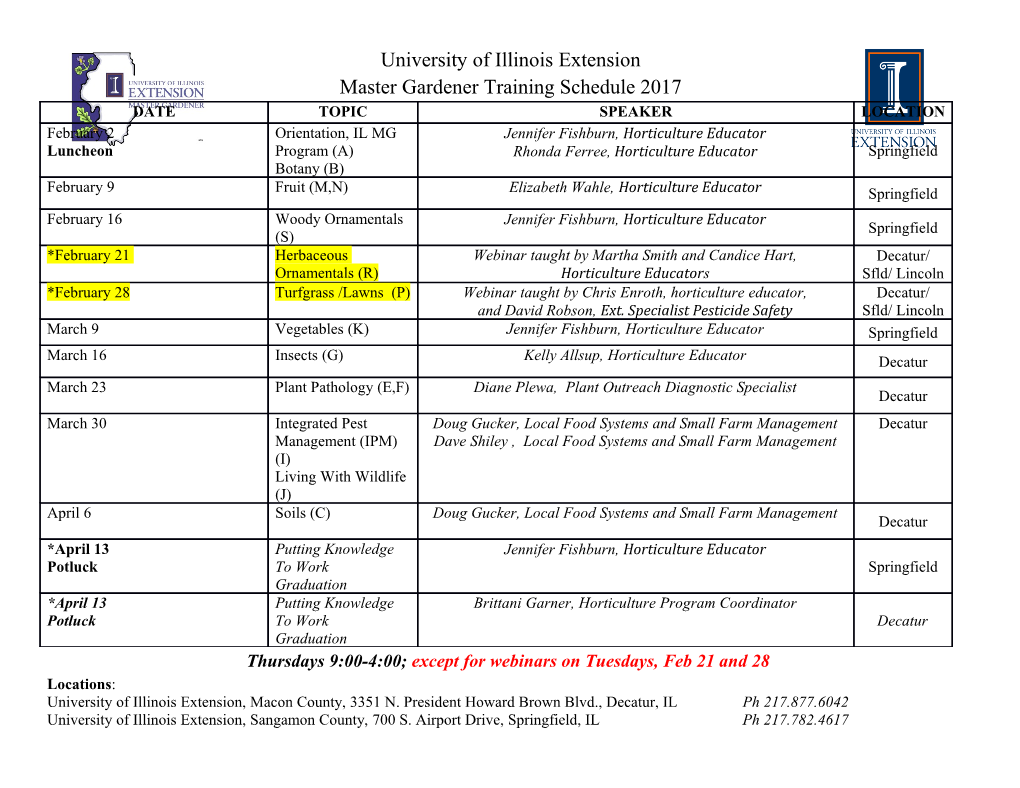
Online Ad Serving: Theory and Practice Aranyak Mehta Vahab Mirrokni June 7, 2011 Outline of this talk I Ad delivery for contract-based settings I Ad Serving I Planning I Ad serving in repeated auction settings I General architecture. I Allocation for budget constrained advertisers. I Other interactions I Learning + allocation I Learning + auction I Auction + contracts Contract-based Ad Delivery: Outline I Basic Information I Ad Serving. I Targeting. I Online Allocation I Ad Planning: Reservation I Cost-Per-Impression (CPM). I Not Auction-based: offline negotiations + Online allocations. Display/Banner Ads: I Q1, 2010: One Trillion Display Ads in US. $2:7 billion. I Top Advertiser: AT&T, Verizon, Scottrade. I Ad Serving Systems e.g. Facebook, Google Doubleclick, AdMob. Contract-based Online Advertising I Pageviews (impressions) instead of queries. I Display/Banner Ads, Video Ads, Mobile Ads. Display/Banner Ads: I Q1, 2010: One Trillion Display Ads in US. $2:7 billion. I Top Advertiser: AT&T, Verizon, Scottrade. I Ad Serving Systems e.g. Facebook, Google Doubleclick, AdMob. Contract-based Online Advertising I Pageviews (impressions) instead of queries. I Display/Banner Ads, Video Ads, Mobile Ads. I Cost-Per-Impression (CPM). I Not Auction-based: offline negotiations + Online allocations. I Ad Serving Systems e.g. Facebook, Google Doubleclick, AdMob. Contract-based Online Advertising I Pageviews (impressions) instead of queries. I Display/Banner Ads, Video Ads, Mobile Ads. I Cost-Per-Impression (CPM). I Not Auction-based: offline negotiations + Online allocations. Display/Banner Ads: I Q1, 2010: One Trillion Display Ads in US. $2:7 billion. I Top Advertiser: AT&T, Verizon, Scottrade. Contract-based Online Advertising I Pageviews (impressions) instead of queries. I Display/Banner Ads, Video Ads, Mobile Ads. I Cost-Per-Impression (CPM). I Not Auction-based: offline negotiations + Online allocations. Display/Banner Ads: I Q1, 2010: One Trillion Display Ads in US. $2:7 billion. I Top Advertiser: AT&T, Verizon, Scottrade. I Ad Serving Systems e.g. Facebook, Google Doubleclick, AdMob. Internet Ad Revenues - 2010 Internet Advertising Revenues - 2010 Other 6% Search Classified 46% 10% Display 38% 24% increase Total $26.0 billion I Objective Functions: I Efficiency: Users and Advertisers. Revenue of the Publisher. I Smoothness, Fairness, Delivery Penalty. Display Ad Delivery: Overview Display Ad Delivery Planning: Ad Serving: Targeting: Allocation: 1. Planning: Contracts/Commitments with Advertisers. 2. Ad Serving: I Targeting: Predicting value of impressions. I Ad Allocation: Assigning Impressions to Ads Online. I Objective Functions: I Efficiency: Users and Advertisers. Revenue of the Publisher. I Smoothness, Fairness, Delivery Penalty. Display Ad Delivery: Overview Display Ad Delivery Planning: Offline, Online Strategic, Stochastic Ad Serving: Targeting: Allocation: Online, Stochastic 1. Planning: Contracts/Commitments with Advertisers. 2. Ad Serving: I Targeting: Predicting value of impressions. I Ad Allocation: Assigning Impressions to Ads Online. I Objective Functions: I Efficiency: Users and Advertisers. Revenue of the Publisher. I Smoothness, Fairness, Delivery Penalty. Display Ad Delivery: Overview Display Ad Delivery Planning: Offline, Online Forecasting Strategic, Stochastic Supply of impressions Demand for ads Ad Serving: Targeting: Allocation: Online, Stochastic 1. Planning: Contracts/Commitments with Advertisers. 2. Ad Serving: I Targeting: Predicting value of impressions. I Ad Allocation: Assigning Impressions to Ads Online. I Objective Functions: I Efficiency: Users and Advertisers. Revenue of the Publisher. I Smoothness, Fairness, Delivery Penalty. Display Ad Delivery: Overview Display Ad Delivery Planning: Delivery Constraints, Budget Offline, Online Forecasting Strategic, Stochastic Supply of impressions Demand for ads Ad Serving: Targeting: Allocation: Online, Stochastic 1. Planning: Contracts/Commitments with Advertisers. 2. Ad Serving: I Targeting: Predicting value of impressions. I Ad Allocation: Assigning Impressions to Ads Online. I Objective Functions: I Efficiency: Users and Advertisers. Revenue of the Publisher. I Smoothness, Fairness, Delivery Penalty. Display Ad Delivery: Overview Display Ad Delivery Planning: Delivery Constraints, Budget Offline, Online Forecasting Strategic, Stochastic Supply of impressions Demand for ads Ad Serving: Targeting: CTR Allocation: Online, Stochastic 1. Planning: Contracts/Commitments with Advertisers. 2. Ad Serving: I Targeting: Predicting value of impressions. I Ad Allocation: Assigning Impressions to Ads Online. Display Ad Delivery: Overview Display Ad Delivery Planning: Delivery Constraints, Budget Offline, Online Forecasting Strategic, Stochastic Supply of impressions Demand for ads Ad Serving: Targeting: CTR Allocation: Online, Stochastic I Objective Functions: I Efficiency: Users and Advertisers. Revenue of the Publisher. I Smoothness, Fairness, Delivery Penalty. Contract-based Ad Delivery: Outline I Basic Information I Ad Serving. I Targeting. I Online Ad Allocation I Ad Planning: Reservation I Behavioral Targeting I Interest-based Advertising. I Yan, Liu, Wang, Zhang, Jiang, Chen, 2009, How much can Behavioral Targeting Help Online Advertising? I Contextual Targeting I Information Retrieval (IR). I Broder, Fontoura, Josifovski, Riedel, A semantic approach to contextual advertising I Creative Optimization I Experimentation Targeting Estimating Value of an impression. I Contextual Targeting I Information Retrieval (IR). I Broder, Fontoura, Josifovski, Riedel, A semantic approach to contextual advertising I Creative Optimization I Experimentation Targeting Estimating Value of an impression. I Behavioral Targeting I Interest-based Advertising. I Yan, Liu, Wang, Zhang, Jiang, Chen, 2009, How much can Behavioral Targeting Help Online Advertising? I Creative Optimization I Experimentation Targeting Estimating Value of an impression. I Behavioral Targeting I Interest-based Advertising. I Yan, Liu, Wang, Zhang, Jiang, Chen, 2009, How much can Behavioral Targeting Help Online Advertising? I Contextual Targeting I Information Retrieval (IR). I Broder, Fontoura, Josifovski, Riedel, A semantic approach to contextual advertising Targeting Estimating Value of an impression. I Behavioral Targeting I Interest-based Advertising. I Yan, Liu, Wang, Zhang, Jiang, Chen, 2009, How much can Behavioral Targeting Help Online Advertising? I Contextual Targeting I Information Retrieval (IR). I Broder, Fontoura, Josifovski, Riedel, A semantic approach to contextual advertising I Creative Optimization I Experimentation I Long-term vs. Short-term value of display ads? I Archak, Mirrokni, Muthukrishnan, 2010 Graph-based Models. I Computing Adfactors based on AdGraphs I Markov Models for Advertiser-specific User Behavior Predicting value of Impressions for Display Ads I Estimating Click-Through-Rate (CTR). I Budgeted Multi-armed Bandit I Probability of Conversion. Predicting value of Impressions for Display Ads I Estimating Click-Through-Rate (CTR). I Budgeted Multi-armed Bandit I Probability of Conversion. I Long-term vs. Short-term value of display ads? I Archak, Mirrokni, Muthukrishnan, 2010 Graph-based Models. I Computing Adfactors based on AdGraphs I Markov Models for Advertiser-specific User Behavior Contract-based Ad Delivery: Outline I Basic Information I Ad Planning: Reservation I Ad Serving. I Targeting. I Online Ad Allocation Outline: Online Allocation I Online Stochastic Assignment Problems I Online (Stochastic) Matching I Online Stochastic Packing I Online Generalized Assignment (with free disposal) I Experimental Results I Online Learning and Allocation I Display Ads (DA) problem: P I Maximize value of ads served: max viaxia P i;a I Capacity of ad a: i2A(a) xia ≤ Ca Online Ad Allocation I When page arrives, assign an eligible ad. I value of assigning page i to ad a: via Online Ad Allocation I When page arrives, assign an eligible ad. I value of assigning page i to ad a: via I Display Ads (DA) problem: P I Maximize value of ads served: max viaxia P i;a I Capacity of ad a: i2A(a) xia ≤ Ca Online Ad Allocation I When page arrives, assign an eligible ad. I revenue from assigning page i to ad a: bia I \AdWords" (AW) problem: P I Maximize revenue of ads served: max biaxia P i;a I Budget of ad a: i2A(a) biaxia ≤ Ba 1 Greedy: 2 , [MSVV,BJN]: Worst-Case 1 1 [KVV]: 1− e -aprx 1− e -aprx General Form of LP X max viaxia i;a X xia ≤ 1 (8 i) a X siaxia ≤ Ca (8 a) i xia ≥ 0 (8 i; a) Online Matching: Disp. Ads (DA): AdWords (AW): via = sia = 1 sia = 1 sia = via General Form of LP X max viaxia i;a X xia ≤ 1 (8 i) a X siaxia ≤ Ca (8 a) i xia ≥ 0 (8 i; a) Online Matching: Disp. Ads (DA): AdWords (AW): via = sia = 1 sia = 1 sia = via 1 Greedy: 2 , [MSVV,BJN]: Worst-Case 1 1 [KVV]: 1− e -aprx 1− e -aprx [FMMM09,MOS11]: 0.702-aprx i.i.d with known distribution [DH09]: Stochastic ? 1−-aprx, (i.i.d.) if opt max via Stochastic i.i.d model: I i.i.d model with known distribution I random order model (i.i.d model with unknown distribution) Ad Allocation: Problems and Models Online Matching: Disp. Ads (DA): AdWords (AW): via = sia = 1 sia = 1 sia = via 1 Greedy: 2 , [MSVV,BJN]: Worst Case 1 ? 1 [KVV]: 1− e -aprx 1− e -aprx [FMMM09,MOS11]: 0.702-aprx i.i.d with known distribution Ad Allocation: Problems and Models Online Matching: Disp. Ads (DA): AdWords (AW): via = sia = 1 sia = 1 sia = via 1 Greedy: 2 , [MSVV,BJN]: Worst Case 1 ? 1 [KVV]: 1− e -aprx 1− e -aprx [DH09]: Stochastic ? 1−-aprx, (i.i.d.) if opt max via Stochastic i.i.d model: I i.i.d model with known distribution I random order model (i.i.d model with unknown distribution) Ad Allocation: Problems and Models Online Matching: Disp. Ads (DA): AdWords (AW): via = sia = 1 sia = 1 sia = via 1 Greedy: 2 , [MSVV,BJN]: Worst Case 1 ? 1 [KVV]: 1− e -aprx 1− e -aprx [FMMM09,MOS11]: [DH09]: Stochastic 0.702-aprx ? 1−-aprx, (i.i.d.) i.i.d with known if distribution opt max via Stochastic i.i.d model: I i.i.d model with known distribution I random order model (i.i.d model with unknown distribution) Ad Allocation: Problems and Models Online Matching: Disp.
Details
-
File Typepdf
-
Upload Time-
-
Content LanguagesEnglish
-
Upload UserAnonymous/Not logged-in
-
File Pages139 Page
-
File Size-