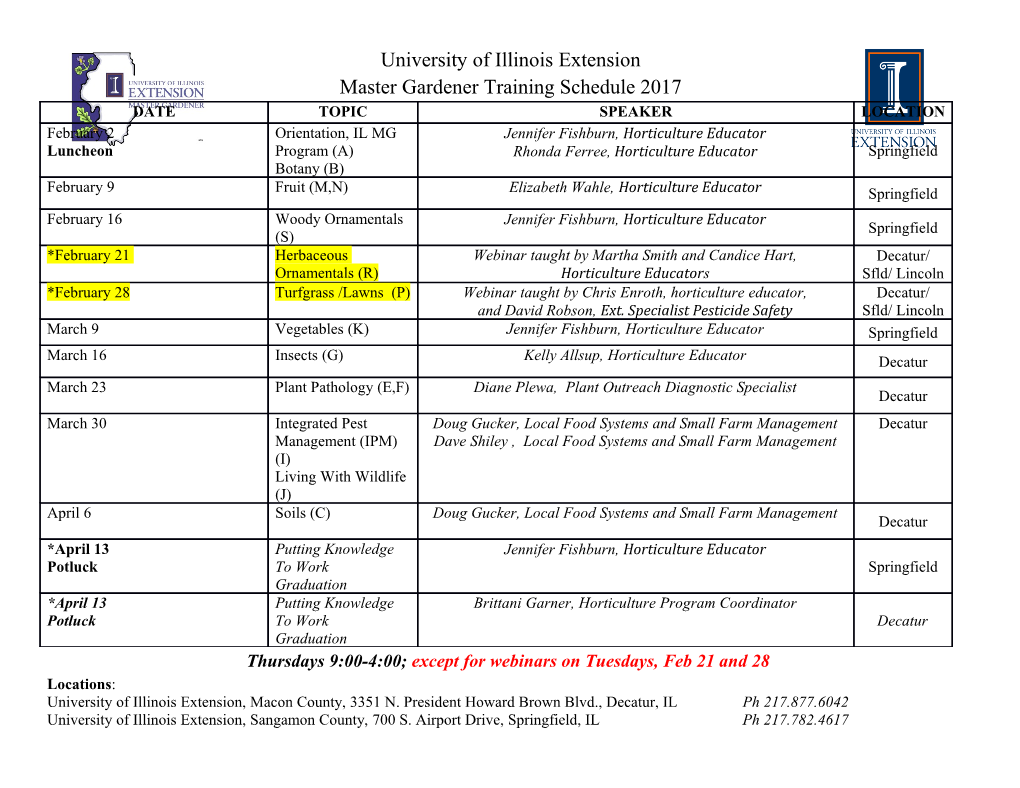
EEM 463 Introduction to Image Processing Week 5: Image Restoration and Reconstruction Fall 2013 Instructor: Hatice Çınar Akakın, Ph.D. [email protected] Anadolu University 12.11.2013 Image Restoration • Image restoration: recover an image that has been degraded by using a prior knowledge of the degradation phenomenon. • Model the degradation and applying the inverse process in order to recover the original image. • The principal goal of restoration techniques is to improve an image in some predefined sense. • Although there are areas of overlap, image enhancement is largely a subjective process, while restoration is for the most part an objective process. 12.11.2013 A Model of the Image Degradation/Restoration Process • The degraded image in the spatial domain: 푔 푥, 푦 = ℎ 푥, 푦 ∗ 푓 푥, 푦 + 휂 푥, 푦 • Frequency domain representation 퐺 푢, 푣 = 퐻 푢, 푣 퐹 푢, 푣 + 푁(푢, 푣) 12.11.2013 Noise Models • The principal sources of noise in digital images arise during image acquisition and/or transmission • Light levels and sensor temperature during acquisition • Lightning or other atmospheric disturbance in wireless network during transmission • White noise: Fourier spectrum this noise is constant • carryover from the physical proporties of White light, which contains nearly all frequencies in the visible spectrum in equal proportions. • With the exception of spatially periodic noise, we assume • Noise is independent of spatial coordinates • Noise is uncorrelated with respect to the image itself 12.11.2013 Gaussian Noise • The pdf of a Gaussian random variable, z, is given by 1 2 푝 푧 = 푒−(푧−푧) /2휎2 2휋휎 where z represents intensity, 푧 is the mean (average) value of z , and σ is its standard deviation. • 70% of its values will be in the range ( ),( ) • 95% of its values will be in the range ( 2),( 2) 12.11.2013 Rayleigh Noise • The pdf ofThe Rayleigh PDF ofnoise Rayleighis given noiseby is given by 2 2 (z a ) e(z a ) / b for z a pz( ) b 0 for za The mean and variance of this density are given by z a b / 4 b(4 ) 2 4 12.11.2013 Erlang (Gamma) Noise • The pdf of ErlangThe PDFnoise of isErlang given noiseby is given by azbb1 ezaz for 0 pz( ) (b 1)! 0 for za • where a > 0 and b is a positive integer. The mean and variance of this density are given by The mean and variance of this density are given by z b/ a 22 ba/ 12.11.2013 Exponential Noise • The PDF ofThe exponential PDF of exponential noise is given noise by is given by aeaz for z 0 pz( ) 0 for za where a > 0 . The mean and variance of this density are given by The mean and variance of this density are given by za1/ 221/ a 12.11.2013 Uniform Noise • The PDF ofThe uniform PDF noiseof uniform is given noise by is given by 1 for a zb pz( ) ba 0 otherwise • The mean andThe variance mean and of variance this density of this function density ar aree given given by by z( a b ) / 2 22(ba ) /12 12.11.2013 Impulse (Salt-and-Pepper) Noise • The PDF ofThe impulse PDF of noise (bipolar) is given impulse by noise is given by Pa for z a p( z ) Pb for z b 0 otherwise • If b > a , intensity b appears as a light dot in the image. Conversely, intensity a will appear like a dark dot. • If either Pa or Pb is zero, the impulse noise is called unipolar. 12.11.2013 12.11.2013 • Example: Original test image 12.11.2013 12.11.2013 12.11.2013 Periodic Noise • Periodic noise in an image arises typically from electrical or electromechanical interference during image acquisition. • It can be reduced significantly by using frequency domain filtering 12.11.2013 Estimation of Noise Parameters • The parameters of periodic noise can be estimated by inspection of the Fourier spectrum of the image. • Periodic noise tends to produce frquency spikes, which are detectable even by visual analysis • Automated analysis is possible if the noise spikes are either exceptionally pronounced, or when knowledge is available about the general location of the frequency components of the interference. 12.11.2013 • It is often necessary to estimate the noise probability density functions for a particular imaging arrangement. • When images already generated by a sensor are available, it may be possible to estimate the parameters of the probability density functions from small patches of reasonably constant background intensity. The shape of the histogram identifies the closest PDF match 12.11.2013 Consider a subimage denoted by S, and let psi( z ), i 0, 1, ..., L -1, • If thedenoteimage thestripe probability(subimage estimates) S is ofgiven the ,intensities of the pixels in S. • CalculateThe meanmean andand variancevariance of ofthe intensity pixels inlevels S: L1 z zi p s( z i ) i0 L1 22 and (zi z ) p s ( z i ) i0 where S denote a stripe and pS (zi ) , i = 0,1,2,...,L −1, denote the probability estimates of the intensities of the pixels in S • The shape of the histogram identifies the closest probability density function match. • The Gaussian probability density function is completely specified by these two parameters. 12.11.2013 Restoration in the Presence of Noise Only ̶ Spatial Filtering • When theNoise only degradationmodel without present degradation in an image is noise, g( x , y ) f ( x , y ) ( x , y ) and G( u , v ) F ( u , v ) N ( u , v ) • Since the noise terms are unknown, subtracting them from g(x,y ) or G(u,v ) is not a realistic option. 12.11.2013 Mean Filters • Arithmetic mean filter Let Sxy represent the set of coordinates in a rectangle subimageArithmetic window mean of sizefilter m n, centered at ( x , y). 1 f( x , y ) g ( s , t ) mn (,)s t Sxy 12.11.2013 • GeometricGeometricmean meanfilter filter 1 mn f( x , y ) g ( s , t ) (,)s t Sxy • A geometric mean filter achieves smoothing comparable to the arithmetic mean filter, but it tends to lose less image detail in the process. 12.11.2013 • HarmonicHarmonicmean filter mean filter mn f( x , y ) 1 (,)s t Sxy g(,) s t which works well for some types of noise like Gaussian noise and salt noise, but fails for pepper noise. 12.11.2013 • ContraharmonicContraharmonicmean filter mean filter Q1 g(,) s t Q>0 for pepper noise and Q<0 for salt (,)s t Sxy f( x , y ) noise. g(,) s t Q where Q is called the order of the(,)s filter t Sxy. • It is well suited for reducing or eliminating the effects of salt-and-pepper noise. • When Q = 0 , the contraharmonic mean filter reduces to the arithmetic mean filter. • When Q = −1 , the contraharmonic mean filter becomes the harmonic mean filter. 12.11.2013 Example corrupted version with additive Gaussian noise of zero mean and variance of 400. In general, the arithmetic and geometric mean filters are suited for random noise like Gaussian or uniform noise. 12.11.2013 • The positive-order filter did a better job of cleaning the background, at the expense of slightly thinning and blurring the dark areas. • The opposite was true of the negative- order filter. 12.11.2013 The contraharmonic mean filter is well suited for impulse noise, with the disadvantage that it must be known whether the noise is dark or light in order to select Q . 12.11.2013 Order Statistic Filters The median filters are Median filter particularly effective in the presence of both bipolar and unipolar f( x , y ) median g ( s , t ) impulse noise. (,)s t Sxy Max filter f( x , y ) max g ( s , t ) (,)s t Sxy Min filter f( x , y ) min g ( s , t ) (,)s t Sxy 12.11.2013 The midpoint filter works best for Midpoint filter random distributed noise, 1 like Gaussian or uniform noise. f( x , y ) max g ( s , t ) min g ( s , t ) (,)s t S 2 (,)s t Sxy xy • Suppose that, we delete d /2 lowest and the d /2 highest intensity values of g(s,t) in Sxy . Let gr (s,t) represent the remaining mn −d pixels, an alpha-trimmed mean filter is given by Alpha-trimmed mean filter 1 When d = 0 , the alpha-trimmed f( x , y ) g ( s , t ) mean filter is reduced to the mn d r arithmetic mean filter. (,)s t Sxy If d = mn −1 , the alpha-trimmed mean filter becomes a median filter. 12.11.2013 Note that, repeated passes of a median filter will blur the image, so keep the number of passes as low as possible!! 12.11.2013 The min filter did a better job on noise removal, but it removes some white points around the 12.11.2013 border of light objects. 12.11.2013 Adaptive filters • Adaptive filters are capable of performance superior to that of the filters discussed thus far. However, the price paid for improved filtering power is an increase in filter complexity. • The behavior changes based on statistical characteristics of the image inside the filter region defined by the mхn rectangular window. 12.11.2013 Adaptive, Local Noise Reduction Filters Sxy : local region The response of the filter at the center point (x,y) of Sxy is based on four quantities: The mean gives a measure of average intensity in the (a) g( x , y ), the value of the noisy image at ( x , y ); region over which the mean 2 is computed, and the (b) , the variance of the noise corrupting f( x , y ) variance gives a measure of contrast in that region.
Details
-
File Typepdf
-
Upload Time-
-
Content LanguagesEnglish
-
Upload UserAnonymous/Not logged-in
-
File Pages55 Page
-
File Size-