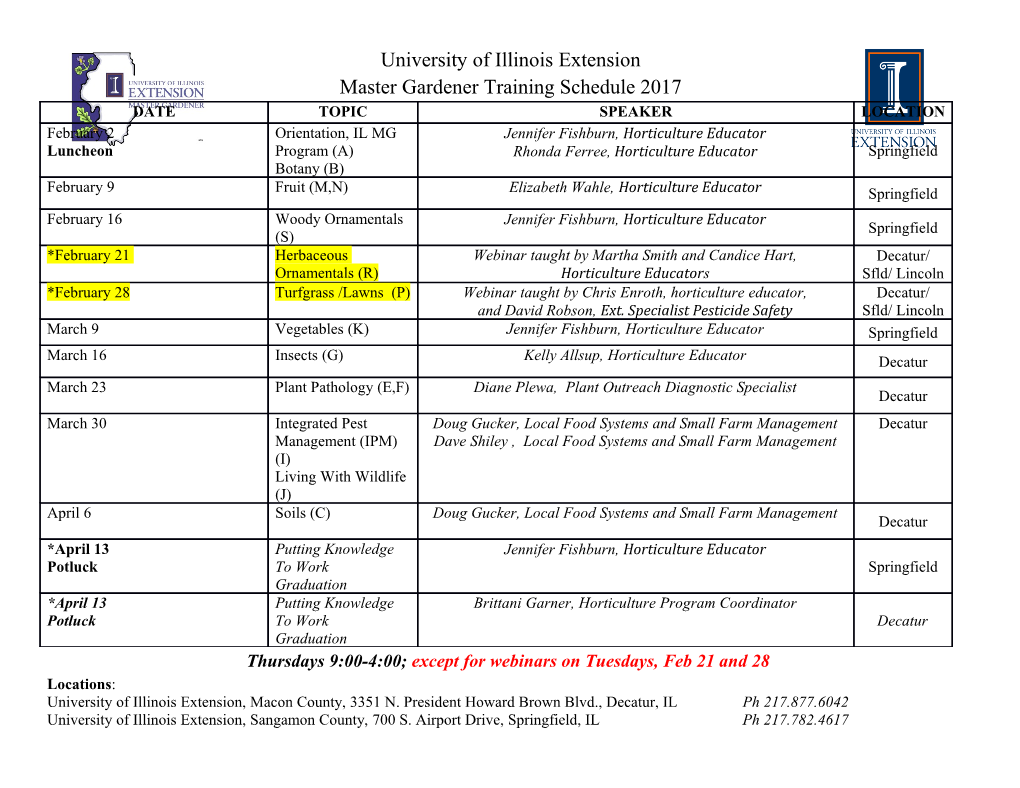
Attractor Metafeatures and Their Application in Biomolecular Data Analysis Tai-Hsien Ou Yang Submitted in partial fulfillment of the requirements for the degree of Doctor of Philosophy in the Graduate School of Arts and Sciences COLUMBIA UNIVERSITY 2018 ©2018 Tai-Hsien Ou Yang All rights reserved ABSTRACT Attractor Metafeatures and Their Application in Biomolecular Data Analysis Tai-Hsien Ou Yang This dissertation proposes a family of algorithms for deriving signatures of mutually associated features, to which we refer as attractor metafeatures, or simply attractors. Specifically, we present multi-cancer attractor derivation algorithms, identifying correlated features in signatures from multiple biological data sets in one analysis, as well as the groups of samples or cells that exclusively express these signatures. Our results demonstrate that these signatures can be used, in proper combinations, as biomarkers that predict a patient’s survival rate, based on the transcriptome of the tumor sample. They can also be used as features to analyze the composition of the tumor. Through analyzing large data sets of 18 cancer types and three high-throughput platforms from The Cancer Genome Atlas (TCGA) PanCanAtlas Project and multiple single-cell RNA-seq data sets, we identified novel cancer attractor signatures and elucidated the identity of the cells that express these signatures. Using these signatures, we developed a prognostic biomarker for breast cancer called the Breast Cancer Attractor Metagenes (BCAM) biomarker as well as a software platform to analyze the tumor sample, called Analysis of the Single-Cell Omics for Tumor (ASCOT). Contents List of Figures ................................................................................................................................. ii List of Tables ................................................................................................................................. iii Acknowledgements ........................................................................................................................ iv Chapter 1 Introduction .................................................................................................................... 1 1.1 Background .......................................................................................................................... 1 1.2 Prior Work on Cancer Signature Identification .................................................................... 6 1.3 Contributions of the Thesis ................................................................................................ 14 Chapter 2 Derivation of the Attractor Metafeatures ..................................................................... 17 2.1 Introduction ........................................................................................................................ 17 2.2 Definitions .......................................................................................................................... 17 2.3 Implementation ................................................................................................................... 32 2.4 Comparison with Previous Work ....................................................................................... 33 Chapter 3 Attractor Biological Signatures .................................................................................... 37 3.1 Introduction ........................................................................................................................ 37 3.2 Multicancer Signatures ....................................................................................................... 37 3.3 Single-Cell-Attractor Signatures ........................................................................................ 53 Chapter 4 Prognostic Cancer Biomarker ...................................................................................... 61 4.1 Introduction ........................................................................................................................ 61 4.2 Methods .............................................................................................................................. 62 4.3 Results ................................................................................................................................ 72 4.4 Discussion .......................................................................................................................... 79 Chapter 5 Tumor Analysis Using Single-Cell Transcriptomes .................................................... 85 5.1 Introduction ........................................................................................................................ 85 5.2 Methods .............................................................................................................................. 88 5.3 Results ................................................................................................................................ 91 5.4 Discussion .......................................................................................................................... 96 Chapter 6 Conclusions and Future Work ...................................................................................... 98 6.1 Single-Cell Epigenomic-Attractor Signatures .................................................................... 99 6.2 Reconstruction of the evolution and the development processes of the cell populations 100 6.3 Predictive markers for precision medicine in cancer ....................................................... 101 Bibliography ............................................................................................................................... 103 Appendix A Comparison of the Consensus Attractors and the Attractor Clusters ..................... 117 Appendix B The Consensus Attractors in the TCGA PanCanAtlas Data Sets ........................... 118 Appendix C Significance of Consistency of the Attractor Signatures ........................................ 123 Appendix D The Single Cell Consensus Attractors .................................................................... 125 Appendix E Interrelationships and Concordance Indices of the Attractor Signatures ............... 126 Appendix F Cell-Specific Expression of the Single Cell Consensus Attractors ........................ 130 i List of Figures Figure 2.1 (A) Strength and (B) number of attractors against exponent 휶 for the consensus- attractor derivation method in TCGA bulk data sets. ............................................................30 Figure 2.2 (A) Strength and (B) number of attractors against exponent 휶 for the consensus- attractor derivation method in scRNA-seq data sets. .............................................................30 Figure 3.1 Scatter plots of(A) CD53, SASH3, IL10RA in 18 TCGA data sets, (B) Leukocyte, M+, M- attractor metafeatures in 17 TCGA data sets ...........................................................45 Figure 3.2 Scatter plots of (A) the T-cell signature and (B) the B-cell signature against the mitosis signature. ...................................................................................................................57 Figure 3.3 Top hits of the MSigDB gene-set enrichment analysis against the top genes of the stem-like signature. ................................................................................................................59 Figure 3.4 Scatter plots of the stem-like signature against the fibroblast signature (A) in the melanoma data set and (B) in the breast cancer data set. .......................................................60 Figure 3.5 Boxplots of the expression levels of the stem-like signature by type of cells in (A) the melanoma data set and in (B) the breast cancer data set. .................................................60 Figure 4.1 Screenshot of the feature-selector facility. ...................................................................66 Figure 4.2 Scatter plot of the YAP1 and the SMAD6 proteins in the AML patients. ...................70 Figure 4.3 Scores of the optimal combinations of different numbers of the features. ...................74 Figure 4.4 Estimated breast cancer-specific ten-year survival rate as a function of the BCAM score. ......................................................................................................................................76 Figure 4.5 Kaplan–Meier plots of (A) FGD3-SUSD3 and (B) ESR1 in breast cancer data sets. ..78 Figure 4.6 Frequency distributions of BCAM scores for subgroups of ER-negative breast cancer cases. ...........................................................................................................................82 Figure 5.1 Relationships of the exponent for metacell discovery and the number of discovered metacells in the annotated scRNA-seq data set GSE36552.................................90 Figure 5.2 t-SNE plot of the classification result of the samples from (A) melanoma patient CY79 and (B) melanoma patient CY84. ................................................................................93 Figure 5.3 Boxplots of the stem-like signatures by the type of cells in (A) the cells from patient CY79 and (B) the cells from patient CY84 of the melanoma data set. ......................94 Figure 5.4 t-SNE plot of the cell populations in the breast cancer data set. ..................................96 ii List of Tables Table 3.1 List
Details
-
File Typepdf
-
Upload Time-
-
Content LanguagesEnglish
-
Upload UserAnonymous/Not logged-in
-
File Pages139 Page
-
File Size-