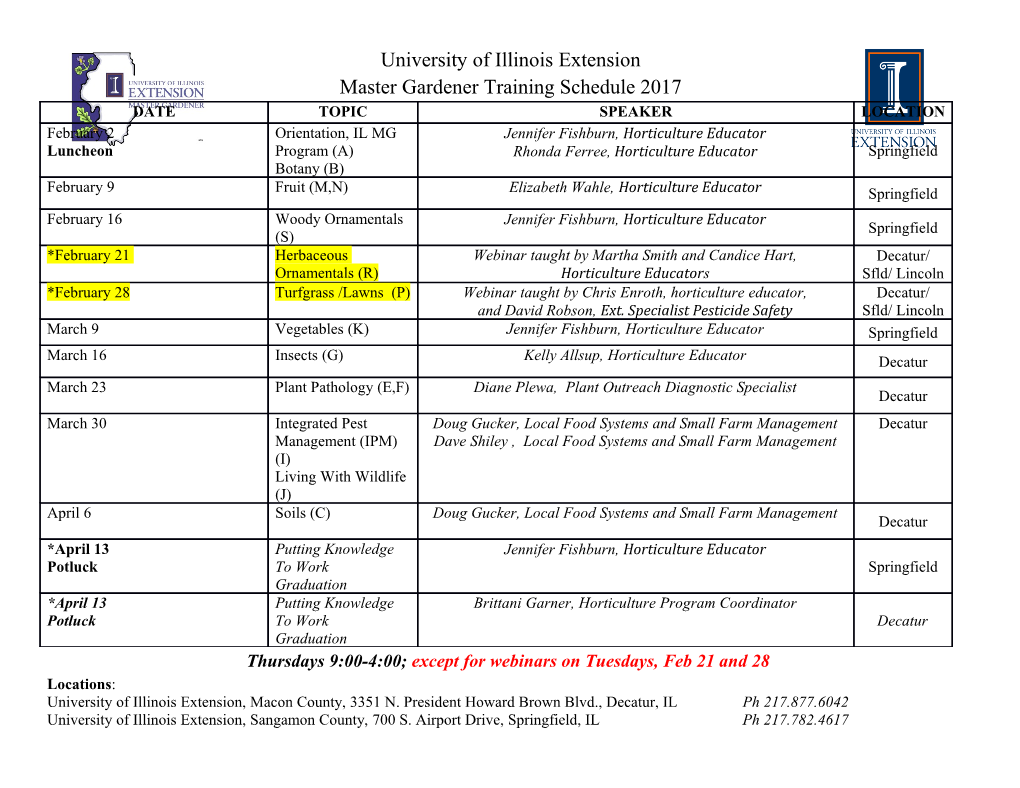
Audio Information Extraction Dan Ellis <[email protected]> Laboratory for Recognition and Organization of Speech and Audio (LabROSA) Electrical Engineering, Columbia University http://labrosa.ee.columbia.edu/ Outline 1 Audio information extraction 2 Speech, music, and other 3 General sound organization 4 Future work & summary AIE @ MIT - Dan Ellis 2002-04-23 - 1 Lab ROSA 1 Audio Information Extraction 4000 frq/Hz 3000 0 2000 -20 1000 -40 0 -60 0 2 4 6 8 10 12 time/s level / dB Analysis Voice (evil) Voice (pleasant) Stab Choir Rumble Strings • Analyzing and describing complex sounds: - continuous sound mixture → distinct objects & events • Human listeners as the prototype - strong subjective impression when listening - ..but hard to ‘see’ in signal AIE @ MIT - Dan Ellis 2002-04-23 - 2 Lab ROSA Bregman’s lake “Imagine two narrow channels dug up from the edge of a lake, with handkerchiefs stretched across each one. Looking only at the motion of the handkerchiefs, you are to answer questions such as: How many boats are there on the lake and where are they?” (after Bregman’90) • Received waveform is a mixture - two sensors, N signals ... • Disentangling mixtures as primary goal - perfect solution is not possible - need knowledge-based constraints AIE @ MIT - Dan Ellis 2002-04-23 - 3 Lab ROSA The information in sound Steps 1 Steps 2 4000 3000 freq / Hz 2000 1000 0 0 1 2 3 4 0 1 2 3 4 time / s • Hearing confers evolutionary advantage - optimized to get ‘useful’ information from sound • Auditory perception is ecologically grounded - scene analysis is preconscious (→ illusions) - special-purpose processing reflects ‘natural scene’ properties - subjective not canonical (ambiguity) AIE @ MIT - Dan Ellis 2002-04-23 - 4 Lab ROSA Positioning AIE AutomaticSpeech Recognition Music ASR Information Retrieval Music IR Text Audio Information Spoken Fingerprinting Retrieval Document IR Retrieval SDR Audio Audio Text Information Content-based Information Extraction Retrieval Multimedia Extraction AIE Audio CBR Information IE Retrieval MMIR Independent Component Unsupervised Blind Source Computational Clustering Analysis Auditory Separation ICA BSS Scene Analysis CASA • Domain - text ... speech ... music ... general audio • Operation - recognize ... index/retrieve ... organize AIE @ MIT - Dan Ellis 2002-04-23 - 5 Lab ROSA AIE Applications • Multimedia access - sound as complementary dimension - need all modalities for complete information • Personal audio - continuous sound capture quite practical - different kind of indexing problem • Machine perception - intelligence requires awareness - necessary for communication • Music retrieval - area of hot activity - specific economic factors AIE @ MIT - Dan Ellis 2002-04-23 - 6 Lab ROSA Outline 1 Audio information extraction 2 Speech, music, and other - Speech recognition: Tandem modeling - Multi-speaker processing: Meeting recorder - Music classification - Other sounds 3 General sound organization 4 Future work & summary AIE @ MIT - Dan Ellis 2002-04-23 - 7 Lab ROSA Automatic Speech Recognition (ASR) • Standard speech recognition structure: sound Feature calculation feature vectors Acoustic model parameters Acoustic classifier Word models phone probabilities s ah t HMM D A T A D A Language model decoder p("sat"|"the","cat") phone / word p("saw"|"the","cat") sequence Understanding/ application... •‘State of the art’ word-error rates (WERs): - 2% (dictation) - 30% (telephone conversations) • Can use multiple streams... AIE @ MIT - Dan Ellis 2002-04-23 - 8 Lab ROSA Tandem speech recognition (with Hermansky, Sharma & Sivadas/OGI, Singh/CMU, ICSI) • Neural net estimates phone posteriors; but Gaussian mixtures model finer detail • Combine them! Hybrid Connectionist-HMM ASR Conventional ASR (HTK) Feature Neural net Noway Feature Gauss mix HTK calculation classifier decoder calculation models decoder h# C0 pcl bcl C tcl 1 dcl C2 Ck tn s ah t s ah t tn+w Input Speech Phone Words Input Speech Subword Words sound features probabilities sound features likelihoods Tandem modeling Feature Neural net Gauss mix HTK calculation classifier models decoder h# C0 pcl bcl C tcl 1 dcl C2 Ck tn s ah t tn+w Input Speech Phone Subword Words sound features probabilities likelihoods • Train net, then train GMM on net output - GMM is ignorant of net output ‘meaning’ AIE @ MIT - Dan Ellis 2002-04-23 - 9 Lab ROSA Tandem system results • It works very well (‘Aurora’ noisy digits): WER as a function of SNR for various Aurora99 systems 100 50 Average WER ratio to baseline: HTK GMM: 100% Hybrid: 84.6% 20 Tandem: 64.5% Tandem + PC: 47.2% 10 5 WER / % (log scale) HTK GMM baseline 2 Hybrid connectionist Tandem Tandem + PC 1 -5 0 5 10 15 20 clean SNR / dB (averaged over 4 noises) System-features Avg. WER 20-0 dB Baseline WER ratio HTK-mfcc 13.7% 100% Neural net-mfcc 9.3% 84.5% Tandem-mfcc 7.4% 64.5% Tandem-msg+plp 6.4% 47.2% AIE @ MIT - Dan Ellis 2002-04-23 - 10 Lab ROSA Relative contributions • Approx relative impact on baseline WER ratio for different components: Combo over msg: +20% plp Neural net classifier h# C0 pcl bcl C tcl 1 dcl C2 Ck tn tn+w PCA Gauss mix HTK orthog'n models decoder Pre-nonlinearity over + Input posteriors: +12% sound s ah t msg Neural net Words classifier PCA over Combo-into-HTK over h# C0 pcl bcl Combo-into-noway: tcl direct: C 1 dcl C2 +8% +15% Ck tn Combo over plp: tn+w +20% Combo over mfcc: NN over HTK: Tandem over HTK: Tandem over hybrid: +25% +15% +35% +25% Tandem combo over HTK mfcc baseline: +53% AIE @ MIT - Dan Ellis 2002-04-23 - 11 Lab ROSA Inside Tandem systems: What’s going on? • Visualizations of the net outputs “one eight three” Clean 5dB SNR to ‘Hall’ noise (MFP_183A) 4 4 10 0 3 3 -10 Spectrogram 2 2 -20 freq / kHz 1 1 -30 0 0 -40 dB 7 10 10 6 Cepstral-smoothed 5 mel spectrum 5 5 4 freq / mel chan 0 0 3 q q h# h# 1 ax ax uw uw ow ow ao ao ah ah ay ay ey ey eh eh Phone posterior ih ih iy iy w w r r 0.5 estimates n n phone v v th th f f z z s s kcl kcl tcl tcl k k t t 0 q q h# h# 20 ax ax uw uw ow ow ao ao ah ah ay ay 10 ey ey eh eh ih ih Hidden layer iy iy w w 0 r r n n phone v v linear outputs th th f f z z -10 s s kcl kcl tcl tcl k k t t -20 0 0.2 0.4 0.6 0.8 1 0 0.2 0.4 0.6 0.8 1 time / s time / s • Neural net normalizes away noise AIE @ MIT - Dan Ellis 2002-04-23 - 12 Lab ROSA Tandem for large vocabulary recognition • CI Tandem front end + CD LVCSR back end PLP feature Neural net Tandem SPHINX calculation classifier 1 feature recognizer h# C0 pcl calculation bcl C tcl 1 dcl C2 Ck tn tn+w PCA GMM HMM decorrelation classifier decoder Pre-nonlinearity + Input outputs sound Subword Words likelihoods s ah t MSG feature Neural net calculation classifier 2 MLLR h# C0 pcl bcl tcl adaptation C 1 dcl C2 Ck tn tn+w • Tandem benefits reduced: 75 mfc plp 70 tandem1 tandem2 65 60 55 WER / % 50 45 40 35 30 Context Context Context Dep. Independent Dependent +MLLR AIE @ MIT - Dan Ellis 2002-04-23 - 13 Lab ROSA ‘Tandem-domain’ processing (with Manuel Reyes) • Can we improve the ‘tandem’ features with conventional processing (deltas, normalization)? Neural net PCA GMM h# C0 pcl bcl Norm / decorrelation Rank Norm / classifier C tcl 1 dcl ... C2 deltas reduce deltas ... Ck t ? ? ? n tn+w • Somewhat.. Processing Avg. WER 20-0 dB Baseline WER ratio Tandem PLP mis- 11.1% 70.3% match baseline (24 els) Rank reduce @ 18 els 11.8% 77.1% Delta → PCA 9.7% 60.8% PCA → Norm 9.0% 58.8% Delta → PCA → Norm 8.3% 53.6% AIE @ MIT - Dan Ellis 2002-04-23 - 14 Lab ROSA Tandem vs. other approaches Aurora 2 Eurospeech 2001 Evaluation 60 Columbia Philips UPC Barcelona 50 Bell Labs IBM 40 Motorola 1 Motorola 2 30 Nijmegen ICSI/OGI/Qualcomm 20 ATR/Griffith AT&T Alcatel 10 Siemens UCLA 0 Microsoft Rel improvement % - Multicondition Avg. rel. improvement Slovenia -10 Granada • 50% of word errors corrected over baseline • Beat ‘bells and whistles’ system using large-vocabulary techniques AIE @ MIT - Dan Ellis 2002-04-23 - 15 Lab ROSA Missing data recognition (Cooke, Green, Barker @ Sheffield) • Energy overlaps in time-freq. hide features - some observations are effectively missing • Use missing feature theory... - integrate over missing data xm under model M () (), () pxM = ∫ pxp xm M pxm M dxm • Effective in speech recognition - trick is finding good/bad data mask "1754" + noise 90 AURORA 2000 - Test Set A 80 70 MD Discrete SNR 60 MD Soft SNR Missing-data HTK clean training Recognition 50 HTK multi-condition WER 40 "1754" 30 Mask based on 20 stationary noise estimate 10 -50 0 5 10 15 20 Clean AIE @ MIT - Dan Ellis 2002-04-23 - 16 Lab ROSA Maximum-likelihood data mask (Jon Barker @ Sheffield) • Search of sound-fragment interpretations "1754" + noise Mask based on stationary noise estimate Mask split into subbands `Grouping' applied within bands: Spectro-Temporal Proximity Common Onset/Offset Multisource "1754" Decoder - also search over data mask K: pMKx(), ∝ pxKM(), pKM()pM() • Modeling mask likelihoods p(K) ... AIE @ MIT - Dan Ellis 2002-04-23 - 17 Lab ROSA Multi-source decoding • Search for more than one source q2(t) O(t) K2(t) K1(t) q1(t) • Mutually-dependent data masks • Use CASA processing to propose masks - locally coherent regions - p(K|q) • Theoretical vs.
Details
-
File Typepdf
-
Upload Time-
-
Content LanguagesEnglish
-
Upload UserAnonymous/Not logged-in
-
File Pages42 Page
-
File Size-