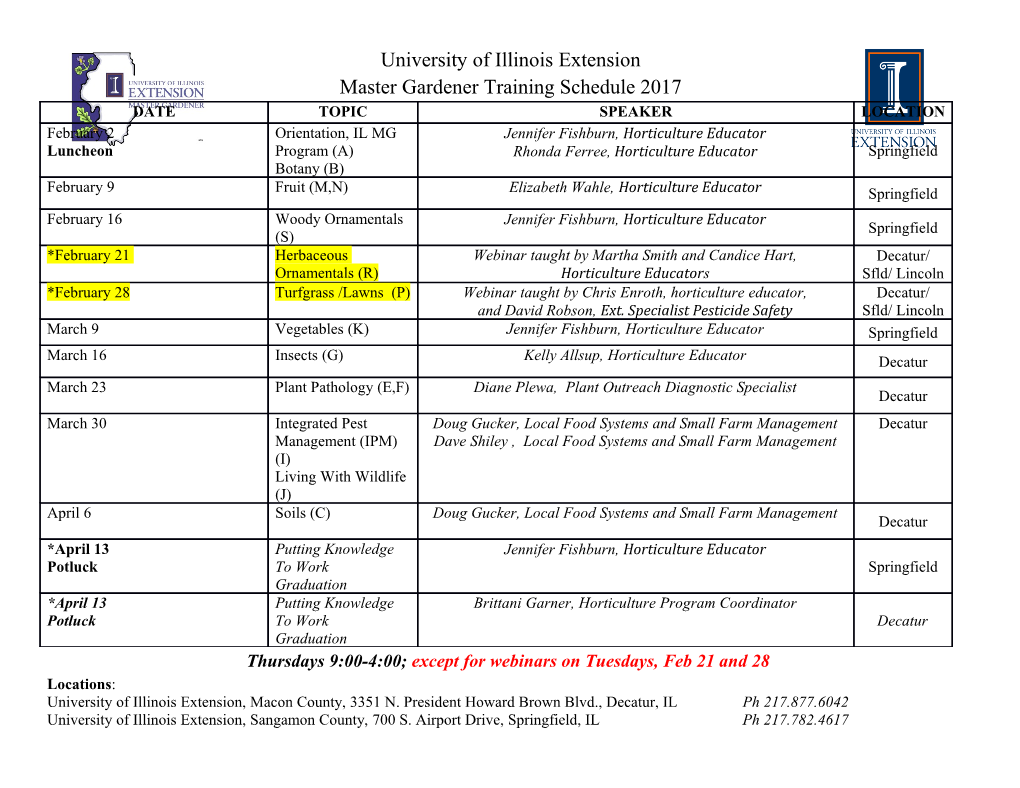
university of copenhagen department of biostatistics university of copenhagen department of biostatistics Faculty of Health Sciences Contents ◮ Planning statistical analyses with missing data. ◮ Missing data Missing data types. ◮ Bias due to missing data or improper handling of these. Analysis of repeated measurements 2017 ◮ Analyzing data with missing values using multiple imputations, likelihood inference, or inverse probability weighting. Julie Lyng Forman & Lene Theil Skovgaard ◮ Analysis of longitudinal studies with death or other Department of Biostatistics, University of Copenhagen intercurrent events. Suggested reading FLW (2011) chapters 18 +19, lecture notes. 2 / 60 university of copenhagen department of biostatistics university of copenhagen department of biostatistics Outline What is missing data? What to worry about when you have missing data Most investigations are planned to be balanced but almost inevitably turn out to have intermittent missing values , or Missing data types patients who drop-out for some reason . Simple methods for handling missing data ◮ Just by coincidence (sample lost or ruined). Advanced methods for handling missing data ◮ The patient moved away (may be worrysome). ◮ The patient has recovered (worrying, i.e. carrying Missing data in population average models (binary data) information). Death and other intercurrent events in longitudinal studies ◮ The patient is too ill to show up (very serious, i.e. carrying unretrievable information). Evaluation 3 / 60 4 / 60 university of copenhagen department of biostatistics university of copenhagen department of biostatistics Missing data is trouble Planning statistical analyses with missing data Missing data should be addressed already in the planning stage. ◮ It complicates statistical analysis. 1. What are the outcomes and explanatory variables? ◮ It may bias statistical results beyond repair. 2. What are the parameters of interest (the study objective)? ◮ It compromises the causal interpretation of treatment effect in randomized trials. 3. Which variables may have missing values? ◮ It reduces statistical power since information is lost. 4. What are the likely reasons they are missing? 5. What other factors (auxiliary variables) could be associated The best way to handle missing data would be to prevent it, with missingness? Are they also associated with the outcome? but this is often not possible . This helps us decide: Missing data should always be recognized as a limitation. 6. What statistical methods should be used for analyses? 5 / 60 6 / 60 university of copenhagen department of biostatistics university of copenhagen department of biostatistics The missing data mechanism Example: CKD study from lecture 1 It is important to understand WHY data is missing. Investigate: ◮ If possible, ask the patients or investigators! ◮ Make separate spaghettiplots for completers and drop-outs. ◮ Make a table comparing the distribution of covariates and other characteristics between the drop-outs and the completers. Speculate: ◮ Think about what differences there might be e.g. between completers and drop-outs in terms of unmeasured outcomes and confounders. ◮ How could these affect the results of your analysis . More drop outs due to adverse events in Eplerenone group! 7 / 60 8 / 60 university of copenhagen department of biostatistics university of copenhagen department of biostatistics Study objectives FDA recommendations for clinical trials What parameter is the target of the study or trial? In your study protocol please include a section describing how you plan to address missing data. Mean of actual data had they all been collected, e.g. We recommend missing data be avoided by continuing to collect ◮ Change in mean over time of the entire study population. (efficacy and safety) data even from subjects who prematurely ◮ Difference in means between two populations at a given time. discontinue study drug. ◮ Difference in means between the initially randomized Our preference is that the primary analysis 1) include all data, not treatment groups regardless of what treatment subjects just data while adhering to study drug, and 2) for the limited actually received ( intention to treat principle ). missing data that do occur, it be represented by what their response likely would have been had it been measured. Mean of counterfactual data had they all been collected, e.g. Because missing data tend to be associated with treatment ◮ Difference in means that would have been found if all subjects adherence, it would not be appropriate to have an analysis that had completed their assigned treatment . uses information from those with data who adhered to treatment ◮ Difference in populations means if all had survived until end of to describe what happened to those without data who did not follow-up . adhere to treatment. 9 / 60 10 / 60 university of copenhagen department of biostatistics university of copenhagen department of biostatistics Your own data Outline Think about the data from your own research project. What to worry about when you have missing data Missing data types ◮ Are any data missing? ◮ How many? Simple methods for handling missing data ◮ Do you know WHY? Advanced methods for handling missing data ◮ Are the observed outcomes representative of the population Missing data in population average models (binary data) you wanted to study, or different somehow? ◮ What exactly is your study objective, considering the missing Death and other intercurrent events in longitudinal studies data? Evaluation 11 / 60 12 / 60 university of copenhagen department of biostatistics university of copenhagen department of biostatistics Types and patterns of missingness MCAR: Missing completely at random Missing data taxonomy MCAR Missing completely at random. Examples: Unrelated to the outcome and the covariates. ◮ Sample lost in the mail. Note: Special case of MAR. ◮ Some data too expensive/inconvenient to collect from the MAR Missing at random. whole sample, hence only collected for a random subsample. Missingness is conditionally independent of the missing values given the observed data. Statistical consequences ◮ NMAR Not missing at random. Reduced power due to reduced sample size . Missingness is not conditionally independent of the ◮ Data may end up being unbalanced (software problem - ?) missing values given the observed data. ◮ Otherwise benign . Missing data patterns in longitudinal studies If missing data is MCAR, then the complete cases form a random Monotone Drops out and stays out. representative subsample from the original study population . Intermittent (aka non-monotone) Comes back later. 13 / 60 14 / 60 university of copenhagen department of biostatistics university of copenhagen department of biostatistics Average curves Example of a missing mechanism MAR Low values are good (e.g. blood pressure): The average curve is representative of the whole population when ◮ When the patient learns he is doing well, he might decide he data is complete or missing data is MCAR. no longer needs to attend visits and staying away does not ◮ When missing data is MAR or NMAR it is likely biased . affect his outcome. Spaghettiplots are always ok. Sample averages are biased. Mean estimates from LMM are ok . 15 / 60 16 / 60 university of copenhagen department of biostatistics university of copenhagen department of biostatistics Example of a missing data mechanism NMAR MAR: Missing at random Low values are bad (e.g. lung function): R1 denotes the response indicator (1=observed, 0=missing). / ◮ When the person gets sufficiently ill, he drops out of the X Y0 labour market ( healthy worker effect ). Simplified DAG ' /' potential drop out Y1 R1 only after baseline. ~ Y2 ◮ Missingness may depend on past observed outcomes and covariates included in the model, e.g. treatment . ◮ Missingness may not depend on current outcome neither directly nor by means of unmeasured confounders . ◮ Future outcome of interest (de facto or counterfactual) after drop out may not depend on missingness. Sample averages are biased . Mean estimates from LMM too. 17 / 60 18 / 60 university of copenhagen department of biostatistics university of copenhagen department of biostatistics MAR depends on the covariates Case: Missing data in calcium study Assume a treatment-gender interaction (or -gene, or . ): ◮ Positive effect in women. Negative effect in men. ◮ Men are overall more likely to drop out. An average positive change is found in the population if gender is not included in the model and the interaction is not recognized! Drop-outs tend to have lower BMD initially. 19 / 60 20 / 60 university of copenhagen department of biostatistics university of copenhagen department of biostatistics Objectives of calcium study Case: Missing data in calcium study Likely causes and effects of drop-out: Two possibilities for defining the target treatment effect: ◮ We expect that the positive effect of calcium ceases when the girl drops out of the trial (NMAR for target 1). 1. Difference in de facto mean BMD at end of study for everyone. ◮ Family moves away or too busy to participate (MCAR for 2. Difference in mean BMD which would have been found had target 2 unless related to unmeasured confounders). everyone completed their assigned treatment. ◮ Parents learn at the visit that BMD is low and decides to Which is closest to the effect of an intervention in the population withdraw because they think the girl is on placebo but needs is not that obvious, since reasons for discontinuing treatment in treatment (MAR for target 2). real life may be
Details
-
File Typepdf
-
Upload Time-
-
Content LanguagesEnglish
-
Upload UserAnonymous/Not logged-in
-
File Pages15 Page
-
File Size-