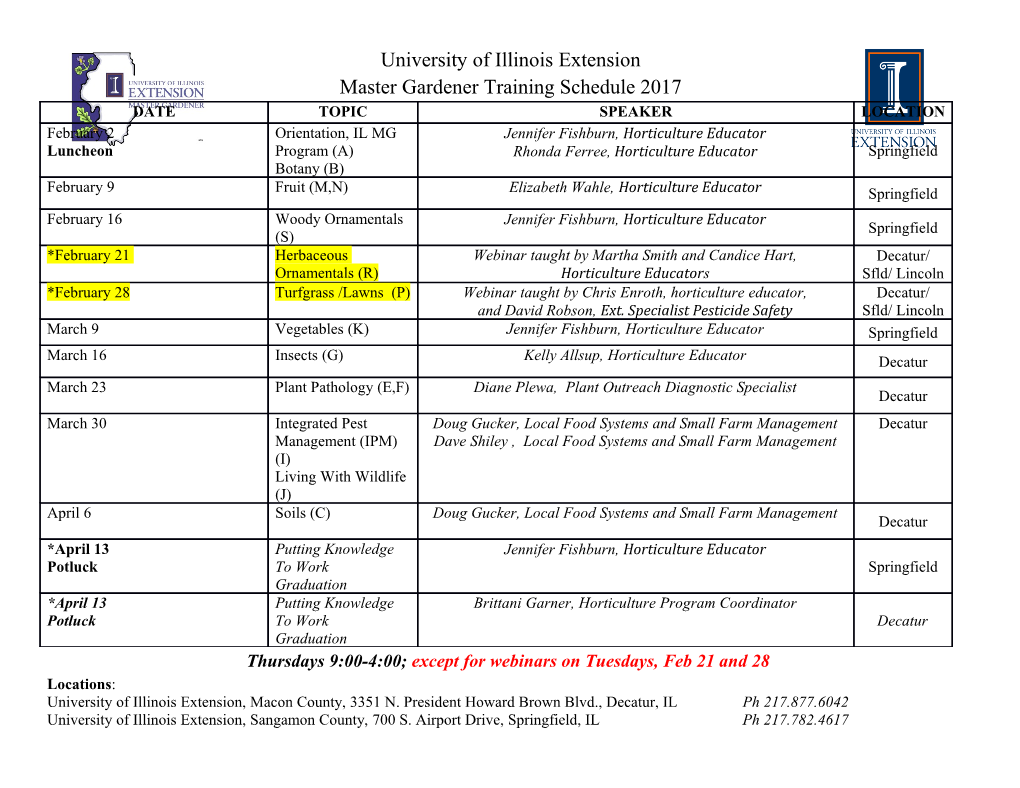
1 Bangladesh Journal of Agricultural Economics, XL 1&2 (2019): 1-16 SMALL AREA ESTIMATION OF POVERTY IN RURAL BANGLADESH Md. Farouq Imam1 Mohammad Amirul Islam1 Md. Akhtarul Alam1* Md. Jamal Hossain1 Sumonkanti Das2 ABSTRACT Poverty is a complex phenomenon and most of the developing countries are struggling to overcome the problem. Small area estimation offers help to allocate resources efficiently to address poverty at lower administrative level. This study used data from Census 2011 and Household Income and Expenditure Survey (HIES)-2010. Using ELL and M-Quantile methods, this study identified Rangpur division as the poorest one where Kurigram is the poorest district. Finally, considering both upper and lower poverty lines this study identified the poverty estimates at upazila level of Rangpur division using ELL and M-Quantile methods. The analyses found that 32% of the households were absolute poor and 19% were extremely poor in rural Bangladesh. Among the upazilas under Rangpur division Rajarhat, Ulipur, Char Rajibpur, Phulbari, Chilmari, Kurigram Sadar, Nageshwari, and Fulchhari Upazilas have been identified as the poorest upazilas. Keywords: Small area, poverty, ELL, M-Quantile methods I. INTRODUCTION Bangladesh is a developing country in the south Asia. According to the recent statistics by Bangladesh Bureau of Statistics (BBS, 2017, HIES, 2010) the per capita annual income of Bangladesh is US$1610, estimated Gross Domestic Product (GDP) is 7.28, and the percentage below the poverty line (upper) is 24.30 percent. The population is predominantly rural, with about 70 percent people living in rural areas (HIES, 2016). In Bangladesh, poverty scenario was first surveyed in 1973-1974. In this study, data were used from the Household Income and Expenditure Survey (HIES, 2010). In HIES (2010), food intake, direct calorie intake and cost of basic needs (CBN) method were used. Daily per capita 2122 kilo-calorie and 1805 kilo-calorie were considered as cut off points for absolute and hardcore poverty respectively. According to HIES (2016), head count ratio of poverty incidence using upper poverty line by CBN method from 50.1% in 1995-96 has been reduced to 24.3% in 2016. Other indices such as poverty gap index (PGI) and squared poverty gap index (SPGI) reduced at national, rural and urban levels. In terms of the upper poverty line based on calorie intake, 41.2 million in rural areas and 14.8 million in urban areas were poor in 2005. The number of hardcore poor was 18.7 1 Department of Agricultural Statistics, Bangladesh Agricultural University, Mymensingh. 2 Department of Statistics, Shahjalal University of Science and Technology, Sylhet *Corresponding author: Md. Akhtarul Alam, Department of Agricultural Statistics, Bangladesh Agricultural University, Mymensingh, Email: [email protected] 2 The Bangladesh Journal of Agricultural Economics million in rural areas and 8.3 million in urban areas in 2005 (BBS, 2005). With appropriate interventions, Bangladesh could successfully reduce the number of poor people. Using per capita consumption expenditure or CBN approach in 2016 the percentage poor in urban areas based on upper poverty line was 18.9% and that for lower poverty line was 7.6%. In the rural areas these were 26.4% and 14.9% respectively (HIES, 2016). This remarkable achievement lead Bangladesh to fulfill the MDG targets and Bangladesh has been recognized highly for such success. However, despite all efforts, rural areas are lagging behind compared to urban areas with more poor people living in rural areas. Therefore, it is necessary to accurately estimate the poverty in rural areas of Bangladesh. Furthermore, recently government has been planning to implement local area level budget, which will require small area level estimates of poverty for efficient disbursement of resources. This study aims to provide small area estimates of poverty in rural Bangladesh using latest statistical methodologies, which has not yet been practiced in Bangladesh. Small area estimation is a mathematical and statistical method, which is applied in many areas of research, e.g. environmental statistics, economics, demography, epidemiology, and so on. The estimates from these models are more accurate at small area level than using only data collected from each small area. The additional accuracy is achieved in many such models by “borrowing strength” for the estimate for a particular small area by using information from areas to which it is similar (Haslett et al. 2014). To estimate poverty direct and indirect estimation and model-based methods are used. In model-based approach, a regression model is developed based on the survey data and known auxiliary variables. These small area estimation methods can be split into two groups according to their use of implicit and explicit models (Das, 2016). Methods that use implicit models related to small areas use supplementary data from census and survey, whereas methods that use explicit models account for variability between small areas and between units in the areas through variation in the auxiliary data (Das, 2016). Poverty mapping is a method to estimate the welfare level and the degree of inequality at lower aggregation levels such as upazila or village. Geographically disaggregated level indicators provide information about the spatial distribution of inequality and poverty within a country or a large state of a country. A poverty map is a useful technique to capture the heterogeneity in poverty and inequality across different regions in a country. Based on the gradient distributions of poverty indicators and their determinants policy makers may design area-specific interventions. A poverty map also helps to allocate aid during periods of natural disaster such as flood or an earthquake (Das, 2016). Globally several projects have been conducted to estimate poverty maps based on SAE methods. The first project was conducted by the US Census Bureau to estimate Small Area Income and Poverty (Citro et al. 1997), which is the ongoing program of US Census Bureau to estimates the number of poor school-age children within states and counties each year. The World Bank initiated to develop expenditure-based SAE method (Hentschel and Lanjouw, 1996) and applied to poverty mapping (Elbers et al. 2002, 2003), which is known as the “World Bank” methodology. More than 60 countries, especially the developing countries, in the world are using this method to map poverty (Elbers and Van der Weide, 2014). To estimate poverty at a disaggregated level, the Small Area Estimation method developed by Elbers et al. (2003) which referred to as ELL, recently has been gaining popularity among development practitioners worldwide. One advantage of the ELL method is that it not only sets out to estimate poverty incidence, but also yields estimates of standard errors on the poverty estimates. Small Area Estimation of Poverty 3 The standard errors are useful about the precision and reliability of the estimates produced with the ELL methodology. Several studies have used this method (Tarozzi and Deaton, 2009; Molina and Rao, 2010; World Bank, 2016). The ELL method assumes that the only significant source of higher-level variation is between- cluster variation, which is equivalent to the assumption that it is possible to incorporate a large enough number of explanatory variables in the two-level regression model to ensure between-area variation. Therefore, the ELL method will fail to provide efficient estimates when there is non- negligible between-area variation in the distribution of the response variable (Das, 2016). Though this method is robust to departures from the assumption of normal errors and is not computationally intensive, it is not robust to the presence of outliers and is not robust to model misspecification. However, it does have the advantage that since the model is fitted at household (unit) level and then simulated, the same predicted model is used to estimate poverty indicators at any level of the population (Guadarrama et al. 2015). Tzavidis et al. (2008) proposed an M-quantile (MQ) approach to poverty mapping as an alternative to the ELL and EBP methods. Several studies have used this method (Giusti et al. 2012; Fabrizi et al. 2012). More specifically, in the study we have used a novel linear M-quantile model (Chambers and Tzavidis, 2007) for poverty estimation at small area levels and provided a poverty map for rural Bangladesh. The poverty maps provide a graphical summary of areas, which are suffering from a relatively high deprivation. The main purpose in producing such maps is to aid the planning of social interventions. Furthermore, the commonly used ELL estimates (Elbers et al. 2003) will be produced using same set of data to compare the efficiency between ELL and M-quantile estimates. Therefore, this study intends to answer the research questions; what are the reliable estimates of poverty at small area levels in rural Bangladesh? Which areas in rural Bangladesh are performing poorly in terms of poverty? To address the questions the study attempted to estimate poverty at small area levels using M-quantile method and compared with the poverty estimates by ELL method and map poverty estimates at small area levels. II. DATA AND METHODS Data This study was based on secondary data, e.g., Household Income and Expenditure Survey (HIES)- 2010 and Census-2011. It can be noted that though HIES 2016 report has been published, the complete set of data has not been released yet. Household Income and Expenditure Survey (HIES) 2010 conducted by Bangladesh Bureau of Statistics (BBS) was a two-stage stratified random sample. In the HIES 2010, a total of 12240 households were randomly selected from 7 divisions, 64 districts, and 384 sub-districts. In this study, we have used 7840 rural households in Bangladesh to identify the important factors associated with poverty in rural Bangladesh. This study used 5% Census-2011 data for small area estimation practice collected from BBS.
Details
-
File Typepdf
-
Upload Time-
-
Content LanguagesEnglish
-
Upload UserAnonymous/Not logged-in
-
File Pages16 Page
-
File Size-