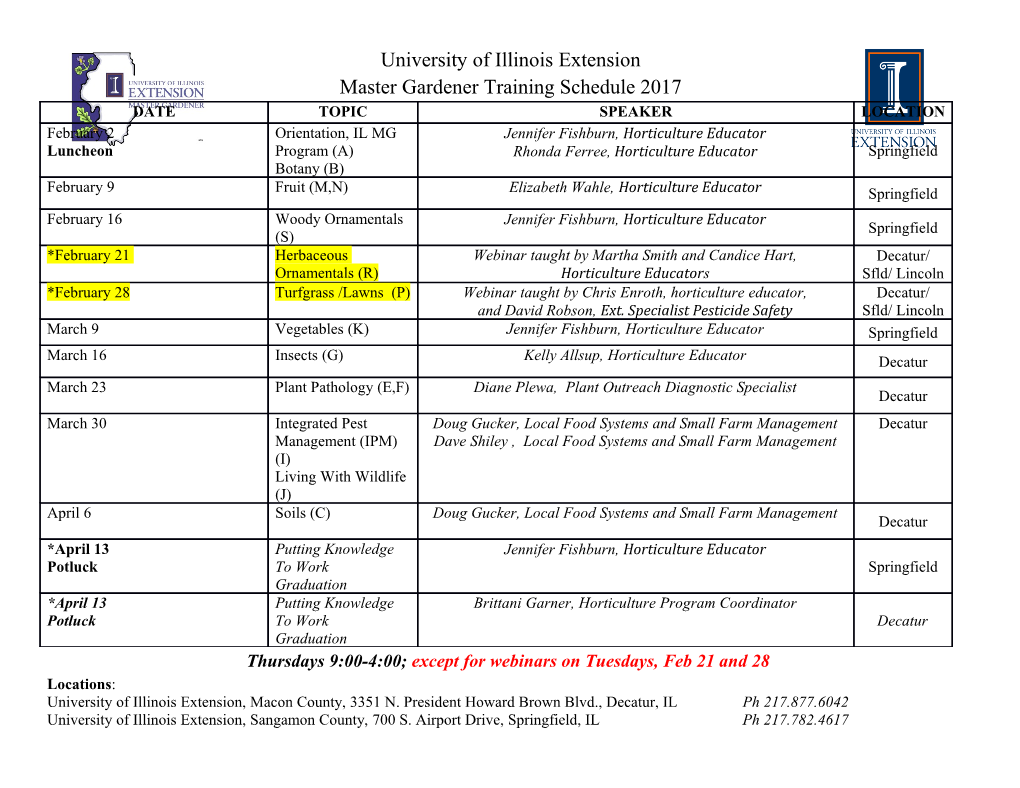
Atmos. Chem. Phys., 12, 9739–9751, 2012 www.atmos-chem-phys.net/12/9739/2012/ Atmospheric doi:10.5194/acp-12-9739-2012 Chemistry © Author(s) 2012. CC Attribution 3.0 License. and Physics Mapping the uncertainty in global CCN using emulation L. A. Lee, K. S. Carslaw, K. J. Pringle, and G. W. Mann Institute for Climate and Atmospheric Science, School of Earth and Environment, University of Leeds, UK Correspondence to: L. A. Lee ([email protected]) Received: 11 April 2012 – Published in Atmos. Chem. Phys. Discuss.: 6 June 2012 Revised: 19 September 2012 – Accepted: 1 October 2012 – Published: 25 October 2012 Abstract. In the last two IPCC assessments aerosol radia- specified precisely. When extended to all process parameters tive forcings have been given the largest uncertainty range the approach presented here will therefore provide a clear of all forcing agents assessed. This forcing range is really global picture of how improved knowledge of aerosol pro- a diversity of simulated forcings in different models. An es- cesses would translate into reduced model uncertainty. sential step towards reducing model uncertainty is to quan- tify and attribute the sources of uncertainty at the process level. Here, we use statistical emulation techniques to quan- tify uncertainty in simulated concentrations of July-mean 1 Introduction cloud condensation nuclei (CCN) from a complex global aerosol microphysics model. CCN was chosen because it is Many of the atmospheric processes that control and shape the aerosol property that controls cloud drop concentrations, the global aerosol distribution cannot be explicitly treated and therefore the aerosol indirect radiative forcing effect. We in models, either due to a lack of understanding or through use Gaussian process emulation to perform a full variance- computational constraints. Treatment of these processes in based sensitivity analysis and quantify, for each model grid models thus relies on simplified parameterisations, which of- box, the uncertainty in simulated CCN that results from 8 ten contain parameters that are not well constrained by mea- uncertain model parameters. We produce global maps of ab- surements or theory. This parametric uncertainty means that solute and relative CCN sensitivities to the 8 model param- every model simulation has some degree of uncertainty as- eter ranges and derive probability density functions for sim- sociated with it. Although one-at-a-time sensitivity tests are ulated CCN. The approach also allows us to include the un- commonly used to estimate the range of model predictions, certainty from interactions between these parameters, which these are far from adequate for estimating the associated con- cannot be quantified in traditional one-at-a-time sensitivity fidence interval around the model since no parameter interac- tests. The key findings from our analysis are that model CCN tions can be taken into account. Rather, most effort to define in polluted regions and the Southern Ocean are mostly only uncertainty has focused on multi-model inter-comparisons sensitive to uncertainties in emissions parameters but in all (Schimel et al., 1996; Penner et al., 2001; Forster et al., 2007; other regions CCN uncertainty is driven almost exclusively Textor et al., 2006, 2007; Meehl et al., 2007), which provide by uncertainties in parameters associated with model pro- an important insight into model diversity, but no estimate of cesses. For example, in marine regions between 30◦ S and the parametric uncertainty of the individual models. In our 30◦ N model CCN uncertainty is driven mainly by param- study, we focus on parameter uncertainty in a single model, eters associated with cloud-processing of Aitken-sized par- quantifying and attributing uncertainties in simulated CCN ticles whereas in polar regions uncertainties in scavenging concentrations from several uncertain model parameters. parameters dominate. In these two regions a single parame- Sensitivity analysis (SA) offers a way of quantifying ter dominates but in other regions up to 50 % of the variance model uncertainty and identifying which processes con- can be due to interaction effects between different param- tribute most to it. SA is usually carried out using stan- eters. Our analysis provides direct quantification of the re- dard “one-at-a-time” (OAT) sensitivity tests which systemat- duction in variance that would result if a parameter could be ically investigate departures of model behaviour from some baseline. However, OAT tests cannot identify and quantify Published by Copernicus Publications on behalf of the European Geosciences Union. 9740 L. A. Lee et al.: Mapping the uncertainty in global CCN using emulation interactions between parameters and they consider only ulation that can form cloud droplets. This is a key quantity a small fraction of the total parameter uncertainty space in the prediction of the aerosol indirect effect. The advantage (Saltelli and Annonia, 2010). A more comprehensive ap- of extending the analysis from point locations to the global proach is to use Monte Carlo simulations in which the sta- scale is clear: it allows the identification of regions where tistical distribution of the model output is populated by parametric uncertainty strongly affects CCN and identifies sampling thousands of possible parameter values across the the role of the different parameters in different regions. multi-dimensional parameter uncertainty space. The output distribution is then used for the sensitivity analysis, such as analysis of variance and variance decomposition to under- 2 Aerosol model description stand contributions to the overall variance. However, Monte The GLObal Model of Aerosol Processes (GLOMAP-mode) Carlo simulation requires a very large number of model sim- (Mann et al., 2010) simulates the size distribution and com- ulations, which is normally prohibitively expensive for com- position of a population of aerosol particles. The model in- plex atmospheric models. cludes new particle formation, coagulation, gas-to-particle A number of studies have been carried out to assess pa- transfer and cloud processing. GLOMAP-mode treats the rameter uncertainty in aerosol models. Pan et al. (1997) used aerosol size distribution using 7 lognormal modes (solu- probabilistic collocation to represent the computer model ble nucleation, Aitken, accumulation and coarse modes plus and carry out uncertainty analysis of the aerosol indirect ef- water-insoluble Aitken, accumulation and coarse modes for fect. Ackerley et al. (2009) used a general circulation model initially insoluble soot and dust particles). The modes de- (GCM) to study the uncertainty in parameters determining scribe the functional shape of the particle size distribution atmospheric aerosol and its effect on the climate. Haerter (particle number versus size). Such a modal model provides a et al. (2009) and Lohmann and Ferrachat (2010) used the simpler and more computationally efficient representation of ECHAM global model to study the parametric uncertainty the particle distribution than alternative sectional schemes in in aerosol and its effect on clouds but in both cases the which the particle distribution is described as a number ver- number of parameters studied was limited by the method. sus size histogram (Spracklen, 2005; Mann et al., 2012). The Haerter et al. (2009) studied the effect of perturbations of 7 model physical and chemical processes calculate the time de- cloud-related parameters on the aerosol indirect effect with pendent evolution and interaction of the number concentra- interactions identified by the differences between OAT ex- tion and size of particles within the seven modes. GLOMAP- periments and multiple parameter perturbation experiments. mode is implemented within the TOMCAT global 3-D offline Lohmann and Ferrachat (2010) used a factorial design with chemistry transport model (Chipperfield, 2006), which has a 4 parameters to examine the effect of parametric uncertainty spatial resolution of 2.81 × 2.81◦ and 31 vertical levels. The on clouds. Partridge et al. (2012) apply Markov Chain Monte model is run with the same setup as described in detail by Carlo (MCMC) methods to a cloud parcel model to inves- (Mann et al., 2010). It includes sea spray, black carbon, or- tigate cloud-aerosol sensitivity to parameter uncertainty. In ganic carbon and dust and has been shown to compare well this study we illustrate the method of emulation to make with ground based observations of aerosol mass and number statistical inferences about the relative effect of aerosol pa- (Mann et al., 2010; Spracklen et al., 2010). rameter uncertainties and their interactions on the cloud- The GLOMAP-mode aerosol model is significantly more related quantity CCN with 8 parameters using a sophisticated complex in its design than the models used in climate sim- aerosol model. This method can easily be extended to more ulations. The main difference is that GLOMAP-mode sim- model parameters and outputs as well as different models. ulates the evolution of the aerosol size distribution, while We use emulation to carry out a parametric sensitivity climate models typically simulate only the masses of the analysis of a global aerosol model. An emulator is a statis- aerosol chemical components or assume a fixed size distri- tical interpolator which takes the output of model simula- bution. Thus, GLOMAP-mode requires many more param- tions spread throughout the parameter uncertainty space and eters to describe the particle distribution as well as param- estimates
Details
-
File Typepdf
-
Upload Time-
-
Content LanguagesEnglish
-
Upload UserAnonymous/Not logged-in
-
File Pages13 Page
-
File Size-