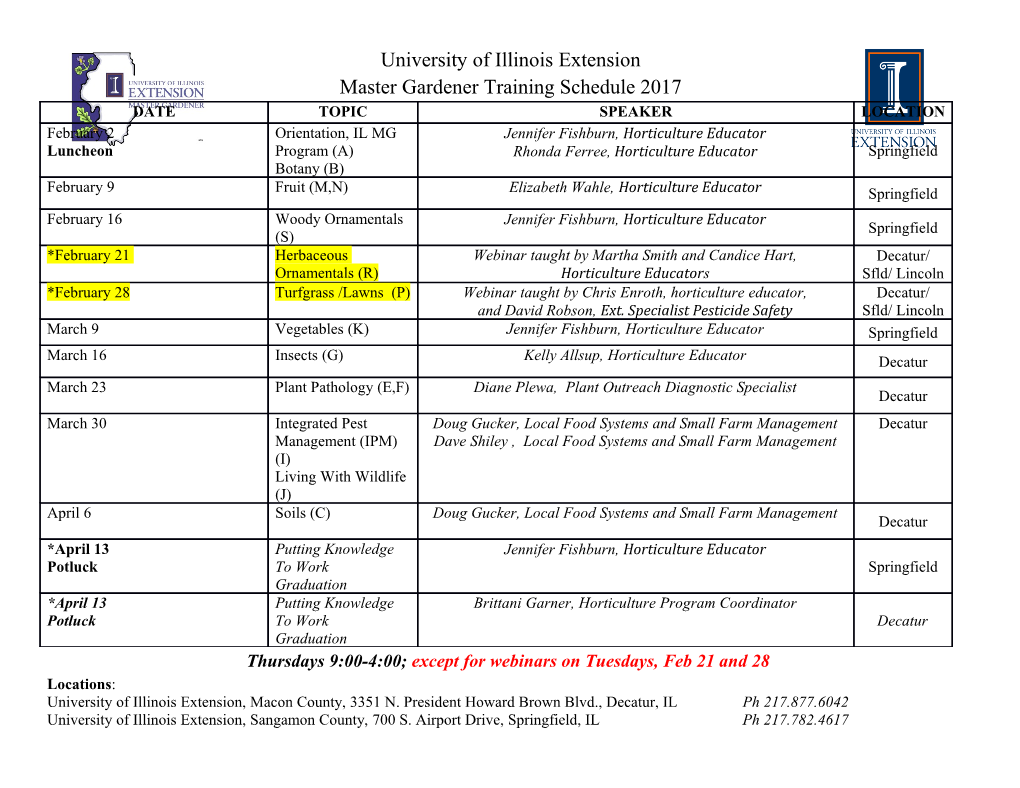
Case-control studies • Overview of different types of studies • Review of general procedures • Sampling of controls – implications for measures of association – implications for bias • Logistic regression modeling Learning Objectives • To understand how the type of control sampling relates to the measures of association that can be estimated • To understand the differences between the nested case-control study and the case-cohort design and the advantages and disadvantages of these designs • To understand the basic procedures for logistic regression modeling Overview of types of case-control studies No designated cohort, Within a designated cohort but should treat source population as cohort Nested case control Case-cohort Cases only Cases and controls 1) Type of sampling - incidence density Case-crossover - cumulative “epidemic” 2) Source of controls - population-based - hospital - neighborhood - friends -family 1 Review of General Procedures Obtaining cases Obtaining controls 1) Define target cases 1) Define target controls 2) Identify potential cases 2) Define mechanism for 3) Confirm diagnosis identifying controls 4) *Obtain physician’s consent 3) Contact control 5) Contact case 4) Confirm control’s eligibility 6) Confirm case’s eligibility 5) *Obtain control’s consent 7) *Obtain case’s consent 6) Obtain exposure data 8) Obtain exposure data *need to account for nonresponse Pros/Cons of Different Methods Collection of information In-person Telephone Mail - hospital - clinic - home Case cohort study T0 Additional cases, Assemble compare all cases to the cohort, sample First case subcohort selected subcohort to be at T0 used in all future analyses 2 Nested case-control Study T0 Assemble Likewise, select controls cohort again at each separate time point; First case; note: each of these randomly cases was eligible to be a control for the select control first case from remaining cohort Crossover designs Period the subject is exposed Period the subject is unexposed • Case-crossover: variation of crossover; case has a pre-disease period which is used as the control period • Good method for control of confounding • May have limited applications • Assumes that neither exposure nor confounders are changing over time in a systematic way Source Population • Think of all case-control studies as nested within a cohort, even when the cohort is not designated • Source population is this underlying cohort • Source population describes the cohort giving rise to the cases; controls are also from this source population • Source population reflects the disease under study, difficulties in diagnosing disease, routine procedures for recording the disease occurrence, and the frequency of disease 3 Classic case-control Source population Cases Controls Sampling fraction (f1) Sampling fraction f2 Exposed Non-exposed Exposed Nonexposed (A) (C) (B) (D) Cases Controls Odds ratio = AD/BC Exposed A B If sampling: OR = f1*A*f2*D = AD Unexposed C D f1*C*f2*B BC Incidence Density Sampling 1) Collect information on each case 2) Collect control at the same time each case is observed; collect control from the underlying source population giving rise to the cases 3) Controls can be cases Cumulative-based sampling 1) Start after the event has happened 2) Ascertain cases 3) Collect controls from the noncases after event (e.g., epidemic) 4 Measures of Association • Key concept, sampling fraction, independent of exposure • Incidence density sampling/nested case-control studies – if exposure odds in controls(B/D) approximates person-time ratio for source population, odds ratio will approximate rate ratio – without rare disease assumption Measures of Association • Case cohort – if ratio B/D approximates overall prevalence of exposure in source population, odds ratio will approximate risk ratio – without rare disease assumption • Cumulative “Epidemic” case-control studies – odds ratio will approximate rate ratio if proportion diseased in each exposure group is low (< 20%) and remains steady during study period When is the rare disease assumption needed? • Cumulative-based sampling if want to approximate the relative risk • Otherwise – nested case-control and incidence density sampling will give an estimate of rate ratio without rare disease assumption – case-cohort will give an estimate of risk ratio without rare disease assumption • If disease is rare, all three measures will be very close 5 A closer look Recall: Note: in R&G notation A1 I T A T A1= A 1 1 1 o IR = = = Ao= C I A0 A T 0 0 1 B1= B T0 B0= D A1 A1 (1− A ) B A B OR = 1 = 1 = 1 o A A 0 0 A0 B1 (1− A0 ) B0 If sampling does not lead to bias and the sample can approximate the person-years of distribution, a case- control study is a more efficient design (i.e. for same number of people, more precision). However not as precise as if you use the full cohort. Pseudo Rates B B This assumes that the sampling f = 1 = 0 = r 2 T T rates are the same for the 1 0 exposed and unexposed A1 Pseudo-rate1 = B1 A0 if r is unknown : Pseudo-rate0 = B 0 A1 T1 A1 1 A1 A1 * If r is known then B B T T r T 1 = 1 1 = 1 = 1 multiplyby r to get rate A0 A T A0 1 A0 0 0 * B0 T r T A1 A1 B1 B0 T0 o o *r = = I1 B1 B1 T1 Pseudo Risks This assumes that the sampling B1 B0 rates are the same for the exposed f2 = = = f N1 N0 and unexposed A Pseudo- risk = 1 1 If f is unknown : B1 A0 A1 N1 A1 1 A1 A1 Pseudo- risk0 = * B B B N N f N 0 1 = 1 1 = 1 = 1 A0 A N A0 1 A0 If f is known then multiply 0 0 * B0 N f N by f to get incidence proportion B0 N0 0 0 A1 A1 B1 * f = = R1 B1 B1 N1 6 Summary • Odds Ratio • Odds Ratio approximates Rate approximates Risk Ratio Ratio – incidence density sampling – case-cohort design – nested case- control studies – cumulative – cumulative sampling if sampling is disease is rare proportion exposed is steady and relatively low Source of Controls population-based: not necessarily equal probability, control selection probability is proportional to the individual’s person time at risk neighborhood: need to think about referral patterns (e.g., not good for veteran’s hosp); also need to worry about overmatching hospital: need to be especially concerned that sampling was independent of exposure; try to use a variety of diagnoses for controls. friends: main problem is with overmatching; cedes the control selection to the case rather than to the investigator family:may be worthwhile design to control for certain variable (e.g., spouse control, environment, twin control genetics and environment) Review of control selection • Select from source population • Select independent of exposure status • Probability of selection should be proportion to amount s/he would have contributed to person-time in the denominator • Not eligible to be a control, if during same time would not have been eligible to be a case 7 Ille-et-Vilaine study (epidat1.txt) • conducted between January 1972 and April 1974 • French department of Ille-et-Vilaine (Brittany) • Men diagnosed in regional hospitals • Controls sampled from electoral lists in each commune of department Other methodological points • exposure opportunity – interest is in the fact of exposure – think about cohort design – make same exclusions to cases as to controls • comparability of information – may not want comparability if errors are not independent • number of different types of control groups – may be appropriate in some situations (e.g., spouse, siblings), but generally not recommended • prior disease history Regression Modeling Regression model- simpler function used to estimate the true regression function Benefit of Regression Modeling: overcome the numerical limitations of categorical analysis Cost of Regression Modeling: assumptions of model; invalid inferences if model is misspecified E(Y|X = x) Y = dependent variable, outcome variable, regressand X = set of independent variables, predictors, covariates, regressors 8 Logistic Regression Y When x = 1, log = α + β x 1−Y eα +β x α +β x α +β x e Y 1+ e α +β x Y = = R(x1) = = e α +β x 1−Y 1+ eα +β x eα +β x 1+ e − When x = 0, 1+ eα +β x 1+ eα +β x eα Y log | x =1 α +β x Y α 1−Y e = 1+ e = eα = log = β 1−Y 1+ eα eα Y eα − log | x = 0 1+ eα 1+ eα 1−Y R(x1) Logistic risk model, bounded by 0 and 1 Likelihood in logistic regression α+∑ β x α+∑ β x e i i e i i (1− ) ∏ α+∑ βixi ∏ α+∑ βixi 1+ e 1+ e Cases Controls Interpreting Coefficients Y log( ) = α + β x 1−Y if X = 1 if exposed, 0 if unexposed Y log( ) | x =1 α + β 1−Y = = β Y log( ) | x = 0 α 1−Y OR = eβ If X is continuous, B represents the impact of a one-unit change in X to Y, the exponential of B will give you the OR for a one unit change Note: both modeling variables as either ordered categorical variables or continuous variables assumes the one unit change in X is the same irrespective of the level of X 9 Logistic Modeling Linear Model Logistic Model Log ( Y) Y $ (1-Y) $ " " Not bounded by 0 and 1 Y = Probability of Disease Y = Odds of Disease 1- Y Y log( ) = Log odds of Disease 1- Y Assessing Linearity • Create categories of continuous variable; categories should represent the same amount of units (e.g., 0-39 grams, 40-79 grams, 80-119, etc) • Plot Beta coefficients; if pattern is approximately linear, keep variable as a continuous variable in model Logistic Model Log ( Y) * * (1-Y) * * * * * * $1 $2 $3 $4 10 Creating Indicator Variables Also referred to as categorical regressors, dummy variables Need to pick a reference level Number of indicator variables created = # categories - 1 The intercept
Details
-
File Typepdf
-
Upload Time-
-
Content LanguagesEnglish
-
Upload UserAnonymous/Not logged-in
-
File Pages13 Page
-
File Size-