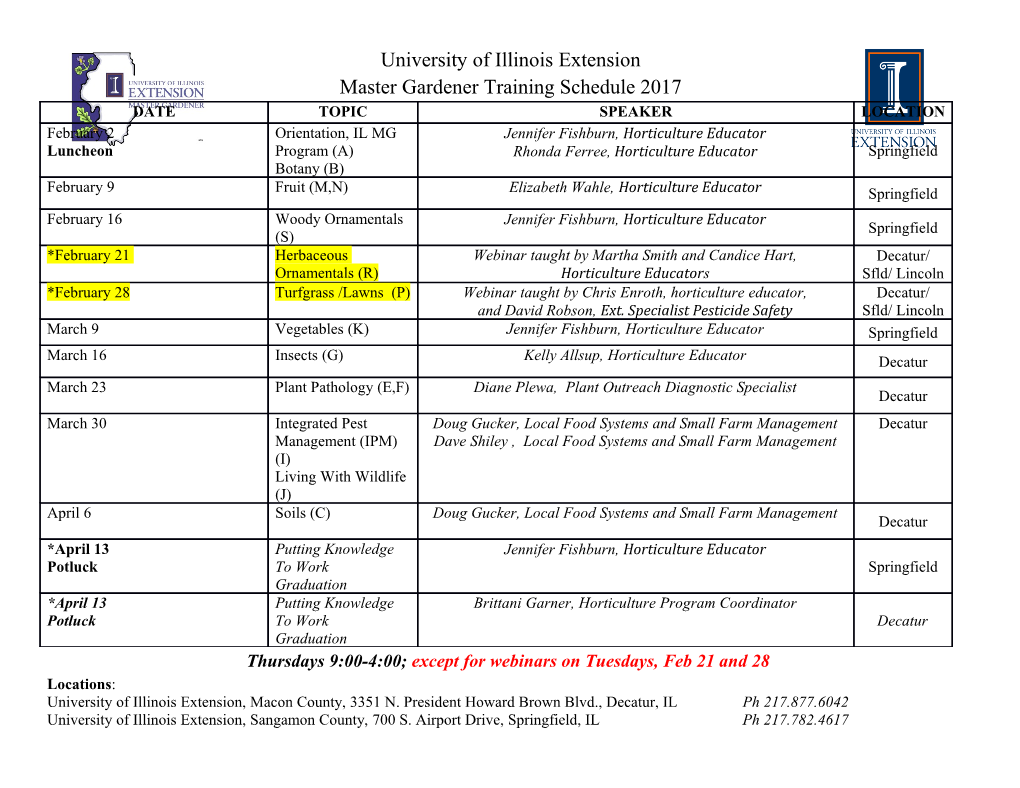
Sea ice modelling Danny Feltham Centre for Polar Observation and Modelling University of Reading UK Sea ice models Sea ice models are formulated as continuum expressions of local balances of momentum, mass, and heat, which are mediated through various processes. In practise, sea ice processes are divided into: • Dynamic processes, which control the motion of ice cover, deformation, and redistribution of thickness. Example processes are air and ocean drag, ridging, sliding, and rupture (rheology). • Thermodynamic processes, which control melting, freezing, and dissolving. Example processes are thermal conduction, brine convection, and solar radiation absorption. • Dynamic and thermodynamic processes are typically closely connected. • Many of these processes are represented in models with parameterisations, simplified mathematical representations designed to capture their major features and work within the technical constraints of the models. A sea ice lead, formed in divergence, results in rapid new ice growth. An ice cover in decline Ice is thinning Ice extent is decreasing 4.0 8 1958 - 1976 1993 - 1997 3.5 2003 - 2007 7 3.0 2.5 ) 2 6 km 6 2.0 5 1.5 1.0 Ice extent (10 Average ice thickness (m) 4 0.5 0.0 3 1978 1983 1988 1993 1998 2003 2008 2013 A B C D E F G Particularly around the periphery Ice is getting younger Alaska Future sea ice modelling needs – but what for? • Climate prediction - Coupled models with spin up period Models must account for future conditions. - Low resolution (but improving) Hitherto marginal processes may assume - Computational constraints primacy, e.g. wave breaking of ice. - Data assimilation not possible - Variability: predict statistics of ice cover and not actual ice cover • Ice “weather” prediction - Forced sea ice models Importance of local processes, e.g. propagation - High resolutions possible/needed of particular cracks, location of MIZ edge. Continuity assumption in danger. - Memory of initial conditions Discrete element modelling? - Data assimilation • Hindcasts - Sea ice/ocean model forced with reanalysis - Understanding proximate causes of change - More stringent test of simulations Accurate representation of important processes - Data assimilation of the past. Filling in missing data, e.g. PIOMAS. Resolution versus realism Sea ice models are not grid-scale independent. They do not resolve individual floes or leads and represent their “average” response. The fundamental assumption of continuity breaks down if the grid resolution approaches the floe scale. This cell only contains one complete floe. A continuum model Finer spatial prediction will not resolution be valid for this floe. 100 km 5 km Parameterisations of processes such as ridging, sliding and air/ocean drag break down as the grid resolution approaches the floe scale. Parameterisations of vertical heat transfer, melt ponds etc break down at finer resolutions. Increasing the resolution of a sea ice model will necessarily result in fine scale structure such as cracks, and this may look plausible and impressive. Be skeptical! This is NOT an argument against using higher resolution, which, in particular, is very important for the ocean simulations (and hence sea ice). CryoSat operational polar monitoring http://www.cpom.ucl.ac.uk/ csopr/seaice.html 5km resolution, 2 day thickness product (not summer months) Derived from radar satellite altimetry An unused product for model initialisation and data assimilation Essential for accurate short term ice prediction A unique CPOM (UK) contribution. Less ice, thinner ice, younger ice – new physics? YES! • Physics is inviolate and unsullied by our Earthly realm • …but which physics we choose to include in models is a human, expedient endeavor influenced by our objectives, observations, understanding, financial or computational budget, complexity, fashion, etc. • Not to mention model tuning… Some emerging areas of sea ice physics relevant to YOPP • Wave-ice interaction and the Floe Size Distribution • High resolution sea ice mechanics, e.g. elastic-plastic models, anisotropy • Inertial ice-ocean coupling • Form drag and its role in Arctic Ocean spin up • Melt ponds on sea ice Melt ponding of sea ice • Melt ponds strengthen the albedo feedback mechanism. • Melt ponds on the summer sea ice surface have increased in recent decades as shown in observations [Roesel et al, 2012] and simulated in models e.g. Flocco et al [2012]. • Physical melt pond models are now being included into climate models [Flocco et al, 2012]. 2012 2000s 1990s 1980s 1996 Melt ponding of sea ice • Melt ponds strengthen the albedo feedback mechanism. • Melt ponds on the summer sea ice surface have increased in recent decades as shown in observations [Roesel et al, 2012] and simulated in models e.g. Flocco et al [2012]. • Physical melt pond models are now being included into climate models [Flocco et al, 2012]. 8 • Pond fraction has been used to skilfully predict 2 ^ m 7 k the summer sea ice minima [Schroeder et al, n o i l l 2014] 3 months in advance, moderated by i M n atmospheric chaos [Serreze and Stroeve, 2015]. i t 6 n e t x • Ongoing work [Schroeder et al] is demonstrating e e c i the utility of melt ponds in coupled climate r 5 e b model predictions. m e t p e • Further work on the surface impact of melt S 4 SSM/I ponds is warranted, e.g. the role of topography f(pond56) hindcast f(pond56) forecast in determining pond fraction, accurate timing of pond formation. 1980 1990 2000 2010 Year g1 GCM-compatible Ice thickness distribution melt pond model g2 Fractional g 3 g Area g 5 gi 1 Sea ice topography 4 Thickness Height and depth distribution functions hsl α Reference height β Flocco and Feltham, JGR, 2007 Flocco et al., JGR, 2010, 2012 Annual melt pond cycle from CICE stand-alone simulation 15 ice thickness categories NOTE: DE-TRENDED time series Schröder, Feltham, Flocco, and Tsamados [2014] Forecast on June 25th: Error 2 σferr = 0.44 Mill. km Skill value S = 0.41 Positive feedback mechanism Pre-conditioning of sea ice HadGEM3 simulations UM-8.6 NEMO-3.4 CICE-5.0 30 years Control Run 35 years Melt pond Run constant radiative forcing for year 1985, 2010, and 2035 0.25 x 0.25 deg ORCA-grid for NEMO + CICE 1.25 x 1.875 deg grid for UM 1985 forcing Ice Extent Ice Volume 1985 forcing Ice Extent Anomaly of September ice extent in in % ice of September extent Anomaly Anomaly of mean pond fraction in % Pond fraction Summary remarks • There is stake-holder interest in sub-daily to seasonal sea ice forecasts • Data assimilation and high resolution are important for ice weather forecasting • Existing sea ice models have been built for climate-type modelling: high spatial and temporal resolution demands attention to first principles • Particular strengths of the UK lie in sea ice thickness observation from satellite data and sea ice physics modelling • Focus on seasonal predictability has pull-through to climate projections Antarctic Bottom Water (AABW) formation is the most prevalent water mass on Earth. Questions? The formation of AABW is intimately connected with the ice cover. Towards a discrete element model? The good: • Discrete element models resolve individual floes and so the continuum assumption is not invalidated • Emergent properties such as the continuum scale rheology are calculated from the aggregate behaviour of assemblies of floes, e.g. Hopkins and Hibler 1991, Wilchinsky et al 2011 • Uncertainties in the physics, e.g. of mechanical failure, are moved from the continuum scale to the floe scale, which is a scale more amenable to field observations, e.g. Coon et al, 1998, Taylor et al 2006. Towards a discrete element model? The good: • Discrete element models resolve individual floes and so the continuum assumption is not invalidated • Emergent properties such as the continuum scale rheology are calculated from the aggregate behaviour of assemblies of floes, e.g. Hopkins and Hibler 1991, Wilchinsky et al 2011 • Uncertainties in the physics, e.g. of mechanical failure, are moved from the continuum scale to the floe scale, which is a scale more amenable to field observations, e.g. Coon et al, 1998, Taylor et al 2006. The bad: • Floes are not atoms. They are not indivisible: they will fragment and freeze together. Floe properties can be modelled with standard continuum theory, e.g. flexure, thermal conduction, melt ponds… • How does a discrete element model deal with the freezing of open ocean? Towards a discrete element model? The good: • Discrete element models resolve individual floes and so the continuum assumption is not invalidated • Emergent properties such as the continuum scale rheology are calculated from the aggregate behaviour of assemblies of floes, e.g. Hopkins and Hibler 1991, Wilchinsky et al 2011 • Uncertainties in the physics, e.g. of mechanical failure, are moved from the continuum scale to the floe scale, which is a scale more amenable to field observations, e.g. Coon et al, 1998, Taylor et al 2006. The bad: • Floes are not atoms. They are not indivisible: they will fragment and freeze together. Floe properties can be modelled with standard continuum theory, e.g. flexure, thermal conduction, melt ponds… • How does a discrete element model deal with the freezing of open ocean? And the ugly: • Discrete element models are computationally demanding and new numerical techniques are needed for O(1) deformations. • Because of this they are not (currently, ever?) suitable for climate modelling .
Details
-
File Typepdf
-
Upload Time-
-
Content LanguagesEnglish
-
Upload UserAnonymous/Not logged-in
-
File Pages25 Page
-
File Size-