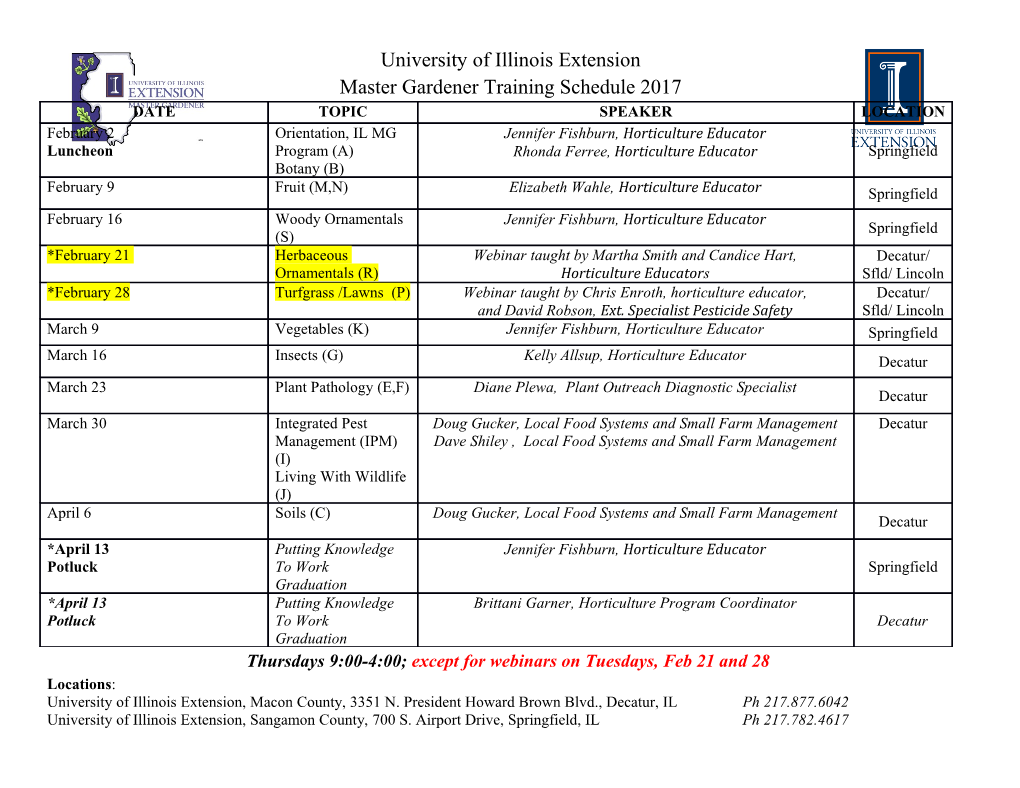
Journal of Nanotoxicology and Nanomedicine Volume 2 • Issue 1 • January-June 2017 exploring Simple, Interpretable, and Predictive QSPR Model of Fullerene C60 Solubility in organic Solvents Lyudvig S. Petrosyan, Department of Physics, Jackson State University, Jackson, MS, USA Supratik Kar, Interdisciplinary Center for Nanotoxicity, Department of Chemistry and Biochemistry, Jackson State University, Jackson, MS, USA Jerzy Leszczynski, Interdisciplinary Center for Nanotoxicity, Department of Chemistry and Biochemistry, Jackson State University, Jackson, MS, USA Bakhtiyor Rasulev, Department of Coatings and Polymeric Materials, North Dakota State University, Fargo, ND, USA ABSTRACT Buckminsterfullerene(C60)anditsderivativeshavecurrentlybeenusedaspromisingnanomaterial fordiagnosticandtherapeuticagents.Theyareappliedinpharmaceuticalindustryduetotheir ,nanostructurecharacteristics,stabilityandhydrophobiccharacter.Duetoitssparinglysolublenature thesolubilityofC60hasbeenofenormousattentionamongcarbonnanostructureinvestigatorsowing toitsfundamentalimportanceandpracticalinterestinnanotechnologyandmedicalindustry.In ordertostudythediverseroleofC60anditsderivativesthedependenceoffullerene’ssolubilityon molecularstructureofthesolventmustbeunderstood.Currentstudywasdedicatedtotheexploration ofthesolubilityoffullereneC60in156organicsolventsusingsimple,interpretableandpredictive .1Dand2Ddescriptorsemployingquantitativestructure-propertyrelationship(QSPR)technique (Theauthorsemployedgeneticalgorithmfollowedbymultiplelinearregressionanalysis(GA-MLR togeneratethecorrelationmodels.Thebestperformanceisaccomplishedbythefour-variableMLR 2 2 modelwithinternalandexternalpredictioncoefficientofQ =0.86andR pred=0.89.Thestudy identifiedvitalpropertiesandstructuralfragments,particularlyvaluableforguidingfuturesynthetic aswellaspredictionefforts.Themodelgeneratedwiththehighestnumberoforganicsolventstodate withsimpledescriptorscanbereproducedinnotimetopredictthesolubilityofC60inanynewor existingorganicsolvents.Thisapproachcanbeusedasanefficientpredictorforfullerenes’solubility .invariousorganicsolvents KeywoRdS C60, Chemometrics, Fullerene, GA, MLR, QSPR, Solubility 1. INTRODUCTIoN Fullerene,ahighlysymmetricalcage-likemoleculehasspecificinteractionwithorganicsolventsand .itsknowledgecanprovidesignificantinformationonthemechanismsofsolute-solventinteractions Thefullereneshavedefinedrigidgeometriesindistinctiontoothersoluteswhoseshapesundergo conformationalchanges.Notonlythatintramolecularvibrationalpartitionfunctionsmayundergo solvent-dependent changes (Prylutskyy et al., 2003). Due to sparingly soluble nature of C60 in .(majororganicsolvents,theproductioncostisstillhighforthisnanomaterial(Shunaevetal.,2015 ,Therefore,understandingoffullerene’ssolubilityprovidessignificantfeatureassistinginpurification DOI: 10.4018/JNN.2017010103 .Copyright©2017,IGIGlobal.CopyingordistributinginprintorelectronicformswithoutwrittenpermissionofIGIGlobalisprohibited 28 Journal of Nanotoxicology and Nanomedicine Volume 2 • Issue 1 • January-June 2017 extraction,bioavailability,reactivity,andriskassessmentoffullerenes.Thisinformationisvitaldue toampleapplicationofcarbonnanostructures,suchasC60anditsderivativesindiverseaspectsof nanotechnology,pharmaceuticals,cosmetic,medicinalchemistry,environmentalapplications(Cook ;etal.,2010;Bogatu,&Leszczynska,2016)andmaterialsscience(Gharagheizi&Alamdari,2008 .(Sivaramanetal.,2001 Quantitativestructure-propertyrelationship(QSPR)representsapowerfultoolformodeling andpredictionofphysiochemicalproperties.TheQSPRmethodisdefinedonthefoundationofa .mathematicalalgorithmprovidingarationalbasisforestablishingapredictivecorrelationmodel Apartfromprovidingamathematicalcorrelation,italsoenablestheexplorationofchemicalfeatures encodedwithinparameters(descriptors)(Roy,Kar,&Das,2015a;Toropova,2016).Hence,diverse setofdescriptorsplaysanoteworthyroleintherecognitionaswellasanalysisofthechemicalbasis involvedinastudiedphenomenon.Therefore,reliableQSPRmodelcanoffertimeandcost-effective .measureofC60solubilityvaluesinorganicsolventsintheabsenceofexperimentaldata AseriesofinvestigationforpredictingC60solubilityinorganicsolventsemployingQSPRmodel -hasbeenreportedinthelast12years.Liuetal.(2005)generatedalinearmodelaswellasaleast squaressupportvectormachine(LSSVM)modelforpredictingthesolubilityofC60in128and122 organicsolvents,respectively.Toropovetal.(2007,2009)demonstratedtwokindsofdescriptors methodsforpredictingsolubilityofC60indifferentorganicsolvents.Samedatasetwasusedtobuild one-variablemodeloncewiththeoptimaldescriptorscalculatedwithsimplifiedmolecularinput lineentrysystem(SMILES)(Toropovetal.,2007)andinanotherworkwithInternationalChemical Identifier(InChI)(Toropovetal.,2009)withhighstatisticalresults.Petrovaetal.(2011)depicted successful application of the GA-MLR technique in combination with quantum-chemical and topologicaldescriptorsyieldsreliablefour-variablemodelsfor122organicsolvents.OneGA-MLR .modelwasdevelopedtopredictthefullerenesolubilityin36benzenederivativesbyPourbasheeretal Ghasemietal.(2013)proposedfirst3D-QSARmodelemployingVolSurfbaseddescriptors.(2011) withSPA-SVM(successiveprojectionalgorithm-supportvectormachine)methodtopredictC60 (solubilityin132organicsolventswithacceptablestatisticalresults.Inrecenttime,Xuetal.(2016 proposedaQSPRmodelforpredictingthesolubilityoffullereneC60in156diverseorganicsolvents .withthenormindexes In this regard, we aimed to find simple, predictive, computationally time-efficient and mechanisticallyinterpretablemodeltopredictthesolubilityofC60inthesamesetoforganicsolvents consideredbyXuetal.(2016).Inaddition,thestudyintendstoestimatepredictivepotentialofthe simple1Dand2DdescriptorstomodelthesolubilityofthefullereneC60inalargenumberoforganic solvents. 2. MATeRIALS ANd MeTHODS 2.1. data Set Theexperimentalsolubility(S)dataofC60in156organicsolvents(Table1)werecollectedfrom twodatasets:BeckandMándi(1997)andSemenovetal.(2010).Asthelogarithmicvaluesofmolar fractionscorrespondedtothefreeenergychangesinthesolvationprocess,theunitofsolubilitywas .(consideredaslogS,insteadofweightunits(e.g.,mg/mL 29 Journal of Nanotoxicology and Nanomedicine Volume 2 • Issue 1 • January-June 2017 2.2. descriptors Calculation SolventstructureswerepreparedinHyperChem8softwarepackage(HyperChem(TM))andsaved as.molextensionfile.Thereafter,DRAGON6(DRAGON,TALETEsrl,Italy)softwareemployed togenerateapoolofdescriptorstocorrelatewithlogSfollowedbyfindingbestfeatureswhich areresponsibleforC60solubilityinorganicsolvents.Atotalof319descriptorsgeneratedfrom constitutionalindices,topologicalindices,walk-pathcount,connectivityindices,functionalgroup counts,ETAindices,Atom-centeredfragments,Atom-typeE-stateindices&molecularproperties .havebeenconsidered.Detailsaboutthedescriptorsisdiscussedinthefollowinglink:http://www talete.mi.it/products/dragon_molecular_descriptor_list.pdf 2.3. Model development TheinitialdatasetwasdividedintotrainingandtestsetbasedonsortedexperimentalpropertylogS responsevaluefromlowertohigher.Then,every2ndmoleculefromthefoursolventsconsidered ,inthetestsetfromthesortedcolumnandtheprocesscarriedoutforthewholedataset.Therefore ,datasetdividedinto3:1ratiowith117and39solventsinthetrainingandtestset,respectively.Then geneticalgorithm(GA)wasemployedasthedescriptorselectionstatisticaltoolimplementedinthe GeneticAlgorithm1.4softwarepackage(http://teqip.jdvu.ac.in/QSAR_Tools/).WeappliedGA toselectonlythebestcombinationsofdescriptorsforbuildingmodelswiththehighestpredictive powerofsolubility.Thenthemultiplelinearregression(MLR)analysiswasperformedbyMLR PlusValidationGUI1.2software(http://teqip.jdvu.ac.in/QSAR_Tools/),followedbyvalidationof themodelusingthetestsetcompounds.Overall,thecombinedGA-MLRtechniquewasutilizedto selecttheappropriatedescriptorsandtogeneratedifferentQSPRmodelsselectingthebestmodels .withvariablesintherangefrom1to6 2.4. Model Validation Asetofstringentstatisticalmetricswereutilizedtomakesurethefitnessofthein silicomodels throughinternalandexternalvalidationmethodologies.Thegoodness-of-fitoftheequationwas :checkedbyregressioncoefficient(R2),aswellasbyusingthefollowinginternalvalidationmetrics 2 2 2 theleave-one-outcrossvalidation(Q LOO),andexternalvalidationmetric(R pred).Therm metrics Royetal.,2012;Roy&Kar,2015a),GolbraikhandTropsha’scriteriawerealsocheckedforeach) model(Roy&Kar,2015a).Wealsocheckedthepredictionqualityofallthemodelsintermsofthe
Details
-
File Typepdf
-
Upload Time-
-
Content LanguagesEnglish
-
Upload UserAnonymous/Not logged-in
-
File Pages16 Page
-
File Size-