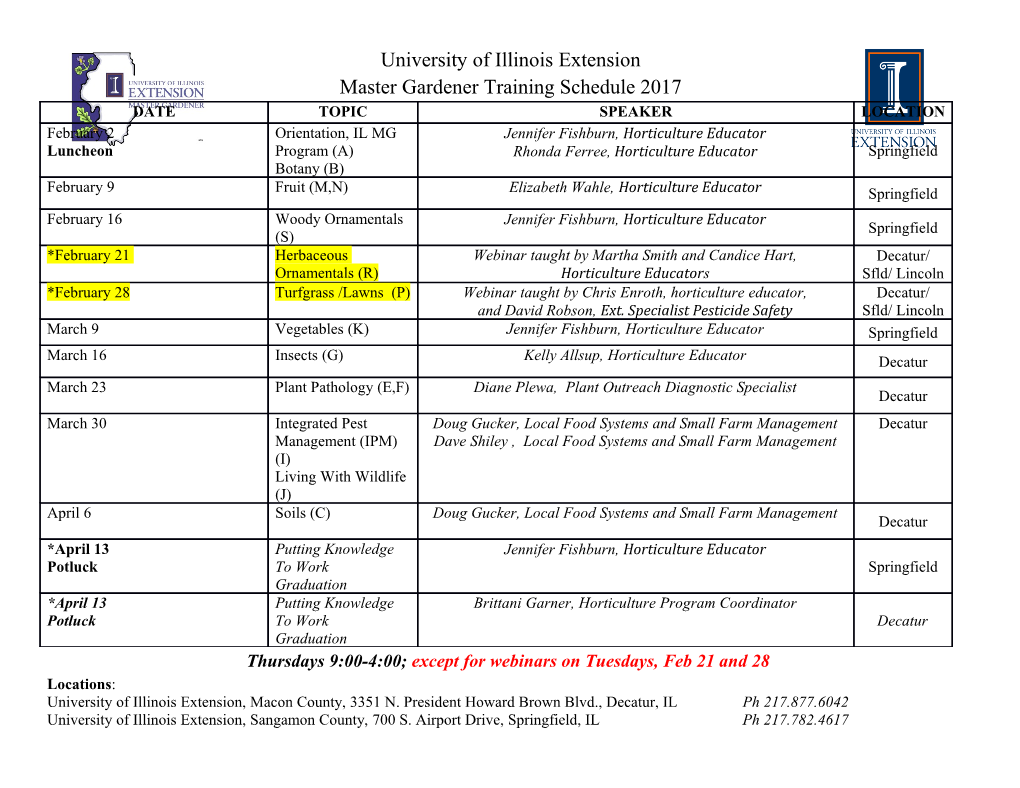
View metadata, citation and similar papers at core.ac.uk brought to you by CORE provided by Elsevier - Publisher Connector Journal of Computational and Applied Mathematics 235 (2011) 2662–2669 Contents lists available at ScienceDirect Journal of Computational and Applied Mathematics journal homepage: www.elsevier.com/locate/cam On a class of inverse quadratic eigenvalue problem Yongxin Yuan a,∗, Hua Dai b a School of Mathematics and Physics, Jiangsu University of Science and Technology, Zhenjiang 212003, PR China b Department of Mathematics, Nanjing University of Aeronautics and Astronautics, Nanjing 210016, PR China article info a b s t r a c t Article history: In this paper, we first give the representation of the general solution of the following inverse Received 25 September 2009 monic quadratic eigenvalue problem (IMQEP): given matrices Λ D diagfλ1; : : : ; λpg 2 Received in revised form 3 December 2009 p×p n×p C , λi 6D λj for i 6D j, i; j D 1;:::; p, X DTx1;:::; xpU 2 C , rank.X/ D p, and N both Λ and X are closed under complex conjugation in the sense that λ2j D λ2j−1 2 C, MSC: n n x2j D xN2j−1 2 C for j D 1;:::; l, and λk 2 R, xk 2 R for k D 2l C 1;:::; p, 65F18 2 C C D 15A24 find real-valued symmetric matrices D and K such that XΛ DXΛ KX 0: Then Q Q n×n O O we consider a best approximation problem: given D; K 2 R , find .D; K/ 2 SDK such Keywords: O O Q Q Q Q that k.D; K/ − .D; K/kW D min.D;K/2 k.D; K/ − .D; K/kW ; where k · kW is a weighted Quadratic eigenvalue problem SDK Frobenius norm and SDK is the solution set of IMQEP. We show that the best approximation Inverse eigenvalue problem O O Monic quadratic pencil solution .D; K/ is unique and derive an explicit formula for it. Partially prescribed spectral information ' 2010 Elsevier B.V. All rights reserved. Best approximation 1. Introduction In a recent treatise, Tisseur and Meerbergen [1] have surveyed many applications, mathematical properties, and a variety of numerical techniques for the so-called quadratic eigenvalue problem (QEP). The problem concerns, given n × n complex matrices M; D and K, find scalars λ and nonzero vectors x such that Q (λ)x D 0; (1.1) where Q (λ) VD Q (λI M; D; K/ D λ2M C λD C K (1.2) is called a quadratic pencil defined by M; D and K. The scalars λ and the corresponding nonzero vectors x are called, respectively, eigenvalues and eigenvectors of the pencil. The QEP is currently receiving much attention because of its extensive applications in areas such as applied mechanics, electrical oscillation, vibro-acoustics, fluid mechanics, gyroscopic systems, and signal processing. It is known that the QEP has 2n finite eigenvalues over the complex field, provided that the leading matrix coefficient M is nonsingular. The ``direct'' problem is, of course, to find the eigenvalues and eigenvectors when the coefficient matrices M; D and K are given, and an inverse QEP is to determine the matrix coefficients M; D and K from a prescribed set of eigenvalues and eigenvectors. In most of the applications involving (1.1), specifications of the underlying physical system are embedded in the matrix coefficients M; D and K while the resulting bearing of the system usually can be interpreted via its eigenvalues and eigenvectors. A direct QEP, therefore, is meant generally to induce the dynamical behavior from given physical parameters. ∗ Corresponding author. Tel.: +86 0511 4401748. E-mail address: [email protected] (Y. Yuan). 0377-0427/$ – see front matter ' 2010 Elsevier B.V. All rights reserved. doi:10.1016/j.cam.2010.11.017 Y. Yuan, H. Dai / Journal of Computational and Applied Mathematics 235 (2011) 2662–2669 2663 The inverse QEP reverse the line of induction. It is meant to construct the physical parameters from a given or desired behavior. The inverse problem is equally important in practice as the direct problem. Matrix coefficients in QEPs arising in practice often maintain some additional properties. A typical case is that each of the matrix coefficients is of certain symmetry and that M is symmetric positive definite. Thus, for practicality, inverse QEPs should be solved with these additional constraints on the matrix coefficients in mind. An inverse QEP of interest here is that when the leading matrix coefficient M is known and fixed, only D and K are to be determined. Our study in this paper stems from the speculation that the notion of the inverse QEP has the potential of leading to an important modification tool for model updating, model tuning, and model correction [2–5], when compared with an analytical model. Consider the fact that if M D LLT denotes the Cholesky decomposition of M, then Q (λ)x D 0 , QQ (λ)(LT x/ D 0; (1.3) where Q 2 −1 −T −1 −T Q (λ) VD λ In C λL DL C L KL : (1.4) Thus, without loss of generality, we may assume that the given matrix M in our inverse problem is the n × n identity matrix In. For this reason, the inverse problem we are dealing with will be called an inverse monic quadratic eigenvalue problem (IMQEP). This paper is mainly concerned with solving the following two problems. Problem I (IMQEP). Given a pair of matrices .Λ; X/ in the form p×p Λ D diagfλ1; : : : ; λpg 2 C (1.5) and n×p X DTx1;:::; xpU 2 C ; (1.6) where diagonal elements of Λ are all distinct, X is of full column rank p, and both Λ and X are closed under complex N n n conjugation in the sense that λ2j D λ2j−1 2 C; x2j D xN2j−1 2 C for j D 1;:::; l, and λk 2 R; xk 2 R for k D 2l C 1;:::; p, find real-valued and symmetric matrices D and K that satisfy the equation XΛ2 C DXΛ C KX D 0: (1.7) In other words, each pair (λt ; xt /; t D 1;:::; p, is an eigenpair of the monic quadratic pencil 2 Q (λ) D λ In C λD C K: Q Q n×n O O Problem II (Approximation Problem). Given D; K 2 R , find .D; K/ 2 SDK such that O O Q Q Q Q k.D; K/ − .D; K/kW D min k.D; K/ − .D; K/kW ; (1.8) .D;K/2SDK where k · kW is the weighted Frobenius norm, and SDK is the solution set of Problem I. In Section 2, we show that Problem I is always solvable, and the representation of the solution set of Problem I, denoted by SDK , is presented. In Section 3, we prove that Problem II is uniquely solvable, and the expression of the unique solution .DO ; KO / is given. In Section 4, a numerical algorithm to acquire the best approximation solution under a weighted Frobenius norm sense is described and two numerical examples are provided. Some concluding remarks are given in Section 5. In this paper, we shall adopt the following notation. Let Cm×n; Rm×n denote the set of all m×n complex and real matrices, × respectively. ORn n denotes the set of all orthogonal matrices in Rn×n. Capital letters A; B; C;::: , denote matrices, lower case letters denote column vectors, Greek letters denote scalars, αN denotes the conjugate of the complex number α; AT denotes m×n the transpose of the matrix A, and k · k stands for the matrix Frobenius norm. Given two matrices A DTaijU 2 R and m×n m×n B DTbijU 2 R ; A ∗ B represents the Hadamard product of the matrices A and B, that is, A ∗ B DTaijbijU 2 R . We write A > 0 .A ≥ 0/ if A is real symmetric positive definite (positive semi-definite). 2. Solving Problem I Let αi D Re(λi/ (the real part of the complex number λi), 0 < βi D Im (λi/ (the imaginary part of the complex number λi), yi D Re.xi/; zi D Im .xi/ for i D 1; 3;:::; 2l − 1 and [ ] [ ] Q α1 β1 α2l−1 β2l−1 p×p Λ D diag ;:::; ; λ2lC1; : : : ; λp 2 R ; (2.1) −β1 α1 −β2l−1 α2l−1 Q n×p X D y1; z1;:::; y2l−1; z2l−1; x2lC1;:::; xp 2 R : (2.2) 2664 Y. Yuan, H. Dai / Journal of Computational and Applied Mathematics 235 (2011) 2662–2669 Then, the equation (1.7) is equivalent to the equation XQ ΛQ 2 C DXQ ΛQ C KXQ D 0: (2.3) Since rank.X/ D rank.XQ / D p, the singular value decomposition (SVD) of XQ is of the form [Σ] XQ D U Q T D U ΣQ T ; (2.4) 0 1 n×n n×p p×p where U DTU1; U2U 2 OR with U1 2 R ; Q 2 OR , and Σ D diagfσ1; : : : ; σpg > 0. Now, based on the SVD of XQ , for an arbitrary matrix A 2 Rn×n, we can define a norm termed weighted Frobenius norm that kAkW VD kWAW k; (2.5) h i D Σ 0 T k · k h i VD where W U 0 I U > 0. It is easy to check that W is a norm induced by the inner product A; B 2 T 2 n×n n×n trace.W B W A/ for all matrices A; B 2 R .
Details
-
File Typepdf
-
Upload Time-
-
Content LanguagesEnglish
-
Upload UserAnonymous/Not logged-in
-
File Pages8 Page
-
File Size-