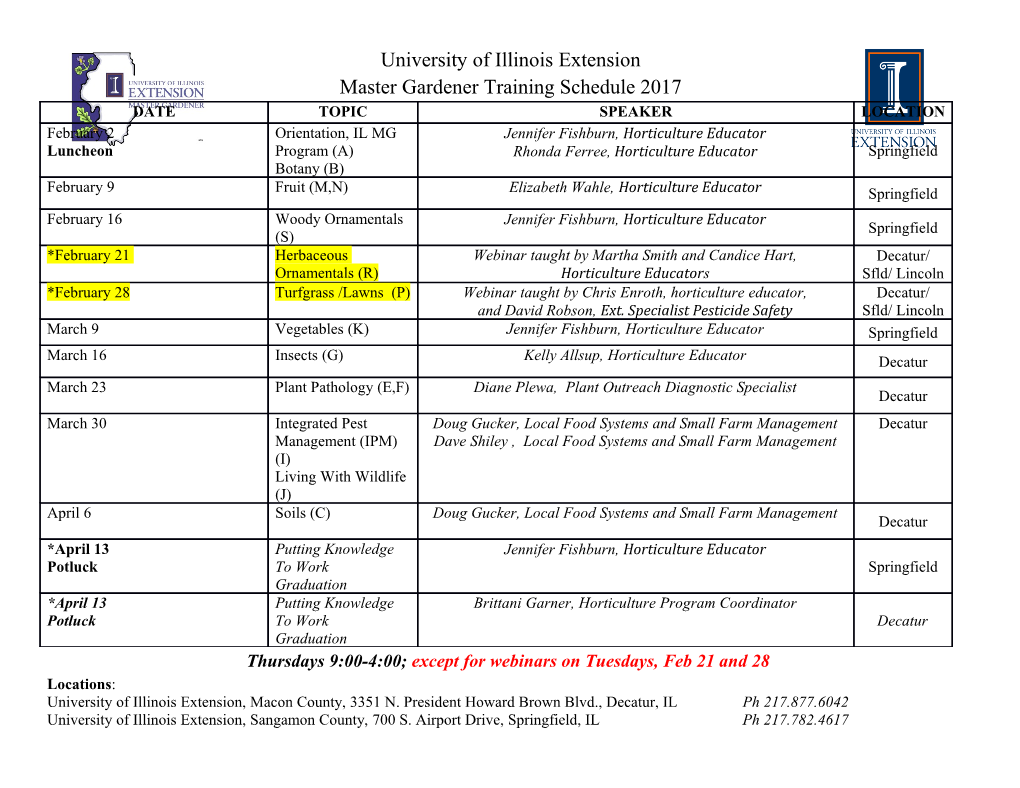
Deleted: ,*, Opportunities and obstacles for deep learning in Formatted ... [1] Formatted ... [2] biology and medicine Deleted: Xie8, Formatted A DOI-citable preprint of this manuscript is available at https://doi.org/10.1101/142760. ... [3] Deleted: Rosen9, This manuscript was automatically generated from greenelab/deep-review@b3b57d3 on January 19, 2018. Formatted ... [4] Deleted: Lengerich10, Authors Formatted ... [5] Deleted: Israeli11, 1, 2 3 Travers Ching , Daniel S. Himmelstein , Brett K. Beaulieu-Jones , Alexandr A. 4 5 2 6 7 Formatted ... [6] Kalinin , Brian T. Do , Gregory P. Way , Enrico Ferrero , Paul-Michael Agapow , Michael 12 Zietz2, Michael M. Hoffman8,9,10, Wei Xie11, Gail L. Rosen12, Benjamin J. Deleted: Lanchantin , Lengerich13, Johnny Israeli14, Jack Lanchantin15, Stephen Woloszynek12, Anne E. Formatted ... [7] Carpenter16, Avanti Shrikumar17, Jinbo Xu18, Evan M. Cofer19,20, Christopher A. Deleted: Woloszynek9, Lavender21, Srinivas C. Turaga22, Amr M. Alexandari17, Zhiyong Lu23, David J. Formatted ... [8] Harris24, Dave DeCaprio25, Yanjun Qi15, Anshul Kundaje17,26, Yifan Peng23, Laura K. Deleted: Carpenter13, Wiley27, Marwin H.S. Segler28, Simina M. Boca29, S. Joshua Swamidass30, Austin Huang31, Anthony Gitter32,33,†, Casey S. Greene2,† Formatted ... [9] Deleted: Shrikumar14, — Author order was determined with a randomized algorithm Formatted ... [10] † — To whom correspondence should be addressed: [email protected] (A.G.) and Deleted: Xu15, [email protected] (C.S.G.) Formatted ... [11] 1. Molecular Biosciences and Bioengineering Graduate Program, University of Hawaii at Manoa, Honolulu, HI Deleted: Cofer16, 2. Department of Systems Pharmacology and Translational Therapeutics, Perelman School of Medicine, University of Formatted Pennsylvania, Philadelphia, PA ... [12] 3. Genomics and Computational Biology Graduate Group, Perelman School of Medicine, University of Pennsylvania, Deleted: Harris17, Philadelphia, PA Formatted 4. Department of Computational Medicine and Bioinformatics, University of Michigan Medical School, Ann Arbor, MI ... [13] 5. Harvard Medical School, Boston, MA Deleted: DeCaprio18, 6. Computational Biology and Stats, Target Sciences, GlaxoSmithKline, Stevenage, United Kingdom Formatted 7. Data Science Institute, Imperial College London, London, United Kingdom ... [14] 8. Princess Margaret Cancer Centre, Toronto, ON, Canada Deleted: Qi12, 9. Department of Medical Biophysics, Toronto, ON, Canada Formatted 10. Department of Computer Science, Toronto, ON, Canada ... [15] 11. Electrical Engineering and Computer Science, Vanderbilt University, Nashville, TN Deleted: Kundaje19, 12. Ecological and Evolutionary Signal-processing and Informatics Laboratory, Department of Electrical and Computer Peng20, Engineering, Drexel University, Philadelphia, PA Deleted: 13. Computational Biology Department, School of Computer Science, Carnegie Mellon University, Pittsburgh, PA Formatted ... [16] 14. Biophysics Program, Stanford University, Stanford, CA 15. Department of Computer Science, University of Virginia, Charlottesville, VA Formatted ... [17] 16. Imaging Platform, Broad Institute of Harvard and MIT, Cambridge, MA Deleted: Wiley21, 17. Department of Computer Science, Stanford University, Stanford, CA 18. Toyota Technological Institute at Chicago, Chicago, IL Formatted ... [18] 19. Department of Computer Science, Trinity University, San Antonio, TX Deleted: Segler22, 20. Lewis-Sigler Institute for Integrative Genomics, Princeton University, Princeton, NJ 21. Integrative Bioinformatics, National Institute of Environmental Health Sciences, National Institutes of Health, Formatted ... [19] Research Triangle Park, NC Deleted: Gitter23,†, 22. Howard Hughes Medical Institute, Janelia Research Campus, Ashburn, VA 23. National Center for Biotechnology Information and National Library of Medicine, National Institutes of Health, Formatted ... [20] * … Bethesda, MD Deleted: — Author order was determined with a... [21] 24. Department of Wildlife Ecology and Conservation, University of Florida, Gainesville, FL 25. ClosedLoop.ai, Austin, TX Moved (insertion) [1] 26. Department of Genetics, Stanford University, Stanford, CA Deleted: and Department of Computer Science 27. Moved up [1]: National Center for Biotechnology 28. Division of Biomedical Informatics and Personalized Medicine, University of Colorado School of Medicine, Aurora, Information and National Library of Medicine, CO National Institutes of Health, Bethesda, MD 29. Institute of Organic Chemistry, Westfälische Wilhelms-Universität Münster, Münster, Germany 30. Innovation Center for Biomedical Informatics, Georgetown University Medical Center, Washington, DC 31. Department of Pathology and Immunology, Washington University in Saint Louis, Saint Louis, MO 32. Department of Medicine, Brown University, Providence, RI 33. Department of Biostatistics and Medical Informatics, University of Wisconsin-Madison, Madison, WI Deleted: and 34. Morgridge Institute for Research, Madison, WI Abstract Deep learning, which describes a class of machine learning algorithms, has recently showed impressive results across a variety of domains. Biology and medicine are data rich, but the data are complex and often ill-understood. Problems of this nature may be particularly well-suited to deep learning techniques. We examine applications of deep learning to a variety of biomedical problems— Deleted: -- patient classification, fundamental biological processes, and treatment of patients—and discuss Deleted: -- to predict whether deep learning will transform these tasks or if the biomedical sphere poses unique challenges. We find that deep learning has yet to revolutionize or definitively resolve any of these problems, but promising advances have been made on the prior state of the art. Even when improvement over a previous baseline has been modest, we have seen signs that deep learning methods may speed or aid human investigation. More work is needed to address concerns related to interpretability and how to best model each problem. Furthermore, the limited amount of labeled data for training presents problems in some domains, as do legal and privacy constraints on work with Deleted: can sensitive health records. Nonetheless, we foresee deep learning powering changes at both bench Deleted: the and bedside with the potential to transform several areas of biology and medicine. Introduction to deep learning Biology and medicine are rapidly becoming data-intensive. A recent comparison of genomics with social media, online videos, and other data-intensive disciplines suggests that genomics alone will equal or surpass other fields in data generation and analysis within the next decade [1]. The volume and complexity of these data present new opportunities, but also pose new challenges. Automated algorithms that extract meaningful patterns could lead to actionable knowledge and change how we develop treatments, categorize patients, or study diseases, all within privacy-critical environments. The term deep learning has come to refer to a collection of new techniques that, together, have demonstrated breakthrough gains over existing best-in-class machine learning algorithms across several fields. For example, over the past five years these methods have revolutionized image classification and speech recognition due to their flexibility and high accuracy [2]. More recently, deep learning algorithms have shown promise in fields as diverse as high-energy physics [3], dermatology [4], and translation among written languages [5]. Across fields, “off-the-shelf” Deleted: " implementations of these algorithms have produced comparable or higher accuracy than previous Deleted: " best-in-class methods that required years of extensive customization, and specialized implementations are now being used at industrial scales. Deep learning approaches grew from research in neural networks, which were first proposed in 1943 [6] as a model for how our brains process information. The history of neural networks is interesting in its own right [7]. In neural networks, inputs are fed into the input layer, which feeds into Deleted: a hidden one or more hidden layers, which eventually link to an output layer. A layer consists of a set of Deleted: produce nodes, sometimes called “features” or “units,” which are connected via edges to the immediately earlier and the immediately deeper layers. In some special neural network architectures, nodes can connect to themselves with a delay. The nodes of the input layer generally consist of the variables being measured in the dataset of interest—for example, each node could represent the intensity value of a specific pixel in an image or the expression level of a gene in a specific transcriptomic experiment. The neural networks used for deep learning have multiple hidden layers. Each layer essentially performs feature construction for the layers before it. The training process used often allows layers deeper in the network to contribute to the refinement of earlier layers. For this reason, these algorithms can automatically engineer features that are suitable for many tasks and customize those features for one or more specific tasks. Deep learning does many of the same things as more familiar machine learning approaches. In Deleted: Like a clustering algorithm, it particular, deep learning approaches can be used both in supervised applications—where the goal is Deleted: build features that describe recurrent to accurately predict one or more labels or outcomes
Details
-
File Typepdf
-
Upload Time-
-
Content LanguagesEnglish
-
Upload UserAnonymous/Not logged-in
-
File Pages201 Page
-
File Size-