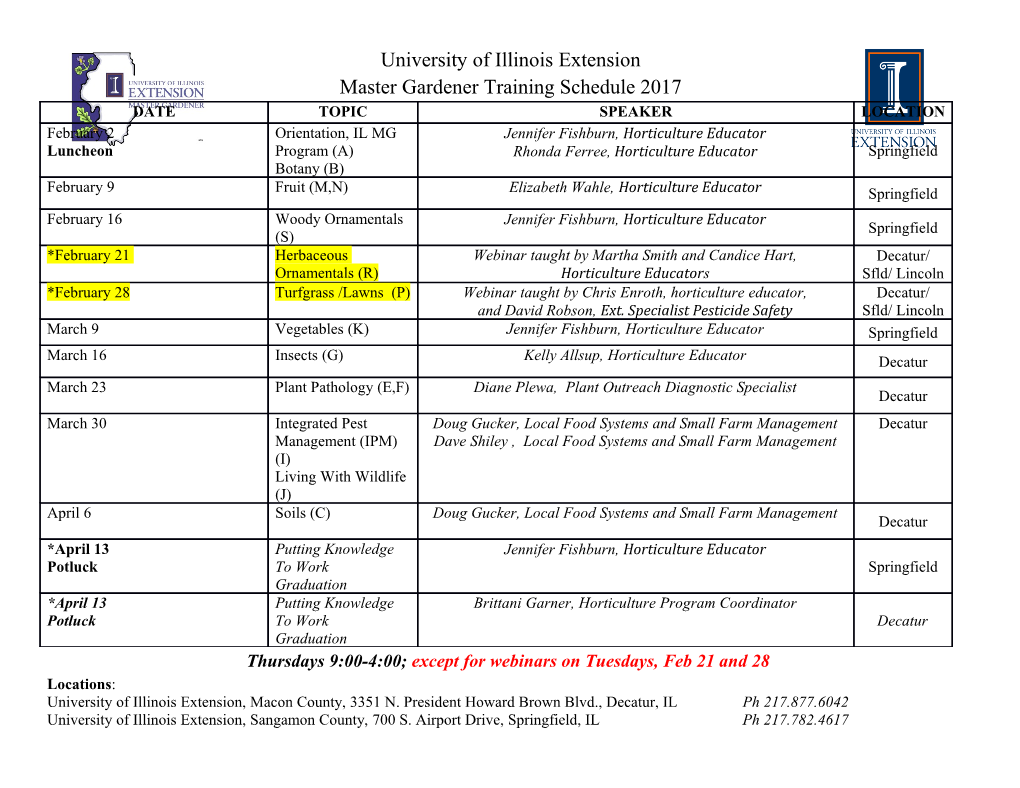
Ecological Modelling 252 (2013) 44–52 Contents lists available at SciVerse ScienceDirect Ecological Modelling journa l homepage: www.elsevier.com/locate/ecolmodel System dynamics modeling for urban energy consumption and CO2 emissions: A case study of Beijing, China ∗ Y.Y. Feng, S.Q. Chen, L.X. Zhang State Key Joint Laboratory of Environmental Simulation and Pollution Control, School of Environment, Beijing Normal University, Beijing 100875, China a r t i c l e i n f o a b s t r a c t Article history: It is clear that city must be part of the solution if an urbanizing world is to grapple successfully with Received 19 May 2012 ecological challenges such as energy depletion and climate change. A system dynamics model was devel- Received in revised form 7 September 2012 oped in this study using STELLA platform to model the energy consumption and CO2 emission trends for Accepted 8 September 2012 the City of Beijing over 2005–2030. Results show that the total energy demand in Beijing is predicted Available online 1 October 2012 to reach 114.30 million tonnes coal equivalent (Mtce) by 2030, while that value in 2005 is 55.99 Mtce, which is 1.04 times higher than the level in 2005. Accordingly, the total CO2 emissions in 2030 will reach Keywords: 169.67 million tonnes CO2 equivalent (Mt CO2-eq), 0.43 times higher than that of 2005. The change of System dynamics model energy structure from carbon rich fuel as coal to low-carbon fuel as natural gas will play a very essential Energy consumption role in carbon emission reduction activities of Beijing. The modeling results also shows that the service CO2 emissions STELLA sector will gradually replace the industrial dominant status in energy consumption as the largest energy consuming sector, followed by industrial and transport sector. The sensitive analysis suggests that change of economic development mode and control of rational population growth will have a far-reaching influ- ence on energy consumption and on carbon emissions. All these results will provide essential information for Beijing’s future energy and carbon emission profiles. © 2012 Elsevier B.V. All rights reserved. 1. Introduction other. A variety of factors associated with economic development, population growth and migration, climate change, and even con- More than one-half of the world’s population now lives in urban sumer behavioral patterns need to be considered simultaneously in areas, but they are blamed for producing as much as 80% of human- a simulation model. Thus, energy demand forecasting at different ity’s greenhouse gas (GHG) emissions. In China, for instance, the 35 temporal and spatial scales must fully account for varying levels of largest cities contain about 18% of the country’s population and system complexity. contribute to 40% of the country’s energy usage and CO2 emis- In the past decades, a number of studies were conducted for sions in 2006 (Dhakal, 2009). Therefore, they have played crucial planning energy management systems and controlling the asso- roles in determining the energy and carbon emission profiles of the ciated pollution gas and GHG emissions at the city level, with nation, and would be of great significance in achieving the country’s the classical energy-modeling approaches which can be catego- national targets such as energy security and climate change miti- rized into three types: top-down (Lu et al., 2010; Xu and Masui, gation (Zhang et al., 2011a,b; Chen and Chen, 2012; Li et al., 2010a; 2009), bottom-up (Phdungsilp, 2010; Gielen and Chen, 2001; Lin Zhang and Hu, 2011; Cai et al., 2009b). Systematic analysis and et al., 2010; Kanudia and Loulou, 1998; Cormio et al., 2003; Zhang dynamic modeling of urban energy demand and carbon emissions et al., 2011a; Feng and Zhang, 2012), and hybrid models (Bohringer are extremely important to understand and address issues associ- and Rutherford, 2009; Liu et al., 2011; Hadley and Short, 2001; ated with fuel security, energy system planning and management, Turton, 2008; Mirasgedis et al., 2007). Traditional energy models growth of GHG, and other emissions and investment decisions applied to the previous studies usually use deterministic forecasts (Akimoto et al., 2008; Vera and Langlois, 2007; Liu et al., 2009; Cai under assumption that we can precisely foresee the evolution of the et al., 2009a). However, it is challenging to achieve the desired pre- energy system. Most of them run with the aid of scenario analysis diction accuracy, because cities are dynamic systems of organized as a snapshot that describes a possible and plausible future, mak- complexity, with multiple independent variables that all affect each ing it difficult to adequately manage the dynamical evolution and the intrinsic stochastic behavior of many vital elements inside the urban energy system. In this context, system dynamics (SD) can be ∗ adopted to describe the inner interactions and structures impact- Corresponding author. Tel.: +86 10 59893229; fax: +86 10 59893229. E-mail address: [email protected] (L.X. Zhang). ing urban development and identify the desirable and undesirable 0304-3800/$ – see front matter © 2012 Elsevier B.V. All rights reserved. http://dx.doi.org/10.1016/j.ecolmodel.2012.09.008 Y.Y. Feng et al. / Ecological Modelling 252 (2013) 44–52 45 interventions, thus capable of illuminating the evolving process and system and depend on the computer simulation to quantitatively predicting the energy development trend of the whole city. analyze the structure of the information feedback system and the This study explores the intrinsic relationship between energy dynamic relation between function and behavior. SD is robust in demand and economic and social environment, which helps fore- that it could reflect the driving forces, incorporate individual sub- cast municipal energy demand and carbon emissions in a fast systems into a general framework and analyze their interactions, growing urban region. Taking the city of Beijing as a case, an inte- thereby providing holistic understanding of the whole system as grated system dynamics model was developed with a coupled well as the relevant policy responses for urban sustainability (Yuan modeling structure based on the framework of STELLA software, et al., 2008). However, it suffers from the shortcoming that all the which offered a realistic platform for predicting the trends of Bei- input data necessary for the operation of SD model need external jing’s energy demand and CO2 emissions from 2005 to 2030. The generation. SD model will hopefully improve our understanding on the inher- In fact, SD has been applied extensively to many areas including ent inter-linkages and dynamic evolutionary structures impacting social-economic systems (Forrester, 1969, 1971), ecological sys- future urban energy system development, and identify the signif- tems (Saysel and Barlas, 2001), transport systems (Suryani et al., icant contributors to urban energy demand and carbon emissions. 2010), environmental management and policy assessment, such as Such information is necessary for Beijing’s future energy planning solid waste management (Dyson and Chang, 2005), water resource and guidelines for policy-making, and highlights possible sugges- management (Stave, 2003; Rehan et al., 2011), GHG mitigation tions for urban energy conservation and carbon emission reduction. (Anand et al., 2005; Kunsch and Springael, 2008), sustainable eval- uation and environmental planning (Guo et al., 2001). Within the energy management regime, SD models have been developed and 2. Study area and methodology applied to national energy policy evaluation (Ford, 1983; Naill, 1992; Qudrat-Ullah, 2005), energy efficiency analysis (Dyner et al., 2.1. Overview of the study area 1995), and the development of energy industry (Bunn and Larsen, 1992; Chi et al., 2009). To the best of our knowledge, not much Beijing is the capital of China which is located on the north- attention has been paid to the research of urban energy and carbon west border of the North China Plain and is surrounded by emissions from the perspective of system dynamics. Yanshan Mountain in the west, north and northeast. Beijing occu- ® ® 2 It is important to note that the software Vensim and Stella are pies an administrative area of around 16,808 km , comprising of widely adopted by system dynamics models for simulation appli- 16 districts and 2 counties (Dong et al., 2012). Similar to other cations, which provide a user-friendly interface. In addition, they metropolises in developing countries, Beijing is undergoing rapid offer a flexible way to dynamically visualize and communicate how transformation, with a growing population, many new buildings, complex systems and ideas really work by building a variety of sim- and increasing automobile traffic. In 2010, Beijing’s GDP increased ulation models from causal loops or stock and flow (Isee System, 10.3% compared with the previous year, reaching 1411.36 billion ® 2006). In this study, the software package Stella was employed Chinese Yuan (CNY), and the per capita GDP was 71,938.43 CNY. to the model building of urban energy consumption and related The population of Beijing exceeded 19.61 million with the floating carbon emissions. population accounting for 35.89%, and the civil motor vehicle fleet exceeded 4.80 million units (including 3.74 million privately owned 2.3. Formulation of the Beijing-STELLA Model vehicles) at the end of 2010 (BMSB, 2011). The Beijing metropolis is also characterized by a scarcity of natural resources, demonstrated In this study, a system dynamics based computer simulation by the fact that all of the natural gas and crude oil consumed by model was developed, which has been named as Beijing-STELLA the city, as well as 95% of the coal, 64% of the electricity, and 60% of Model, for estimation and prediction of urban energy consump- the refined oil consumed has to be imported from outside (Li et al., tion trends and CO2 emissions in Beijing.
Details
-
File Typepdf
-
Upload Time-
-
Content LanguagesEnglish
-
Upload UserAnonymous/Not logged-in
-
File Pages9 Page
-
File Size-