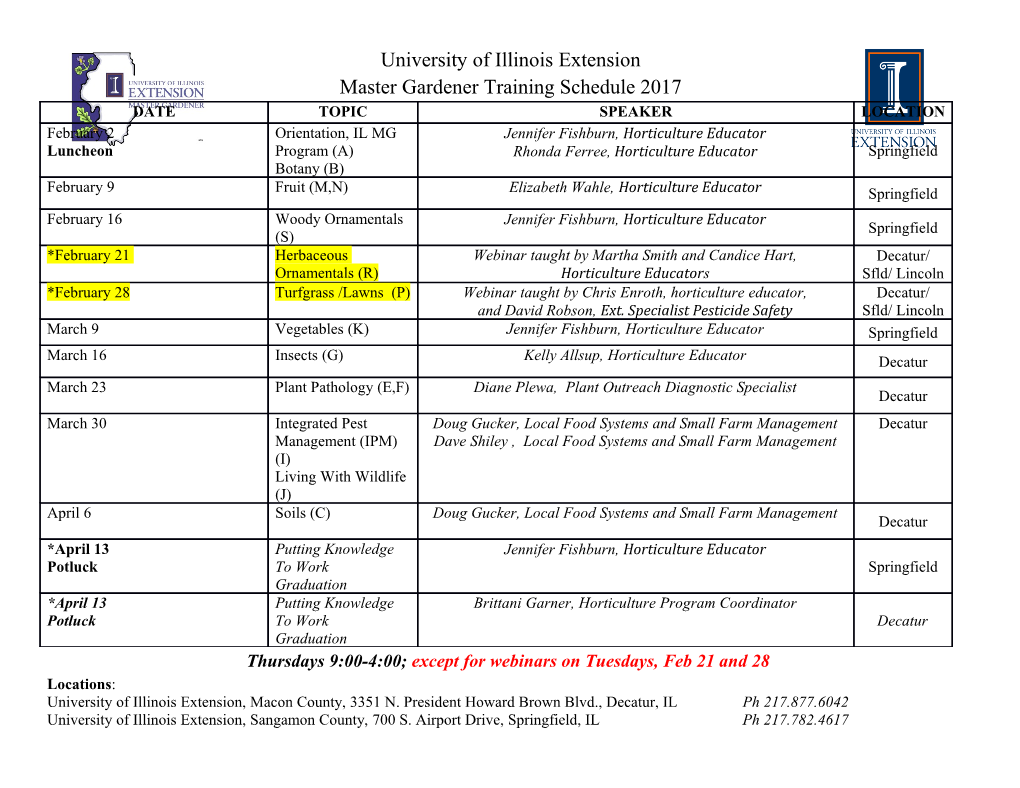
View metadata, citation and similar papers at core.ac.uk brought to you by CORE provided by OAR@UM Forecasting Using Non-Linear Techniques In Time Series Analysis: An Overview Of Techniques and Main Issues Michel Camilleri Department of Computer Science and AI, University of Malta Abstract. The development of techniques in non linear time series analysis has emerged from its time series background and developed over the last few decades into a range of techniques which aim to fill a gap in the ability to model and forecast certain types of data sets such a chaotic determinate systems. These systems are found in many diverse areas of natural and human spheres. This study outlines the background within which these techniques developed, the fundamental elements on which they are based and details some of the predictive techniques. This study aims to provide some insight into their mechanisms and their potential. 1 Introduction: The Need for Forecasting No one knows the future, but there is a lot of benefit to be derived from attempting to form a picture of what the future could be - even if it is imprecise. It is one of the task most decision makers are expected asked to do - from the hunter-farmers of prehistoric age to the stock analysts of today. [1] Almost all managerial decisions are based on forecasts. Every decision becomes operational at some point in the future, so it should be based on forecasts of future conditions. A significant amount of research has been carried out using time series techniques in such di- verse fields as [13] modelling and predicting solar sun spot activity, [5] analysis of Ethernet traffic, Business cycles, [3] Intelligent analysis of clinical time series in Diabetes Mellitus. Non linear tech- niques are emerging as powerful tool in the hands of the analyst to leverage patterns of behaviour underlying hiding dynamical systems - which may elude analysis using other traditional techniques. [1] A model is an external and explicit representation of a part of reality as it is seen by individuals who wish to use this model to understand, change, manage and control that part of reality. Analysts try to model the system or process which is being studied in a variety of ways. Regression Is the study of relationships among variables, a principle purpose of which is to predict, estimate the value of one variable from known or assumed values of other variables related to it. Using one predictor it is called simple linear regression. Using two or more predictors, multiple regression analysis is employed A Scatter diagram is a graphical representation of the pairs of data to gain an overall view of the problem — is there an apparent relationship? Direct, inverse? If the points lie within a band described by parallel lines we can say there is a linear relationship between the pair of x and y values. If the rate of change is generally not constant then the relationship is curvilinear. Linear Models: One would determine if a linear relationship exists between t and y and then attempt to produce a linear equation stating y as a function of x in the form of y = a + bx + e 20 Michel Camilleri where a is the intercept, b is the slope and e is the error term accounting for variables that affect y but are not included as predictors, and/or otherwise unpredictable and uncontrollable factors. Least squares method: To predict the mean y-value for a given x-value, we need a line which passes through the mean value of both x and y and which minimizes the sum of the distance between each of the points and the predictive line — the best fit to the sample data. The least squares method achieves this by calculating the minimum averaged squared deviations between sample y points and the estimated line. A procedure is used for finding values of a and b which reduces to the solution of simultaneous linear equations. Shortcut formula have been developed as an alternative. Solution methods: Techniques of matrix algebra can be manually employed to solve simultaneous linear equations especially useful when there are more than two equations and two unknowns. Sev- eral computer packages using matrix algebra to solve simultaneous equations are widely available and can be utilised to relieve the computational problems - can solve both linear and polynomial equations Variables of interest: To make predictions or estimates we must identify the effective predictors of the variable of interest which variables are important indicators? And can be measured at the least cost? Which carry only a little information? Which are redundant? 2 Time Series Analysis [10] A time series is a set of numbers that measures the status of some activity over time. It is the historical record of some activity, with measurements taken at equally (not always) spaced intervals with a consistency in the activity and the method of measurement [1]. Time Series Analysis comprises methods for the quantitative characterization, the manipulation, and the understanding of time series data and the underlying systems. Time Series Forecasting Approaches There are two basic approaches to forecasting time series: the self-projecting time series and the cause-and-effect approach. It is possible that both approaches still lead to the creation of accurate and useful forecasts. The first is often the more difficult to implement and validate than the second. Self-projecting time series: This approach uses only the time series data of the activity to be forecast to generate forecasts. The basic idea behind self-projecting time series forecasting model is to find a mathematical formula that will approximately generate the historical patterns in a time series. Linear correlations in time are the most common and best studied sources of predictability. Linear statistical methods assume a random nature of the data since the underlying auto-regressive and moving average models are stochastic. Auto-regressive models and moving average models are stochastic due to the assumed random nature of the data [10]. The randomness prevents one from making any precise predictions, even with an absolute knowledge of the present state, but the strong correlation assures one that the next observation will be given by approximately by a linear combination of the preceding observations, except for additive noise ie: Pm sn+1 = a0 + j=1 ajsn−m+j Autoregressive models is one of a group of linear prediction formulae that attempt to predict an output of a system based on the previous outputs and inputs. Autoregressive processes as their name implies, regress on themselves. The current value of the series is a linear combination of the Forecasting Using Non-Linear Techniques In Time Series Analysis 21 p most recent past values of itself plus an error term which incorporates everything new in the series at time t that is not explained by the past values. An autoregressive model AR is a model which depends only on the previous outputs of the system is called. A moving average model MA. Is a model which depends only on the inputs to the system. AN autoregressive-moving-average model ARMA is a model based on both inputs and output. Deterministic dynamical systems: Pure dynamical systems are described either by discrete time maps where: xn+1 = f(xn)(n is a discrete unit of time) Or by first order ordinary differential equations: dx(t0)/dt = f(t, x(t)) (t is a real value of time) Both are descriptions of the fact that all future states of such nonlinear deterministic dynamical systems are unambiguously determined by specifying its present state at some time n (or t). Thus there also exists a deterministic forecasting function. Nonlinear deterministic dynamical systems: Another type of correlation in time is present in non- linear deterministic dynamical systems. The underlying systems would have a deterministic nature but display irregularities. Chaos hence is an alternative source for unpredictability and irregularity in observed data, an alternative with respect to stochastic inputs. These irregularities in the measurements arise from processes which co-exist with the system under study or are due to inaccuracies (noise) in the measurement process itself. Any irregularities in the knowledge of the present state will evolve over time, in in the case of a chaotic system, will grow exponentially. However the uncertainty is amplified only at a finite rate and one can still hope to make reasonable short term forecasts. Nonlinear prediction techniques exploit correlations over time visible only by using non-linear statistics. 3 Non-Linear Time Series Analysis [11] The pioneering work by Packard et al. and the seminal paper by F. Takens stimulated, about 20 years ago, a new approach towards complex dynamical phenomena, nowadays called nonlinear time series analysis. Extensions towards nonlinear stochastic models and towards nonlinear statistical analysis are manifold and have been considered within various frameworks. Stationarity: [10] A signal is called stationary if all transition probabilities from one state of the system to another are independent of time within the observation period. All relevant parameters have to be kept constant and that phenomena belonging to the dynamics are contained in the time series sufficiently frequently so that the probabilities or other rule scan be inferred properly. Unpredictability: [10] of the future is the most striking feature of chaos despite a deterministic evolution. This unpredicatbiity is a consequence of the inherent instability of the solutions reflected by what is called sensitive dependence on initial conditions. The tiny deviations between the intial conditions of all the trajectories are blown up in a few time steps and every unobserved detail of the state at time zero is important for the precise path of the trajectory in state space.
Details
-
File Typepdf
-
Upload Time-
-
Content LanguagesEnglish
-
Upload UserAnonymous/Not logged-in
-
File Pages10 Page
-
File Size-