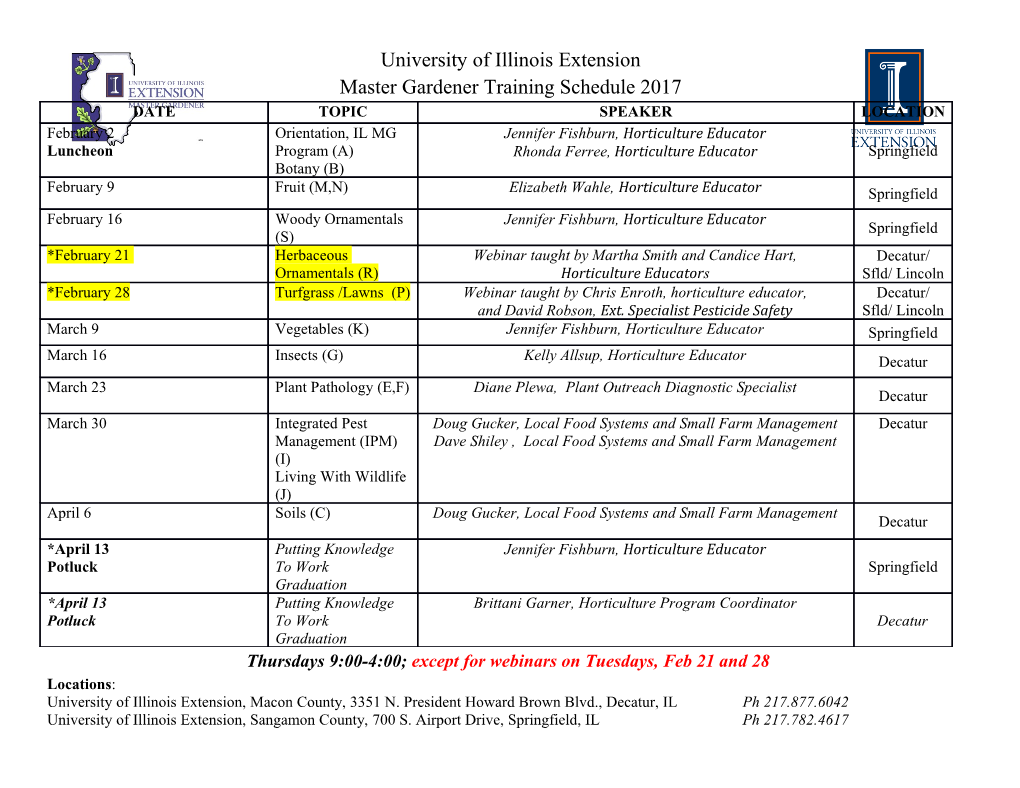
Automatic classification of folk narrative genres Dong Nguyen1, Dolf Trieschnigg1, Theo Meder2, Mariet¨ Theune1 1University of Twente, Enschede, The Netherlands 2Meertens Institute, Amsterdam, The Netherlands {d.nguyen,d.trieschnigg}@utwente.nl [email protected],[email protected] Abstract Folk narratives span a wide range of genres and in this paper we present work on identifying these Folk narratives are a valuable resource for genres. We automatically classify folk narratives humanities and social science researchers. as legend, saint’s legend, fairy tale, urban leg- This paper focuses on automatically recog- end, personal narrative, riddle, situation puzzle, nizing folk narrative genres, such as urban joke or song. Being able to automatically classify legends, fairy tales, jokes and riddles. We explore the effectiveness of lexical, struc- these genres will improve accessibility of narra- tural, stylistic and domain specific features. tives (e.g. filtering search results by genre) and We find that it is possible to obtain a good test to what extent these genres are distinguish- performance using only shallow features. able from each other. Most of the genres are not As dataset for our experiments we used the well defined, and researchers currently use crude Dutch Folktale database, containing narra- heuristics or intuition to assign the genres. tives from the 16th century until now. Text genre classification is a well-studied prob- lem and good performance has been obtained us- 1 Introduction ing surface cues (Kessler et al., 1997). Effective features include bag of words, POS patterns, text Folk narratives are an integral part of cultural her- statistics (Finn and Kushmerick, 2006), and char- itage and a valuable resource for historical and acter n-grams (Kanaris and Stamatatos (2007), contemporary comparative folk narrative studies. Sharoff et al. (2010)). They reflect moral values and beliefs, and identi- Finn and Kushmerick (2006) argued that genre ties of groups and individuals over time (Meder, classifiers should be reusable across multiple top- 2010). In addition, folk narratives can be studied ics. A classifier for folk narrative genres should to understand variability in transmission of narra- also be reusable across multiple topics, or in par- tives over time. ticular across story types1. For example, a narra- Recently, much interest has arisen to increase tive such as Red Riding Hood should not be clas- the digitalization of folk narratives (e.g. Meder sified as a fairy tale because it matches a story (2010), La Barre and Tilley (2012), Abello et al. type in the training set, but because it has charac- (2012)). In addition, natural language process- teristics of a fairy tale in general. This allows us ing methods have been applied to folk narrative to distinguish between particular genres, instead data. For example, fairy tales are an interest- of just recognizing variants of the same story. In ing resource for sentiment analysis (e.g. Moham- addition, this is desirable, since variants of a story mad (2011), Alm et al. (2005)) and methods have type such as Red Riding Hood can appear in other been explored to identify similar fairy tales (Lobo genres as well, such as jokes and riddles. and de Matos, 2010), jokes (Friedland and Al- lan, 2008) and urban legends (Grundkiewicz and 1Stories are classified under the same type when they Gralinski, 2011). have similar plots. 378 Proceedings of KONVENS 2012 (LThist 2012 workshop), Vienna, September 21, 2012 Most of the research on genre classification fo- Time period Frequency cused on classification of text and web genres. - 1599 8 1600 - 1699 11 To the best of our knowledge, we are the first to 1700 - 1799 24 automatically classify folk narrative genres. Our 1800 - 1899 826 dataset contains folk narratives ranging from the 1900 - 1999 8331 16th century until now. We first give an overview 2000 - 4609 Unknown 1154 of the dataset and the folk narrative genres. We then describe the experiments, discuss the results Table 1: Spread of data over time periods and suggest future work. Legends are situated in a known place and time, and occur in the recent past. They were regarded 2 Dataset as non-fiction by the narrator and the audience at 2.1 Overview the time they were narrated. Although the main characters are human, legends often contain su- Our dataset is a large collection of folk narratives pernatural elements such as witches or ghosts. collected in the Netherlands2. Although the col- Saint’s legends are narratives that are centered on lection contains many narratives in dialect, we a holy person or object. They are a popular genre restrict our focus to the narratives in standard in Catholic circles. Dutch, resulting in a total of 14,963 narratives. The narratives span a large time frame, with most Urban legends are also referred to as contem- of them from the 19th, 20th and 21th century, porary legends, belief legends or FOAF (Friend but some even dating from the 16th century as Of A Friend) tales in literature. The narratives can be seen in Table 13. Each narrative has been are legends situated in modern times and claimed manually annotated with metadata, such as genre, by the narrator to have actually happened. They named entities, keywords and a summary. tell about hazardous or embarrassing situations. Many of the urban legends are classified in a type- 2.2 Narrative genres index by Brunvand (1993). Folk narrative genres vary between cultures. Personal narratives are personal memories (not Legend, myth and folktales are major genres that rooted in tradition) that happened to the narrator are present in many cultures. Bascom (1965) himself or were observed by him. Therefore, the proposed a formal definition of these genres, stories are not necessarily told in the first person. based on belief, time, place, attitude and principal Riddles are usually short, consisting of a question characters of the narratives. In this work, we and an answer. Many modern riddles function as restrict our attention to genres that are applicable a joke, while older riddles were more like puzzles to the Dutch folk narratives. The selected genres to be solved. are described below and based on how annotators Situation puzzles, also referred to as kwispels assign narratives to genres in the Dutch Folktale (Burger and Meder, 2006), are narrative riddle database. games and start with the mysterious outcome of a plot (e.g. A man orders albatross in a restaurant, Fairy tales are set in an unspecified time (e.g. eats one bite, and kills himself ). The audience the well-known Once upon a time . ) and place, then needs to guess what led to this situation. The and are believed not to be true. They often have storyteller can only answer with ‘yes’ or ‘no’. a happy ending and contain magical elements. Jokes are short stories for laughter. The collec- Most of the fairy tales in the collection are classi- tion contains contemporary jokes, but also older fied under the Aarne-Thompson-Uther classifica- jokes that are part of the ATU index (Uther, 2004). tion system, which is widely used to classify and Older jokes are often longer, and do not necessar- organize folk tales (Uther, 2004). ily contain a punchline at the end. 2 Songs These are songs that are part of oral tradi- Dutch Folktale database: http://www.verhalenbank.nl/. tion (contrary to pop songs). Some of them have 3Although standard Dutch was not used before the 19th century, some narratives dating from before that time have a story component, for example, ballads that tell been recorded in standard Dutch. the story of Bluebeard. 379 Proceedings of KONVENS 2012 (LThist 2012 workshop), Vienna, September 21, 2012 Genre Train Dev Test Punctuation. The number of punctuation Situation puzzle 53 7 12 characters such as ? and ”, normalized by Saint’s legend 166 60 88 Song 18 13 5 total number of characters. Joke 1863 719 1333 Whitespace. A feature counting the number Pers. narr. 197 153 91 Riddle 755 347 507 of empty lines, normalized by total number Legend 2684 1005 1364 of lines. Included to help detect songs. Fairy tale 431 142 187 Text statistics. Document length, average Urban legend 2144 134 485 Total 8311 2580 4072 and standard deviation of sentence length, number of words per sentence and length of Table 2: Dataset words. III Domain knowledge. Legends are character- 2.3 Statistics ized by references to places, persons etc. We We divide the dataset into a training, development therefore consider the number of automati- and test set, see Table 2. The class distribu- cally tagged named entities. We use the Frog tion is highly skewed, with many instances of leg- tool (Van Den Bosch et al., 2007) to count ends and jokes, and only a small number of songs. the number of references to persons, organi- Each story type and genre pair (for example Red zations, location, products, events and mis- Riding Hood as a fairy tale) only occurs in one of cellaneous named entities. Each of them is the sets. As a result, the splits are not always even represented as a separate feature. across the multiple genres. IV Meta data. We explore the added value of 3 Experimental setup the manually annotated metadata: keywords, named entities and summary. Features were 3.1 Learning algorithm created by using the normalized frequencies We use an SVM with a linear kernel and L2 of their tokens. We also added a feature for regularization, using the liblinear (Fan et al., the manually annotated date (year) the story 2008) and scikit-learn libraries (Pedregosa et al., was written or told. For stories of which the 2011). We use the method by Crammer and date is unknown, we used the average date.
Details
-
File Typepdf
-
Upload Time-
-
Content LanguagesEnglish
-
Upload UserAnonymous/Not logged-in
-
File Pages5 Page
-
File Size-