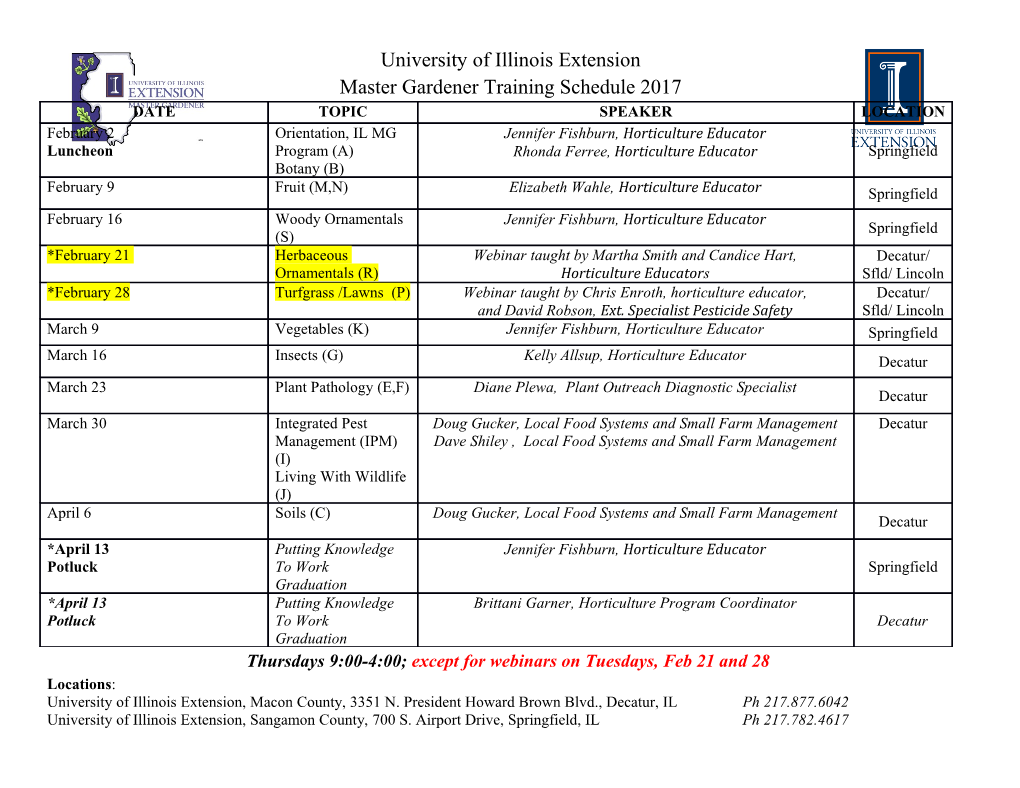
HETEROGENEOUS CPU+GPU COMPUTING Ana Lucia Varbanescu – University of Amsterdam [email protected] Significant contributions by: Stijn Heldens (U Twente), Jie Shen (NUDT, China), Heterogeneous platforms • Systems combining main processors and accelerators • e.g., CPU + GPU, CPU + Intel MIC, AMD APU, ARM SoC • Everywhere from supercomputers to mobile devices Heterogeneous platforms • Host-accelerator hardware model Accelerator FPGAs Accelerator PCIe / Shared memory ... Host MICs Accelerator GPUs Accelerator CPUs Our focus today … • A heterogeneous platform = CPU + GPU • Most solutions work for other/multiple accelerators • An application workload = an application + its input dataset • Workload partitioning = workload distribution among the processing units of a heterogeneous system Few cores Thousands of Cores 5 Generic multi-core CPU 6 Programming models • Pthreads + intrinsics • TBB – Thread building blocks • Threading library • OpenCL • To be discussed … • OpenMP • Traditional parallel library • High-level, pragma-based • Cilk • Simple divide-and-conquer model abstractionincreasesLevel of 7 A GPU Architecture Offloading model Kernel Host code 9 Programming models • CUDA • NVIDIA proprietary • OpenCL • Open standard, functionally portable across multi-cores • OpenACC • High-level, pragma-based • Different libraries, programming models, and DSLs for different domains Level of abstractionincreasesLevel of CPU vs. GPU 10 ALU ALU CPU Control Low latency, high Throughput: ~ALU 500 GFLOPsALU flexibility. Bandwidth: ~ 60 GB/s Excellent for irregular codes with Cache limited parallelism. Heterogeneous computingDo we gain= CPU anything? + GPU work together … GPU High throughput. Throughput: ~ 5.500 GFLOPs Excellent for Bandwidth: ~ 350 GB/s massively parallel workloads. Case studies 450 TG TD TC TMax 400 350 (ms) 300 250 Matrix Multiplication (SGEMM) time Best = (80,20) 200 n = 2048 x 2048 (48MB) 150 Execution 100 50 0 Only-GPU Only-CPU granularity = 10% TG = GPU kernel execution time TG TD TD = Data transfer time TC TC = CPU kernel execution time TMax= max(TG+TD, TC) TMax Case studies 450 TG TD TC TMax 400 TG = GPU kernel execution time 350 TD = Data transfer time (ms) 300 Best = (80,20) T = CPU kernel execution time 250 C time 200 TMax= max(TG+TD, TC) 150 Execution 100 50 Matrix Multiplication (SGEMM) 0 n = 2048 x 2048 (48MB) Only-GPU Only-CPU granularity = 10% 250 TG TD TC TMax 180 TG TD TC TMax 160 200 140 (ms) (ms) 120 Best = (30,70) 150 Best =Only-CPU time 100 time 80 100 60 Execution Execution 40 50 20 0 0 Very few applications are GPU-only. Separable Convolution (SConv) Dot Product (SDOT) n = 6144x6144 (432MB) n = 14913072 (512MB) Possible advantages of heterogeneity • Increase performance • Both devices work in parallel • Decrease data communication • Which is often the bottleneck of the system • Different devices for different roles • Increase flexibility and reliability • Choose one/all *PUs for execution • Fall-back solution when one *PU fails • Increase energy efficiency • Cheaper per flop Main challenges: programming and workload partitioning! Challenge 1: Programming models 15 Programming models (PMs) • Heterogeneous computing = a mix of different processors on the same platform. • Programming • Mix of programming models • One(/several?) for CPUs – OpenMP • One(/several?) for GPUs – CUDA • Single programming model (unified) • OpenCL is a popular choice Low level High level 4.0 OmpSs 16 Heterogeneous Computing PMs High productivity; not Domain and/or all applications are High application specific. easy to implement. level Focus on: productivity and performance OpenACC, OpenMP 4.0 HyGraph, OmpSS, StarPU, … Cashmere, HPL Generic GlassWing Specific TOTEM OpenCL OpenMP+CUDA Domain specific, focus The most common atm. on performance. Useful for performance, Low More difficult to use. more difficult to use in level Quite rare. practice Heterogeneous computing PMs • CUDA + OpenMP/TBB • Typical combination for NVIDIA GPUs • Individual development per *PU • Glue code can be challenging • OpenCL (KHRONOS group) • Functional portability => can be used as a unified model • Performance portability via code specialization • HPL (University of A Coruna, Spain) • Library on top of OpenCL, to automate code specialization Heterogeneous computing PMs • StarPU (INRIA, France) • Special API for coding • Runtime system for scheduling • OmpSS (UPC + BSC, Spain) • C + OpenCL/CUDA kernels • Runtime system for scheduling and communication optimization Heterogeneous computing PMs • Cashmere (VU Amsterdam + NLeSC) • Dedicated to Divide-and-conquer solutions • OpenCL backend. • GlassWing (VU Amsterdam) • Dedicated to MapReduce applications • TOTEM (U. of British Columbia, Canada) • Graph processing • CUDA+Multi-threading • HyGraph (TUDelft, UTwente, UvA, NL) • Graph processing • Based on CUDA+OpenMP Challenge 2: Workload partitioning Workload • DAG (directed acyclic graph) of “kernels” k0 k0 k0 k2 k0 k0 k1 k1 k1 ... ... k3 k4 kn kn k5 I II III IV V SK-One SK-Loop MK-Seq MK-Loop MK-DAG 22 Determining the partition • Static partitioning (SP) vs. Dynamic partitioning (DP) Mul3ple Cores Thousands of Cores Mul3ple Cores Thousands of Cores 23 Static vs. dynamic • Static partitioning • + can be computed before runtime => no overhead • + can detect GPU-only/CPU-only cases • + no unnecessary CPU-GPU data transfers • -- does not work for all applications • Dynamic partitioning • + responds to runtime performance variability • + works for all applications • -- incurs (high) runtime scheduling overhead • -- might introduce (high) CPU-GPU data-transfer overhead • -- might not work for CPU-only/GPU-only cases 24 Determining the partition • Static partitioning (SP) vs. Dynamic partitioning (DP) Mul3ple Cores (near-) Optimal Often sub-ptimal High applicability Low applicabilityThousands of Cores Mul3ple Cores Thousands of Cores 25 Heterogeneous Computing today Limited applicability. Single Not interesting, Low overhead => high kernel given that static & performance run-time based Systems/frameworks: systems exist. Qilin, Insieme, SKMD, Glinda, ... Sporradic attempts Libraries: HPL, … and light runtime Static systems Dynamic Run-time based systems: Glinda 2.0 StarPU OmpSS HyGraph … Low overhead => high High Applicability, Multi-kernel performance high overhead Still limited in applicability. (complex) DAG Mul3ple Cores Thousands of Cores Static partitioning: Glinda Glinda* • Modeling the partitioning • The application workload • The hardware capabilities • The GPU-CPU data transfer • Predict the optimal partitioning • Making the decision in practice • Only-GPU • Only-CPU • CPU+GPU with the optimal partitioning *Jie Shen et al., HPCC’14. “Look before you Leap: Using the Right Hardware. Resources to Accelerate Applications 28 Modeling the partitioning • Define the optimal (static) partitioning • β= the fraction of data points assigned to the GPU (+TD) TG +TD = TC 29 Modeling the workload n−1 the area of the rectangle W = ∑wi ≈ i=0 n: the total problem size GPU par33on CPU par33on w: workload per work-item W =w x n x β W =w x n x (1-β) w G C n 0 1 β 1-β W (total workload size) quan3fies how much work has to be done Modeling the partitioning W: total workload size W W O W = (1-β) * W and W = β * W T = G T = C T = C G G P C P D Q P: processing throughput (W/second) G C O: CPU-GPU data-transfer size Q: CPU-GPU bandwidth (B/second) RGC CPU kernel time vs. WG PG 1 GPU kernel time T +T = T = × G D C W P PG O C C 1+ × Q WG RGD GPU data-transfer time vs. GPU kernel execution time Predicting the optimal partitioning • Solving β from the equation Total workload size W P 1 G = G × HW capability ratios W P PG O β predictor C C 1+ × Data transfer size Q WG • There are three β predictors (by data transfer type) v R R R R GC − × GD β = GC β = GC β = w 1 R v + GC 1+ × R + R 1+ RGC w GD GC No data transfer Partial data transfer Full data transfer 32 Glinda outcome • (Any?) data-parallel application can be transformed to support heterogeneous computing • A decision on the execution of the application • only on the CPU • only on the GPU • CPU+GPU • And the partitioning point Results [1] • Quality (compared to an oracle) • The right HW configuration in 62 out of 72 test cases • Optimal partitioning when CPU+GPU is selected Predicted partitioning ) ms Optimal partitioning (auto-tuning) Execution time( Results [2] • Effectiveness (compared to Only-CPU/Only-GPU) • 1.2x-14.6x speedup • If taking GPU for granted, up to 96% performance will be lost Only- Only- Our config GPU CPU Execution time(ms) Related work Glinda achieves similar or better performance than the other partitioning approaches with less cost More advantages … • Support different types of heterogeneous platforms • Multi-GPUs, identical or non-identical • Support different profiling options suitable for different execution scenarios • Online or offline • Partial of full • Determine not only the optimal partitioning but also the best hardware configuration • Only-GPU / Only-CPU / CPU+GPU with the optimal partitioning • Support both balanced and imbalanced applications Glinda: limitations? • Regular applications only >> NO, we support imbalanced applications, too. • Single kernel only? >> NO, we support two types of multi- kernel applications, too. • Single GPU only? >> NO, we support multiple GPUs/ accelerators on the same node • Single node only? >> YES – for now . What about energy? • The Glinda model can be adapted to optimize for energy • Ongoing work at UvA • Not yet clear whether “optimality” can be so easily
Details
-
File Typepdf
-
Upload Time-
-
Content LanguagesEnglish
-
Upload UserAnonymous/Not logged-in
-
File Pages83 Page
-
File Size-