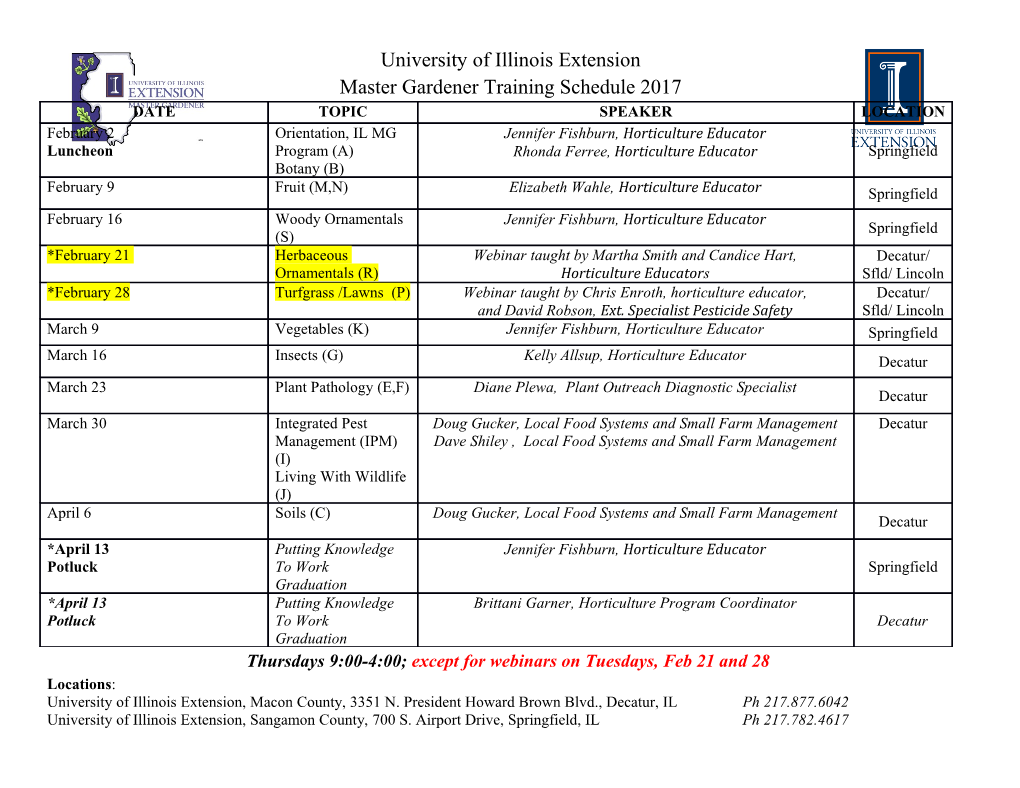
Risk & Business Analytics teach-in November 8, 2018 London 1 | 2 Disclaimer regarding forward-looking statements This presentation contains forward-looking statements within the meaning of Section 27A of the US Securities Act of 1933, as amended, and Section 21E of the US Securities Exchange Act of 1934, as amended. These statements are subject to a number of risks and uncertainties that could cause actual results or outcomes to differ materially from those currently being anticipated. The terms “outlook”, “estimate”, “project”, “plan”, “intend”, “expect”, “should be”, “will be”, “believe”, “trends” and similar expressions identify forward-looking statements. Factors which may cause future outcomes to differ from those foreseen in forward-looking statements include, but are not limited to: current and future economic, political and market forces; changes in law and legal interpretations affecting the RELX Group intellectual property rights; regulatory and other changes regarding the collection, transfer or use of third party content and data; demand for the RELX Group products and services; competitive factors in the industries in which the RELX Group operates; compromises of our data security systems and interruptions in our information technology systems; legislative, fiscal, tax and regulatory developments and political risks; exchange rate fluctuations; and other risks referenced from time to time in the filings of RELX PLC and, historically, RELX N.V. with the US Securities and Exchange Commission. Definitions Underlying figures are additional performance measures used by management, and are calculated at constant currencies, excluding the results of all acquisitions and disposals made in both the year and prior year, assets held for sale, exhibition cycling, and timing effects. 2017 revenue and adjusted operating profit restated for the adoption of IFRS 9, 15 & 16. 2 Introduction to Risk & Business Analytics Mark Kelsey Chief Executive Officer, Risk & Business Analytics 3 Agenda Introduction to Risk & Business Analytics Mark Kelsey CEO, Risk & Business Analytics Approach to technology, AI and ML Vijay Raghavan across RELX CTO, Risk & Business Analytics Business Services Rick Trainor CEO, Business Services Ian Spanswick Vice President, ThreatMetrix Data Services - Accuity Hugh Jones CEO, Data Services Concluding remarks Mark Kelsey CEO, Risk & Business Analytics Q&A 4 | 5 Risk & Business Analytics – position within RELX Group 2017 2017 RELX revenue £7,341m RELX adjusted operating profit £2,284m Underlying revenue growth Underlying adjusted operating profit growth +6% +2% Exhibitions Exhibitions +2% Scientific, +11% Scientific, Technical & +3% Legal Technical & Medical Medical Legal +2% Risk & Risk & Business Business Analytics Analytics +8% +8% 5 | 6 Risk & Business Analytics 2017 revenue £2,073m Format Geography Type Face-to- Print Advertising face Rest of 3% 2% 2% World 5% Europe 15% Subscription 35% North Transactional Electronic America 63% 95% 80% 6 | 7 Delivering strong financial performance Underlying revenue growth Underlying adjusted operating profit growth +9% +9% +9% +8% +8% +8% +8% +7% +7% +6% +6% +6% 2013 2014 2015 2016 2017 2018 H1 2013 2014 2015 2016 2017 2018 H1 Adjusted operating margin Capital expenditure as a % of revenue 36.7% 36.7% 34.3% 35.2% 35.9% 36.0% 4% 4% 4% 3% 3% 2013 2014 2015 2016 2017 2018 H1 2013 2014 2015 2016 2017 7 Risk & Business Analytics revenue by segment Govern- ment Data Services Insurance • Evaluate and mitigate risk • Prevent fraud and cybercrime • Enable commerce Business Services Pro forma 2018 revenue Government includes healthcare products for government customers 8 | 9 Our four key capabilities • Deep customer understanding • Leading data sets: public records, contributory, licensed, proprietary • Sophisticated analytics • Powerful technology in global platforms 9 | 10 Strategic priorities for driving organic growth Core markets • Continuous product innovation to improve customer outcomes; effectiveness, efficiency and compliance • Drive deeper into innovative applications for additional decision points and increase penetration across customer workflows Adjacent markets • Pursue growth in attractive, close adjacent markets where our core strengths can be leveraged International • Address opportunities in selected geographies: leveraging skill sets, technology, analytics and experiences 10 | 11 Risk & Business Analytics underlying revenue growth +9% +8% +8% +7% +6% +6% Base market growth +5% contribution +3% Contribution from recent product introductions* 2011 2012 2013 2014 2015 2016 2017 2018 H1 * Less than 5 years old 11 | 12 12 Approach to technology, Artificial Intelligence and Machine Learning at RELX Group Vijay Raghavan Chief Technology Officer, Risk & Business Analytics 13 What do we mean by technology at RELX? Primary research Public records Entity resolution Proprietary data News Link analysis articles Contributory databases Fusion Big Unstructured Linking Clustering analysis Data records Structured records Refinery Complex analysis Unstructured and Sample capabilities structured content Big Data platforms Analysis applications • Over 3 petabytes of • Grid computing with low-cost servers • Scoring models & • Scientific author content attributes disambiguation • Data-centric languages (brings to code to the data) • 10s of billions of • Social graphs to • Recommendation engines records • Linking algorithms that generate high precision and recall identify patterns • Identity verification • 100s of thousands of • Machine learning algorithms to cluster, link and learn from the • Visualisations to sources data represent clusters, • Fraud detection and prevention • Integrated delivery system for high speed data fabrication links & graphs of • Billions of unique name entities & address combinations without compromising high-speed data retrieval • Case outcome prediction • Know your customer 14 RELX Group technology capabilities • $1.4bn annual technology spend • c8,000 technologists; c50% software engineers • Low attrition at 8% • Technology agnostic • Leverage approaches across RELX • Attract and retain talent 15 | 16 What we mean by Artificial Intelligence The science of getting computers to act without being explicitly programmed* • Artificial Intelligence (AI): a very large and diverse Artificial Intelligence part of Computer Science and Mathematics that enables machines to mimic human intelligence using logic, if-then rules, decision trees, knowledge-bases, or machine learning. • Machine Learning (ML): a subset of AI that uses statistical techniques that enable machines to Machine Learning improve with experience, in either a supervised or unsupervised fashion. • Deep Learning (DL): a subset of ML that enables software to train itself, to perform tasks such as speech and image recognition by exposing multi- layered neural networks to vast amounts of data. Deep Learning *Stanford University 16 | 17 AI/ML algorithms are plentiful Supervised Linear Regression, Random Forests, learning (uses Gradient Boosting, Deep Learning, performance data) Support Vector, Naïve Bayes…. AI/ML algorithms Types of learning Types of algorithms Unsupervised K-Means Clustering, Isolation Forests, learning (does not Hierarchical Clustering, Mahalanobis use performance Distance, PRIDIT…. data) Many popular algorithms are open-source and/or available from multiple providers 17 Knowing when to use which algorithm is critical Comparison of algorithms for auto claims fraud detection 16% 14% 12% 10% 8% 6% 4% Lift in fraud detection % detection fraud inLift 2% 0% Random Forest Gradient Deep Learning Support Vector Logistic PRIDIT Isolation Forest Boosting Machine Regression Machine Learning algorithm type R&BA example 18 | 19 Knowing how to create insights from big data is a core capability Source data Matched data sets Attributes & Scores Primary attributes Tax liens, felonies, • Summarise a particular characteristic bankruptcies of a consumer Property deeds • Examples include: – Number of addresses Professional – Tax assessed residence value licenses Compiled records Court judgments for more than Composite attributes 250m identities Landline & cell • Summarise aggregate consumer phone behaviour (wealth, income, Voter mobility) registration • Created by combining primary attributes Bureau header data Scores Other (education, etc) • Industry specific scores • Custom scores R&BA example 19 19 | 20 Growth in scoring models and attributes 2012 2015 2018 Scoring models <300 <600 >1,000 Attributes <5,000 <20,000 >60,000 R&BA example 20 R&BA Example 1: Driver Signature Use case: Insurers need to determine if an insured person is the driver or the passenger in a car for usage-based driver insurance Key customer issues: Solutions: Driver Signature Benefits to customer: • A smartphone app may • Algorithm predicts • Accurate scoring of track movement but the ‘likelihood of driver’ driver trips only insured may be the using collected data • Detection of non-driver driver or the passenger only trips without user • Second-by-second trip verification recording identifies location, timing, behaviour to identify driving habits • AI/ML used to calculate driver likelihood based on Driver Signature 21 | 22 Improved data and algorithms deliver better scoring over time Time Stamp Lat/Long Heading Speed Attribute Value Driver likelihood Frequent Place YES 2018-10-10 34.980010 364 32.324 07:30:31.499 -82.773442 Familiarity NO 2018-10-10 34.981203 363 33.419 60% 07:30:32.498 -82.772023 Average Speed YES 2018-10-10
Details
-
File Typepdf
-
Upload Time-
-
Content LanguagesEnglish
-
Upload UserAnonymous/Not logged-in
-
File Pages66 Page
-
File Size-