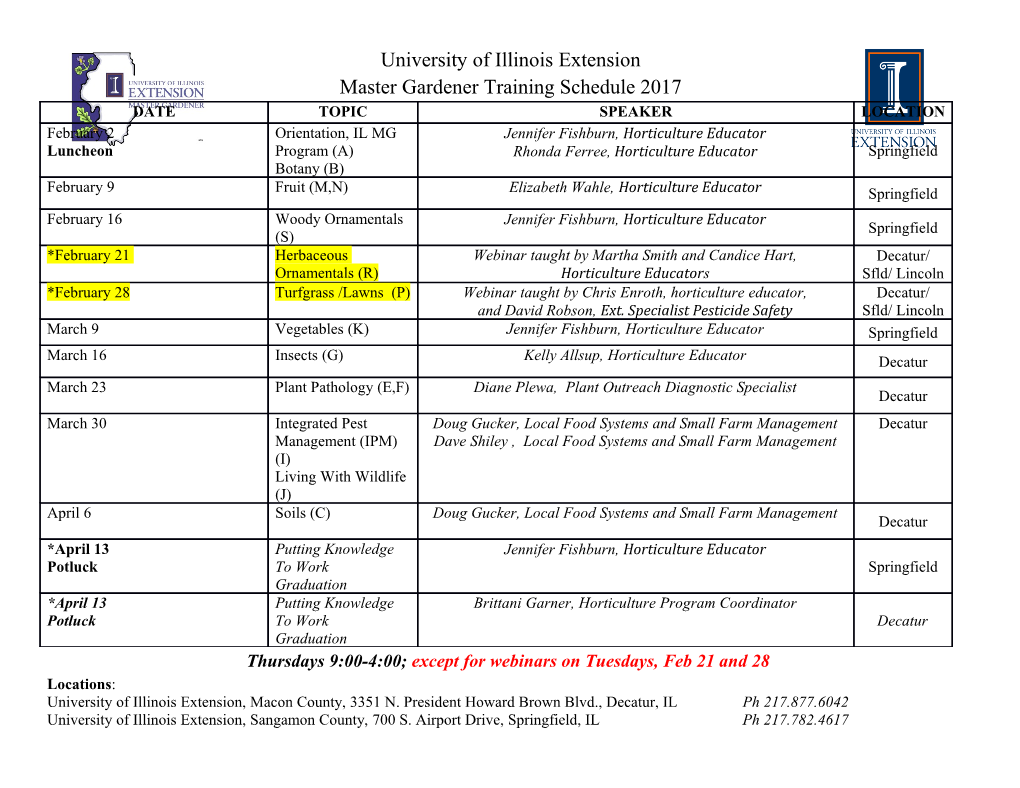
Use Process Capability to Ensure Product Quality Lawrence X. Yu, Ph.D. Director (acting) Office of Pharmaceutical Science, CDER, FDA FDA/ PQRI Conference on Evolving Product Quality September 16-17, 2104, Bethesda, MD 1 2 Quality by Testing vs. Quality by Design Quality by Testing – Specification acceptance criteria are based on one or more batch data (process capability) – Testing must be made to release batches Quality by Design – Specification acceptance criteria are based on performance – Testing may not be necessary to release batches L. X. Yu. Pharm. Res. 25:781-791 (2008) 3 ICH Q6A: Test Procedures and Acceptance Criteria… 4 5 Pharmaceutical QbD Objectives Achieve meaningful product quality specifications that are based on assuring clinical performance Increase process capability and reduce product variability and defects by enhancing product and process design, understanding, and control Increase product development and manufacturing efficiencies Enhance root cause analysis and post-approval change management 6 Concept of Process Capability First introduced in Statistical Quality Control Handbook by the Western Electric Company (1956). – “process capability” is defined as “the natural or undisturbed performance after extraneous influences are eliminated. This is determined by plotting data on a control chart.” ISO, AIAG, ASQ, ASTM ….. published their guideline or manual on process capability index calculation 7 Nomenclature Four indices: – Cp: process capability index – Cpk: minimum process capability index – Pp: process performance index – Ppk: minimum process performance index ASTM E2281: Standard Practice for Process and Measurement Capability Indices 8 Calculation Formula (USL LSL) C (USL LSL) p P 6 p 6SD Mean LSL Cpkl Mean LSL Ppkl 3 3SD USL Mean USL Mean Cpku Ppku 3 3SD Cpk= min (Cpkl, Cpku) Ppk= min (Ppkl, Ppku) USL: upper specification limit; LSL: lower specification limit; Mean: grand average of all the data Sigma hat: estimated inherent variability (noise) of a stable process SD: overall variability 9 A Perfectly Centered Process… USL LSL For this case: LSL USL USL= +4σ LSL = -4σ USL-LSL= 8σ Cp= 1.333 Cpku=1.333 Cpkl=1.333 Cpk=1.333 -5 -4 -3 -2 -1 0 1 2 3 4 5 Mean (μ ), Sigma (σ) 10 Process Mean is not Centered… For this case: USL= +4σ LSL = -4σ USL-LSL= 8σ Cp= 1.333 Cpkl = 1.667 Cpku = 1.0 Cpk= 1.0 When the process is not centered, or deliberately run off-center for economic reasons, or only a single specification limit is involved, Cpk should be used. Similarly, Ppk offsets Pp weakness by introducing process mean in the calculation formula. 11 Cpk, Sigma Value, and PPM Area under Non conforming parts per million (ppm) Cpk Sigma normal Capability Value Value distribution Rating** Unilateral specification Bilateral specification* curve (%)* 0.333 1 68.27 158650 317300 Terrible 0.667 2 95.45 22750 45500 Poor Marginally 1.0 3 99.73 1350 2700 capable 1.333 4 99.993636 32 64 Capable 1.667 5 99.999942 0.29 0.58 Good 2.0 6 99.9999998 0.001 0.002 Excellent *Process mean is centered at middle of the specification limits and has normal distribution **Bothe, D. R., Measuring Process Capability, Cedarburg, W.I., Landmark Publishing Inc., 2001 12 Difference between Cpk and Ppk inherent variability overall variability R MR S N (X X )2 or or SD i d2 d2 c4 i1 N 1 SD: standard deviation of all individual (observed) values, which accounts for both common cause variability (noise) and special cause variability. It is often referred as overall variability. : the inherent variability (noise) due to common cause of a stable process. It is often estimated by using within subgroup variability which is linked to the use of control charts. 13 Difference between Cpk and Ppk Cpk represents the potential process capability (i.e. how well a given process could perform when all special causes have been eliminated). Ppk addresses how the process has performed without the demonstration of the process to be stable. Forecast future batch failure rate – Cpk (Yes) ; Ppk (No) 14 Control Chart CL: the grand average UCL and LCL: • Typically: 3SD from CL • Should not be confused with upper and lower specification limits To evaluate if a process is in a state of statistical control – Western Electric 8 Rules Two Types of Control Chart – Variable control chart: continuous numeric measurements (e.g. assay, dissolution, uniformity, impurity level) – Attribute control chart: discrete data (pass or fail, or counts of defects) 15 Variable Control Chart The average chart (X-bar chart) The variability chart – Moving range chart (MR chart, n=1) – Range chart (R-chart, subgroup size 2-10) – Standard deviation chart (S-chart, subgroup size >10) The average and variability charts are usually prepared and analyzed in pairs. 16 Example Xbar-R Chart Process Capability Analysis of Tablet Assay (first 25 batches, subgroup size =3) Xbar Chart Capability Histogram UCL=102.108 LSL USL n 102 a Specifications e _ M _ LSL 96 p X=100.287 u 100 USL 104 o r g b u LCL=98.466 S 98 1 3 5 7 9 11 13 15 17 19 21 23 25 Batch No. 96 98 100 102 104 R Chart Normal Prob Plot A D: 0.636, P: 0.094 e UCL=4.582 g 4 n a R p _ u 2 o R=1.78 r g b u S 0 LCL=0 1 3 5 7 9 11 13 15 17 19 21 23 25 Batch No. 96 99 102 105 Run Chart Capability Plot Cp: 1.27 104 Within Within Overall ) 102 StDev 1.051 StDev 1.079 % Cp 1.27 Pp 1.24 ( 100 Cpk 1.18 Ppk 1.15 y Overall Cpk: 1.18 a s PPM 229.14 Cpm * s 98 A PPM 323.15 96 Specs 5 10 15 20 25 Ppk: 1.15 Batch No. Data source: Chopra, V., Bairagi, M., Trivedi, P., et al., “A case study: application of statistical process control tool for determining process capability and sigma level,” PDA J Pharm Sci and Tech, 66 (2), 2012, pp. 98-115 17 Attribute Control Chart Control chart for fraction occurrence of an event (p chart) – For example: % of unsuccessful batch at Site A every month – Binominal distribution Control chart for counts of occurrence in a defined time or space increment (c chart) – For example: number of particulate matter in an injection vial – Poisson distribution Other types of control chart: – cumulative sum control chart (CUSUM) – exponentially weighted moving average control charts (EWMA) – etc. ASTM E2587- Standard Practice for Use of Control Charts in Statistical Process Control 18 Example P chart % of “unsuccessful batch”/month at Site A (# of lots attempted: 20-30/month) Binomial Process Capability Analysis of Unsuccess Batch P Chart Unsuccess Rate e UC L=0.1809 t a 0.15 R 20 n o s i s t e r 0.10 c o c p 10 u _ o s r 0.05 P=0.0437 n P U 0.00 LC L=0 0 % 1 3 5 7 9 11 13 15 17 19 21 23 25 20 25 30 Month T otal Batch Manufactured/Month Tests performed w ith unequal sample sizes Histogram Cumulative Unsuccess Rate Process-Z: Tar e Summary Stats t a 10.0 (95.0% confidence) 1.709 R 6 s s % Defectiv e: 4.37 Binomial process e 7.5 y c 5 Low er C I: 2.79 c c u n s capability index: Upper C I: 6.49 e n 4 u 5.0 q U Target: 0.00 e e 0.569 PPM Def: 43726 r v 3 F i t 2.5 a Low er C I: 27917 l Lower 95% u 2 Upper C I: 64891 m u Process Z: 1.7090 0.0 confidence bound C 5 10 15 20 25 Low er C I: 1.5150 0 3 6 9 12 0.505 Month Upper C I: 1.9123 % Unsuccess Rate Similar principles can be used to evaluate process capability of a single product, a product class, different manufacture sites, or a manufacturer global sites. 19 Summary: Process Capability Indices Patient first: clinical relevant specification Consider not only process mean & variability but also in relation to the specification Quantitative and action enabling Applicable for cross sectors (brand, generic, OTC and biotech) No additional testing is required since batch release data is available per current regulation A simple and powerful indicator to ensure product quality and process robustness. 20 Acknowledgements Daniel Peng Alex Viehmann Karthik Iyer 21 .
Details
-
File Typepdf
-
Upload Time-
-
Content LanguagesEnglish
-
Upload UserAnonymous/Not logged-in
-
File Pages21 Page
-
File Size-