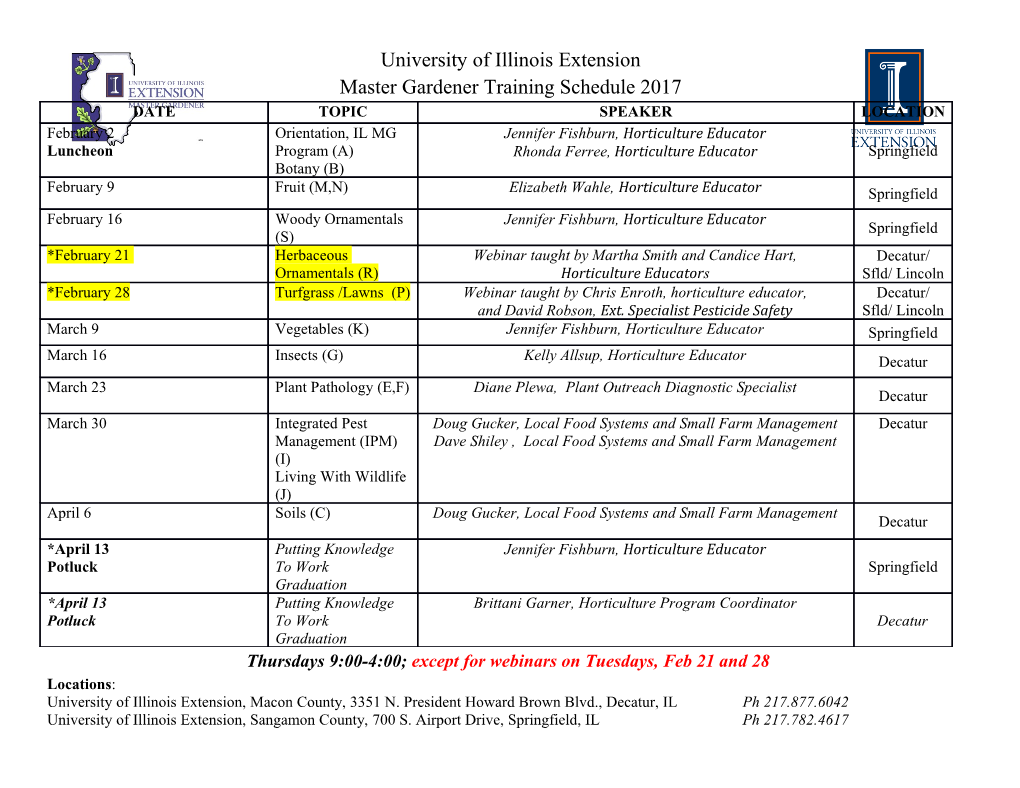
7. NETWORK FLOW I ‣ max-flow and min-cut problems ‣ Ford–Fulkerson algorithm ‣ max-flow min-cut theorem ‣ capacity-scaling algorithm ‣ shortest augmenting paths ‣ Dinitz’ algorithm ‣ simple unit-capacity networks Lecture slides by Kevin Wayne Copyright © 2005 Pearson-Addison Wesley http://www.cs.princeton.edu/~wayne/kleinberg-tardos Last updated on 1/14/20 2:18 PM 7. NETWORK FLOW I ‣ max-flow and min-cut problems ‣ Ford–Fulkerson algorithm ‣ max-flow min-cut theorem ‣ capacity-scaling algorithm ‣ shortest augmenting paths ‣ Dinitz’ algorithm ‣ simple unit-capacity networks SECTION 7.1 Flow network A flow network is a tuple G = (V, E, s, t, c). ・Digraph (V, E) with source s ∈ V and sink t ∈ V. Capacity c(e) ≥ 0 for each e ∈ E. ・ assume all nodes are reachable from s Intuition. Material flowing through a transportation network; material originates at source and is sent to sink. capacity 9 4 15 15 10 10 s 5 8 10 t 15 4 6 15 10 16 3 Minimum-cut problem Def. An st-cut (cut) is a partition (A, B) of the nodes with s ∈ A and t ∈ B. Def. Its capacity is the sum of the capacities of the edges from A to B. cap(A, B)= c(e) e A 10 s 5 t 15 capacity = 10 + 5 + 15 = 30 4 Minimum-cut problem Def. An st-cut (cut) is a partition (A, B) of the nodes with s ∈ A and t ∈ B. Def. Its capacity is the sum of the capacities of the edges from A to B. cap(A, B)= c(e) e A 10 s 8 t don’t include edges from B to A 16 capacity = 10 + 8 + 16 = 34 5 Minimum-cut problem Def. An st-cut (cut) is a partition (A, B) of the nodes with s ∈ A and t ∈ B. Def. Its capacity is the sum of the capacities of the edges from A to B. cap(A, B)= c(e) e A Min-cut problem. Find a cut of minimum capacity. 10 s 8 t 10 capacity = 10 + 8 + 10 = 28 6 Network flow: quiz 1 Which is the capacity of the given st-cut? A. 11 (20 + 25 − 8 − 11 − 9 − 6) B. 34 (8 + 11 + 9 + 6) C. 45 (20 + 25) D. 79 (20 + 25 + 8 + 11 + 9 + 6) capacity s 20 8 10 6 12 8 11 9 8 6 1 16 25 t 7 Maximum-flow problem Def. An st-flow (flow) f is a function that satisfies: For each e E : 0 f(e ) c(e ) [capacity] ・ ∈ ≤ ≤ For each v ∈ V – {s, t} : f(e)= f(e) [flow conservation] ・ e v e v flow capacity inflow at v = 5 + 5 + 0 = 10 5 / 9 outflow at v = 10 + 0 = 10 5 / 15 5 / 10 0 / 4 0 / 15 10 / 10 s 5 / 5 5 / 8 v 10 / 10 t 10 / 15 0 / 4 0 / 6 0 / 15 10 / 10 10 / 16 8 Maximum-flow problem Def. An st-flow (flow) f is a function that satisfies: For each e E : 0 f(e ) c(e ) [capacity] ・ ∈ ≤ ≤ For each v ∈ V – {s, t} : f(e)= f(e) [flow conservation] ・ e v e v Def. The value of a flow f is: val(f)= f(e) f(e) − e s e s 5 / 9 5 / 15 5 / 10 0 / 4 0 / 15 10 / 10 s 5 / 5 5 / 8 10 / 10 t 10 / 15 0 / 4 0 / 6 0 / 15 10 / 10 value = 5 + 10 + 10 = 25 10 / 16 9 Maximum-flow problem Def. An st-flow (flow) f is a function that satisfies: For each e E : 0 f(e ) c(e ) [capacity] ・ ∈ ≤ ≤ For each v ∈ V – {s, t} : f(e)= f(e) [flow conservation] ・ e v e v Def. The value of a flow f is: val(f)= f(e) f(e) − e s e s Max-flow problem. Find a flow of maximum value. 8 / 9 2 / 15 8 / 10 0 / 4 0 / 15 10 / 10 s 5 / 5 8 / 8 10 / 10 t 13 / 15 0 / 4 3 / 6 0 / 15 10 / 10 value = 10 + 5 + 13 = 28 13 / 16 10 7. NETWORK FLOW I ‣ max-flow and min-cut problems ‣ Ford–Fulkerson algorithm ‣ max-flow min-cut theorem ‣ capacity-scaling algorithm ‣ shortest augmenting paths ‣ Dinitz’ algorithm ‣ simple unit-capacity networks SECTION 7.1 Toward a max-flow algorithm Greedy algorithm. ・Start with f (e) = 0 for each edge e ∈ E. ・Find an s↝t path P where each edge has f (e) < c(e). ・Augment flow along path P. ・Repeat until you get stuck. flow capacity flow network G and flow f 0 / 4 0 / 10 0 / 2 0 / 8 0 / 6 0 / 10 value of flow s 0 / 10 0 / 9 0 / 10 t 0 12 Toward a max-flow algorithm Greedy algorithm. ・Start with f (e) = 0 for each edge e ∈ E. ・Find an s↝t path P where each edge has f (e) < c(e). ・Augment flow along path P. ・Repeat until you get stuck. flow network G and flow f 0 / 4 0 / 10 0 / 2 0 / 8 0 / 6 0 / 10 s 0 / 10 0 / 9 0 / 10 t 0 13 Toward a max-flow algorithm Greedy algorithm. ・Start with f (e) = 0 for each edge e ∈ E. ・Find an s↝t path P where each edge has f (e) < c(e). ・Augment flow along path P. ・Repeat until you get stuck. flow network G and flow f 0 / 4 8 0 / 10 —0 / 8 8 0 / 2 0 / 6 —0 / 10 8 s 0 / 10 0 / 9 —0 / 10 t 0 + 8 = 8 14 Toward a max-flow algorithm Greedy algorithm. ・Start with f (e) = 0 for each edge e ∈ E. ・Find an s↝t path P where each edge has f (e) < c(e). ・Augment flow along path P. ・Repeat until you get stuck. flow network G and flow f 0 / 4 0 / 10 2 —0 / 2 8 / 8 0 / 6 10 —8 / 10 2 10 s 0 / 10 —0 / 9 —8 / 10 t 8 + 2 = 10 15 Toward a max-flow algorithm Greedy algorithm. ・Start with f (e) = 0 for each edge e ∈ E. ・Find an s↝t path P where each edge has f (e) < c(e). ・Augment flow along path P. ・Repeat until you get stuck. flow network G and flow f 0 / 4 6 —0 / 10 2 / 2 8 / 8 6 —0 / 6 10 / 10 6 8 s —0 / 10 —2 / 9 10 / 10 t 10 + 6 = 16 16 Toward a max-flow algorithm Greedy algorithm. ・Start with f (e) = 0 for each edge e ∈ E. ・Find an s↝t path P where each edge has f (e) < c(e). ・Augment flow along path P. ・Repeat until you get stuck. ending flow value = 16 flow network G and flow f 0 / 4 6 / 10 2 / 2 8 / 8 6 / 6 10 / 10 s 6 / 10 8 / 9 10 / 10 t 16 17 Toward a max-flow algorithm Greedy algorithm. ・Start with f (e) = 0 for each edge e ∈ E. ・Find an s↝t path P where each edge has f (e) < c(e). ・Augment flow along path P. ・Repeat until you get stuck. but max-flow value = 19 flow network G and flow f 3 / 4 9 / 10 0 / 2 7 / 8 6 / 6 10 / 10 s 9 / 10 9 / 9 10 / 10 t 19 18 Why the greedy algorithm fails Q. Why does the greedy algorithm fail? A. Once greedy algorithm increases flow on an edge, it never decreases it. Ex. Consider flow network G . ・The unique max flow f * has f *(v, w) = 0. ・Greedy algorithm could choose s→v→w→t as first path. flow network G v 2 t 2 1 2 s 2 w Bottom line. Need some mechanism to “undo” a bad decision. 19 Residual network Original edge. e = (u, v) E. ∈ original flow network G Flow f (e). ・ u 6 / 17 v ・Capacity c(e). flow capacity Reverse edge. ereverse = (v, u). ・“Undo” flow sent. residual network Gf residual Residual capacity. capacity u 11 v c(e) f(e) e E cf (e)= − ∈ 6 f(e) e E ∈ reverse edge edges with positive residual capacity Residual network. Gf = (V, Ef , s, t, cf ). where flow on a reverse edge negates flow on reverse ・Ef = {e : f (e) < c(e)} ∪ {e : f (e) > 0}. corresponding forward edge Key property: is a flow in iff is a flow in . ・ f ʹ Gf f + f ʹ G 20 Augmenting path Def. An augmenting path is a simple s↝t path in the residual network Gf . Def. The bottleneck capacity of an augmenting path P is the minimum residual capacity of any edge in P. Key property. Let f be a flow and let P be an augmenting path in Gf . Then, after calling f ʹ ← AUGMENT( f, c, P), the resulting f ʹ is a flow and val( f ʹ) = val( f ) + bottleneck(Gf, P). AUGMENT( f, c, P) ________________________________________________________________________________________________________________________________________________________________________________________________________________________________________________________________________________________________________________________________________________________________________________________________________________________________________________________________________________________________________________________________________________________________________________________________________________________________________________________________________________________________________________________________________________________________________________________________________________ δ ← bottleneck capacity of augmenting path P. FOREACH edge e ∈ P : IF (e ∈ E) f (e) ← f (e) + δ. ELSE f (ereverse) ← f (ereverse) – δ. RETURN f. ________________________________________________________________________________________________________________________________________________________________________________________________________________________________________________________________________________________________________________________________________________________________________________________________________________________________________________________________________________________________________________________________________________________________________________________________________________________________________________________________________________________________________________________________________________________________________________________________________________ 21 Network flow: quiz 2 Which is the augmenting path of highest bottleneck capacity? A. A → F → G → H B. A → B → C → D → H C. A → F → B → G → H D. A → F → B → G → C → D → H residual capacity 5 A 9 B 8 C 6 D source 5 7 8 7 4 5 6 8 5 E 5 F 2 G 3 H 5 target 22 Ford–Fulkerson algorithm Ford–Fulkerson augmenting path algorithm.
Details
-
File Typepdf
-
Upload Time-
-
Content LanguagesEnglish
-
Upload UserAnonymous/Not logged-in
-
File Pages96 Page
-
File Size-