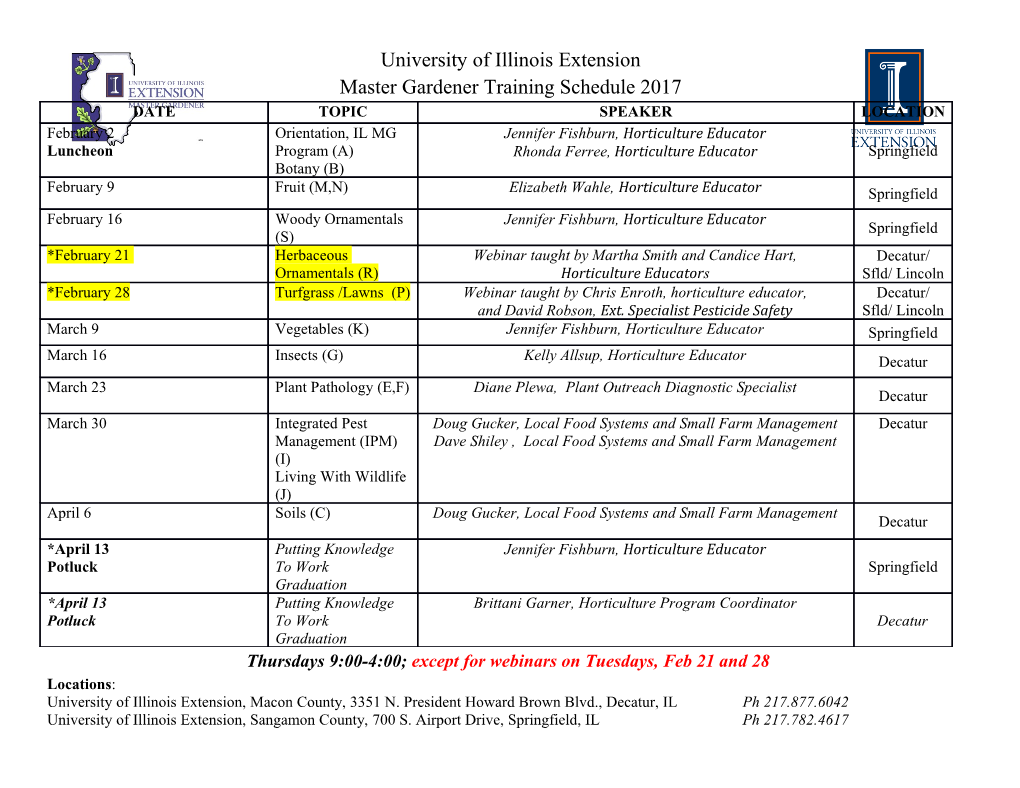
Open Access Library Journal 2020, Volume 7, e6459 ISSN Online: 2333-9721 ISSN Print: 2333-9705 Hybrid Whale Optimization Algorithm with Modified Conjugate Gradient Method to Solve Global Optimization Problems Layth Riyadh Khaleel, Ban Ahmed Mitras Department of Mathematics, College of Computer Sciences & Mathematics, Mosul University, Mosul, Iraq How to cite this paper: Khaleel, L.R. and Abstract Mitras, B.A. (2020) Hybrid Whale Optimi- zation Algorithm with Modified Conjugate Whale Optimization Algorithm (WOA) is a meta-heuristic algorithm. It is a Gradient Method to Solve Global Opti- new algorithm, it simulates the behavior of Humpback Whales in their search mization Problems. Open Access Library for food and migration. In this paper, a modified conjugate gradient algo- Journal, 7: e6459. https://doi.org/10.4236/oalib.1106459 rithm is proposed by deriving new conjugate coefficient. The sufficient des- cent and the global convergence properties for the proposed algorithm proved. Received: May 25, 2020 Novel hybrid algorithm of the Whale Optimization Algorithm (WOA) pro- Accepted: June 27, 2020 posed with modified conjugate gradient Algorithm develops the elementary Published: June 30, 2020 society that randomly generated as the primary society for the Whales opti- Copyright © 2020 by author(s) and Open mization algorithm using the characteristics of the modified conjugate gra- Access Library Inc. dient algorithm. The efficiency of the hybrid algorithm measured by applying This work is licensed under the Creative it to (10) of the optimization functions of high measurement with different Commons Attribution International License (CC BY 4.0). dimensions and the results of the hybrid algorithm were very good in com- http://creativecommons.org/licenses/by/4.0/ parison with the original algorithm. Open Access Subject Areas Mathematical Logic, Foundation of Mathematics Keywords Conjugate Gradient Methods, Meta-Heuristic Algorithms, Whale Optimization Algorithm 1. Introduction Optimization can be defined as one of the branches of knowledge dealing with discovering or arriving at the optimal solutions to a specific issue within a set of alternatives. DOI: 10.4236/oalib.1106459 Jun. 30, 2020 1 Open Access Library Journal L. R. Khaleel, B. A. Mitras The methods of solving optimization problems divided into two types of algo- rithms: Deterministic Algorithms and Stochastic Algorithms. Most of classical algorithms are specific algorithms. For example, the Simplex method in linear programming is a specific algorithm, and some specific algo- rithms use tilt information (Gradient), which called slope-based algorithms. For example, Newton-Raphson algorithm) is an algorithm based on slope or deriva- tive [1]. As for random algorithms, they have two types of algorithms, although the difference between them is small: Heuristic Algorithms and Meta-Heuristic Al- gorithms [2]. The Whale Optimization Algorithm (WOA) is an algorithm inspired by the humpback whale search behavior for its food and hunting method and was first proposed by Lewis and Mirjalili (2016) [3]. In the same year, WOA improved by Trivedi and others by incorporating a new technology called WOA Adaptive Technology (WOA) [4]. In the same year, Touma studied the economic transmission problem on the IEEE Bus-30 system using a whale optimization algorithm, which gives good re- sults compared to other algorithms [5]. In 2017, Hu and others proposed an improved algorithm of whale optimiza- tion by adding its inertia weights called (WOAs). The new algorithm tested us- ing 27 functions and applied to predict the daily air quality index. The proposed algorithm showed efficiency compared to other algorithms [6]. This algorithm used in the same year by researchers Prakash and Lakshmina- rayana in determining the optimal location of the capacitors and determining their size in the radial distribution network, in order to reduce the losses of the distribution network line as the positioning of the capacitors in optimal loca- tions will improve system performance, stability and reliability [7]. In the same year, the researcher Desuky used a whale optimization algorithm to improve two levels of male fertility classification. Recently, diseases and health problems that were common among the elderly only became common among young people, and some of the causes of these medical problems are behavioral, environmental and lifestyle factors. The whale optimization algorithm then combined with the Pegasos algorithm to enhance the male fertility rating at both levels. This integration improved the results by 90% [8]. The algorithm of the whale optimization was also used in the same year by Reddy and others to optimize renewable resources to reduce losses in electricity distribution systems [9]. In the same year, Mafarja and Mirjalili crossed the whale optimization algo- rithm with Simulated Annealing Algorithm and used in the classification process. The results confirm the efficiency of the hybrid algorithm in improving classifi- cation accuracy [10]. The aim of the research is to propose a new hybrid algorithm consisting of a Whale optimization algorithm (WOA) with Modified traditional Conjugate DOI: 10.4236/oalib.1106459 2 Open Access Library Journal L. R. Khaleel, B. A. Mitras Gradient Directional Methods (WOA-MCG). Table 1 represents a definition of the variables used in this study. 2. Conjugate Gradient Method In unconstrained optimization, we minimize an objective function depends on real variables with no restrictions on the values of these variables. The uncon- strained optimization problem is: minfx( ) : x∈ Rn , (1) where fR: n → R is a continuously differentiable function, bounded from be- low. A nonlinear conjugate gradient method generates a sequence {xk } , k: in- teger number, k ≥ 0 . Starting from an initial point x0 , the value of xk calcu- late by the following equation: xxdk+1 = k + λ kk, (2) where the positive step size λk > 0 is obtained by a line search, and the direc- tions dk are generated as: dgk++11=−+ kβ kk d, (3) where dg00= − , the value of βk is determined according to the algorithm of Conjugate Gradient (CG), and its known as a conjugate gradient parameter, sxkk=+1 − x k and gkk=∇= fx( ) f′( x k) , consider . is the Euclidean norm and ygkk=+1 − g k. The termination conditions for the conjugate gradient line search are often based on some version of the Wolfe conditions. The standard Wolfe conditions: T fx( k+−λ kk d) fx( k) ≤ρλ kk gd k, (4) T T gx( k+≥λσ kk d) d k gd k k, (5) Table 1. Represents a definition of the variables used. Variables provide . The standard for any vector ε Small positive value x Local minimum point of function fx( ) λk Positive parameter to minimize the function fx( ) ∇ Directional derivative G Gradient vector of pattern n×1 Gk Hessian matrix nn× dk Vector search pattern n×1 f Target function yk Vector is the difference between two consecutive gradients of the pattern n×1 DOI: 10.4236/oalib.1106459 3 Open Access Library Journal L. R. Khaleel, B. A. Mitras where dk is a descent search direction and 01<≤<ρσ , where βk is de- fined by one of the following formulas: ygTT g g yg T ββ(HS ) = kk+1 (FR) = k++11 k β(PRP) = kk+1 kkTTT; k (6) ydkk ggkk ggkk ggT yg TT gg β(CD) =−kk++11 ββ(LS ) =−=kk+1 (DY ) kk++11 kT;; kk TT (7) gdkk gdkk yskk Al-Bayati and Al-Assady (1986) proposed three forms for the scalar β k de- fined by: 2 22 y yy ββAB12==kAB −= kk βAB 3 kk2T;; k T (8) [11] gk dgkk dykk 3. Proposed a New Conjugacy Coefficient We have the quasi-Newton condition yk= Gs kk (9) We multiply both sides of Equation (9) by sk and we get TT [ yk= G kk s]* s k ⇒= y kk s Gs kk s ysT =kk ⋅ GI2 nn× (10) sk N −1 Let dk++11= −λ Gg kk (11) ysT N = −λ kk dgkk++112 (12) sk Multiply both sides of Equation (12) by yk and we get T TTN yskk ydkk++11= −λ ygkk (13) 2 sk TTCG T ⇒ydk k++11 =−+ yg k kβ kk d y k (14) From (13) and (14) we have T TTyskk T −+ygkk++11βλ kkk dy =−ygkk (15) 2 sk T (DY ) gg We assume that ββ= = kk++11 kk ydT kk Then we have T TTDY yskk T −+ygkk++11βλ k dy kk =−ygkk (16) 2 sk 2 T TTgk +1 yskk T −+ygkk++11 dykk =−λ ygkk (17) dyT2 kk sk DOI: 10.4236/oalib.1106459 4 Open Access Library Journal L. R. Khaleel, B. A. Mitras From Equation (17) we get: T TTyskk −ygkk++11 +=−βτ kk λygkk (18) 2 sk Then, we have 2 T sk yskk TT −+ygkk++11 yg kk 2 f−+ f gsT 2 k k++11 kk sk βk = (19) τ k T yskk TT −+yg++ yg −+T kk11 kk 2( fk f k++11 gs kk) βk = (20) τ k T yskk T 1− yg+ −+T kk1 2( fk f k++11 gs kk) βk = (21) τ k 2 Since τ k +1 > 0 then we suppose: τ kk= g then: ysT 1− kk ygT T kk+1 2( fk−+ f k++11 gs kk) β = k 2 . (22) gk 3.1. Outlines of the Proposed Algorithm n Step (1): The initial step: We select the starting point xR0 ∈ , and we select the accuracy solution ε > 0 is a small positive real number and we find dgkk= − , λ00= Minary( g ) , and we set k = 0 . Step (2): The convergence test: If gk ≤ ε then stop and set the optimal so- lution is xk . Else, go to step (3). Step (3): The line search: We compute the value of λk by Cubic method and that satisfy the Wolfe conditions in Equations (4), (5) and go to step (4). Step (4): Update the variables: xxdk+1 = k + λ kk and compute fx( kk++11), g and sxkk=+1 − x k, ygkk=+1 − g k.
Details
-
File Typepdf
-
Upload Time-
-
Content LanguagesEnglish
-
Upload UserAnonymous/Not logged-in
-
File Pages18 Page
-
File Size-