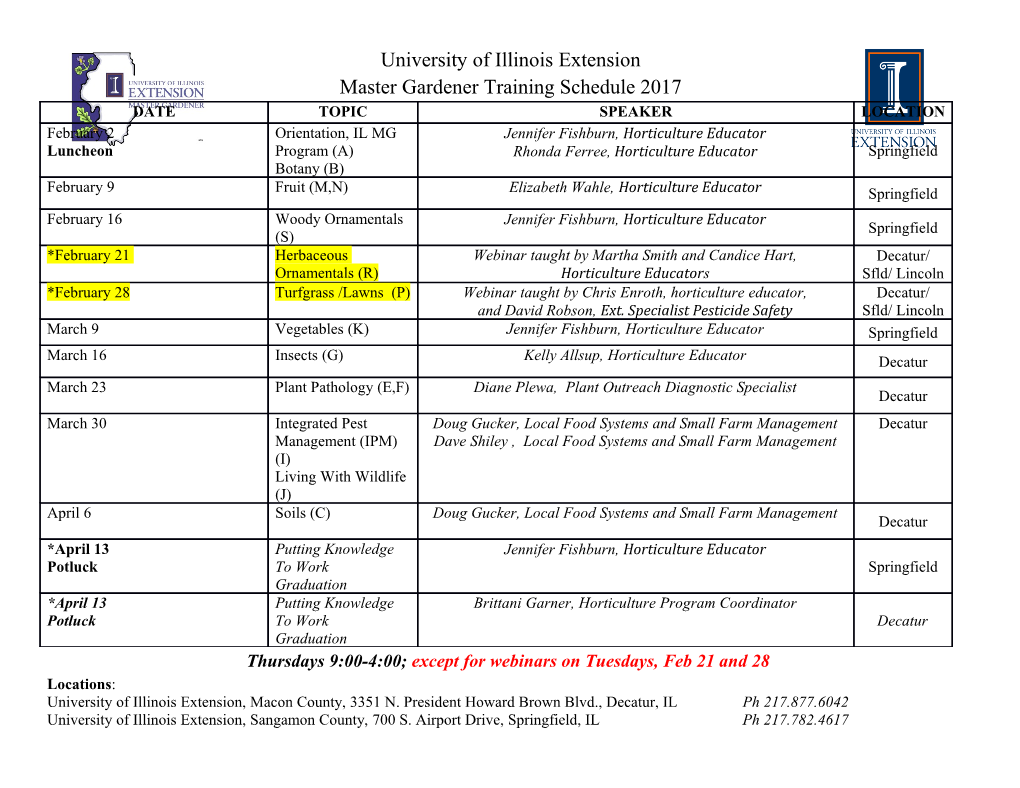
1 Quantitative determinants of aerobic glycolysis identify flux through the enzyme 2 GAPDH as a limiting step 3 4 Alexander A Shestov1*, Xiaojing Liu1*, Zheng Ser1, Ahmad Cluntun2, Yin P Hung3, Lei 5 Huang4, Dongsung Kim2, Anne Le5, Gary Yellen3, John G Albeck6, Jason W 6 Locasale1,2,4** 7 8 1Division of Nutritional Sciences, Cornell University, Ithaca NY 14850 9 2Field of Biochemistry and Molecular Cell Biology, Department of Molecular Biology 10 and Genetics Cornell University, Ithaca NY 14850 11 3Department of Neurobiology, Harvard Medical School, Boston MA 02115 12 4Field of Computational Biology, Department of Biological Statistics and Computational 13 Biology, Cornell University, Ithaca NY 14850 14 5Department of Pathology and Oncology, Johns Hopkins University School of Medicine, 15 Baltimore, MD 21231 16 6Department of Molecular Cell Biology, University of California Davis, Davis CA 95616 17 * Equal contribution 18 ** Correspondence: [email protected] 19 20 Abstract 21 Aerobic glycolysis or the Warburg Effect (WE) is characterized by the increased 22 metabolism of glucose to lactate. It remains unknown what quantitative changes to the 23 activity of metabolism are necessary and sufficient for this phenotype. We developed a 24 computational model of glycolysis and an integrated analysis using metabolic control 25 analysis (MCA), metabolomics data, and statistical simulations. We identified and 26 confirmed a novel mode of regulation specific to aerobic glycolysis where flux through 27 GAPDH, the enzyme separating lower and upper glycolysis, is the rate-limiting step in 28 the pathway and the levels of fructose (1,6) bisphosphate (FBP), are predictive of the rate 29 and control points in glycolysis. Strikingly, negative flux control was found and 30 confirmed for several steps thought to be rate-limiting in glycolysis. Together these 31 findings enumerate the biochemical determinants of the WE, and suggest strategies for 32 identifying the contexts in which agents that target glycolysis might be most effective. 33 34 Impact Statement 35 A computational model of the Warburg Effect is developed that reveals new mechanisms 36 for the regulation of glycolysis. These findings could lead to the definition of biomarkers 37 that determine the responses to targeting glycolysis in malignancy. 38 39 40 41 42 43 44 45 46 1 47 48 49 50 Introduction 51 52 Proliferating cells increase their glucose consumption and secrete lactate as 53 opposed to completely oxidizing the glucose in the mitochondria(Warburg, Wind et al. 54 1927) and is known as aerobic glycolysis or the Warburg Effect. Currently, this altered 55 metabolism is exploited for diagnostics and is subject to multiple drug development 56 efforts(Koppenol, Bounds et al. 2011; Vander Heiden 2011; Hamanaka and Chandel 57 2012). Numerous studies have identified genes such as KRAS, PIK3CA, and cMYC and 58 microenvironments such as hypoxia and hypoglycemia that promote aerobic glycolysis 59 but a complete understanding of the necessary and sufficient biochemical alterations 60 associated with this phenotype is unknown. Furthermore successful translation for 61 biomedical applications is limited by understanding the contexts in which therapies that 62 target glycolysis might be effective. 63 Computational modeling has a successful history in the study of 64 metabolism(Rapoport, Heinrich et al. 1976; Fell 1992; Schilling, Schuster et al. 1999; 65 Cascante, Boros et al. 2002). Genome-scale stoichiometric models of metabolism have 66 been developed to study the effects of drug targets in human metabolism and have had 67 success in predicting the WE(Molenaar, van Berlo et al. 2009; Vazquez, Liu et al. 2010; 68 Folger, Jerby et al. 2011; Shlomi, Benyamini et al. 2011). However a comprehensive 69 quantitative understanding of the WE requires knowledge of enzyme activities and 70 metabolic control. 71 Therefore, we collected and integrated multiple forms of data into a modeling 72 framework involving flux balances of glycolysis, detailed chemical kinetics based on 2 73 reaction mechanisms and parameters measured, physico-chemical constraints from 74 thermodynamics and mass conservation, metabolic control analysis, and Monte Carlo 75 sampling of parameter space. We next use mass spectrometry and isotope tracing to 76 probe concentrations and fluxes through the pathway and their responses to several 77 perturbations. Together we elaborate the determinants of aerobic glycolysis and identify 78 and confirm novel points of regulation in glycolysis that have remained unidentified for 79 over 50 years since the discovery of the pathway. 80 81 Results 82 Biochemical kinetic model of aerobic glycolysis 83 We investigated the kinetics of the glycolytic pathway from glucose uptake to 84 oxidation of pyruvate in the mitochondria or export of lactate out of the cytosol. We 85 modeled each step of the pathway according to enzymatic mechanism and known modes 86 of allosteric control resulting in a set of differential equations (Fig 1A, Materials and 87 Methods, Supplementary File 1). While it is not possible to model every possible 88 interaction explicitly, the aim is to capture enough of the pathway so that a large range of 89 experimentally realized measurements can be obtained and relationships between 90 variables can be observed. 91 Since glycolysis is the most extensively studied biochemical pathway, there is a 92 wealth of information on the kinetic parameters and enzyme expression that govern the 93 equations. Nevertheless, it is also not impossible to capture cellular physiology in any 94 biochemical model with single values of kinetic parameters(Daniels, Chen et al. 2008). 95 This difficulty arises from tremendous amount of heterogeneity within cells at multiple 3 96 levels. The origins of this heterogeneity vary from genetic variation observed across 97 cancer types, tumor types, differences in signaling mechanisms that affect post- 98 translational modifications in each cell, and the differences in microenvironmental 99 pressures (e.g. the oxygen availability) that each cell within a given tumor experiences, as 100 well as the inherent cell to cell variation common to all cells(Marusyk, Almendro et al. 101 2012). Therefore we developed an integrated algorithm to evaluate the statistics of the 102 kinetics of glycolysis that accounts for the possible variation within metabolism (Fig 1B, 103 Supplementary Information). 104 First the model is constrained using mass conservation constraints that conserve 105 the balance of glucose, redox state, and energy status. Next thermodynamic constraints 106 are used to constrain fluxes according to the free energy of the reactions determined by 107 Haldane relationships. These physical constraints are combined with the kinetic 108 mechanisms that define each step of glycolysis and the chemical reactions involving the 109 redox-associated metabolites NAD+, and NADH and energy-associated metabolites ATP, 110 ADP, and AMP. Next, the model is constrained to expression data so that protein 111 concentrations are subsumed in the Vmax values and chosen from typical concentrations 112 in cancer cells, measured kinetic parameters, and measured concentrations of nutrients 113 such as glucose, oxygen, and total intracellular adeno-nucleotide concentration. 114 At this stage, the model is subjected to a thorough statistical analysis. A Monte- 115 Carlo simulation is conducted for which the parameters within the model are randomized 116 and resulting differential equations solved. The distributions for each parameter are 117 chosen to capture observed ranges of variation. After each simulation, numerical stability 118 and the thermodynamics are assessed and all simulations that are unstable or appear 4 119 thermodynamically infeasible (i.e. a positive net flux through glycolysis is required) are 120 rejected. In each simulation, concentrations, fluxes, metabolic control coefficients, and 121 thermodynamic quantities are computed and recorded. This statistical analysis explores 122 the space of glycolysis in the context of the Warburg Effect. By assessing the statistics, 123 inferences can be made on the determinants of the Warburg Effects and its context 124 dependence to pharmacological intervention and nutrient environment. We first utilized 125 a recently developed a NADH/NAD+ fluorescent reporter(Hung, Albeck et al. 2011) and 126 measured the ratio of NADH/NAD+ across a population of cells where some experience 127 hypoxia and others glucose deprivation (Fig 1C). This variation in nutrient availability 128 across individual cells occurs in epithelial cell cultures due to differences in diffusion and 129 available cell surface area(Sheta, Trout et al. 2001). The distribution is plotted for three 130 media concentrations ranging from extreme hypoglycemia to the hyperglycemic 131 conditions typically used in cell culture (0.5 mM, 5.5mM, and 25mM). 132 The distribution of resulting fluxes (Fig 1D) was first found consistent with 133 known measurements. An analysis of the simulation revealed a distribution of NAD+ 134 levels and NADH/NAD+ redox potential consistent with those observed experimentally 135 (Figs 1E,F). ATP levels peaked around 3mM with little variation (Fig 1G) and the 136 ATP/ADP energy state is observed to be bimodal (Fig 1H). Concentrations along the 137 glycolytic pathway varied with means similar to those measured in normal tissues with 138 fructose-1,6-bisphosphate (FBP) being most variable (Fig 1I). Together, these findings 139 indicate
Details
-
File Typepdf
-
Upload Time-
-
Content LanguagesEnglish
-
Upload UserAnonymous/Not logged-in
-
File Pages37 Page
-
File Size-