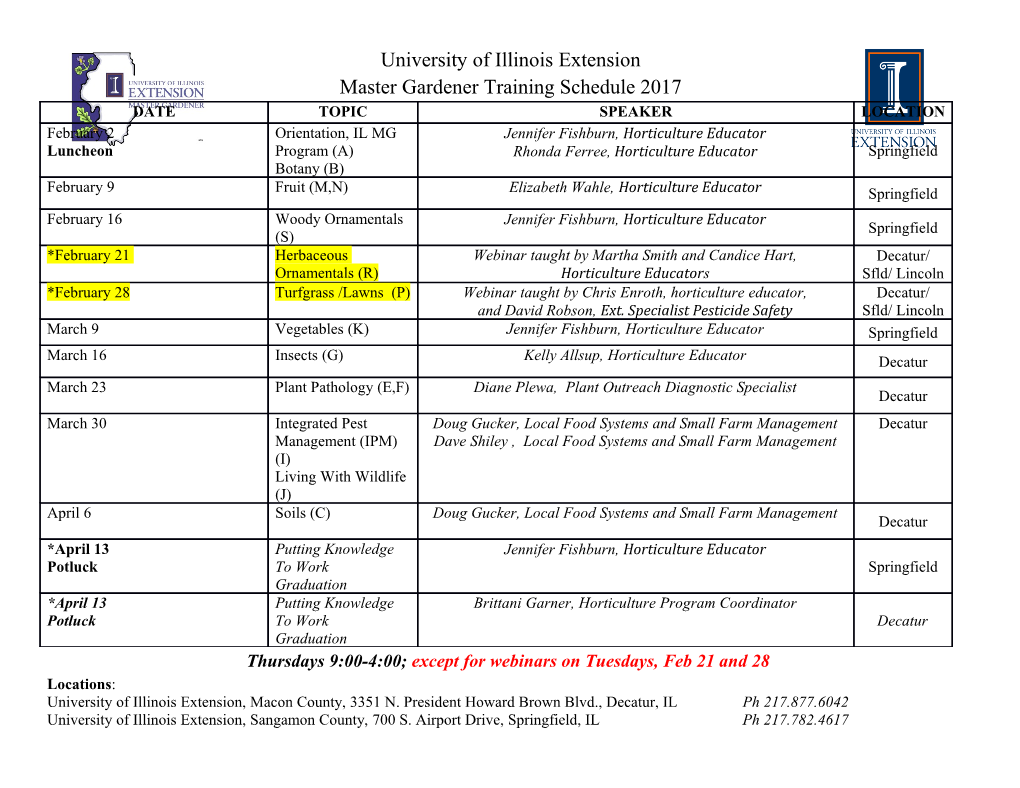
Holmes BMC Bioinformatics (2017) 18:255 DOI 10.1186/s12859-017-1665-1 COMMENTARY Open Access Solving the master equation for Indels Ian H. Holmes Abstract Background: Despite the long-anticipated possibility of putting sequence alignment on the same footing as statistical phylogenetics, theorists have struggled to develop time-dependent evolutionary models for indels that are as tractable as the analogous models for substitution events. Main text: This paper discusses progress in the area of insertion-deletion models, in view of recent work by Ezawa (BMC Bioinformatics 17:304, 2016); (BMC Bioinformatics 17:397, 2016); (BMC Bioinformatics 17:457, 2016) on the calculation of time-dependent gap length distributions in pairwise alignments, and current approaches for extending these approaches from ancestor-descendant pairs to phylogenetic trees. Conclusions: While approximations that use finite-state machines (Pair HMMs and transducers) currently represent the most practical approach to problems such as sequence alignment and phylogeny, more rigorous approaches that work directly with the matrix exponential of the underlying continuous-time Markov chain also show promise, especially in view of recent advances. Keywords: Phylogenetics, Alignment, Indels Background transmission dynamics [28], reconstruct key transmission Models of sequence evolution, formulated as continuous- events [29], and predict future evolutionary trends [30]. time discrete-state Markov chains, are central to statis- There are many other applications; the ones listed above tical phylogenetics and bioinformatics. As descriptions were selected to give some idea of how influential these of the process of nucleotide or amino acid substitu- models have been. tion, their earliest uses were to estimate evolutionary Continuous-time Markov chains describe evolution in distances [1], parameterize sequence alignment algo- astatespace, for example the set of nucleotides rithms [2], and construct phylogenetic trees [3]. Variations ={A,C,G,T}. The stochastic process φ(t) at any given on these models, including extra latent variables, have instant of time, t, takes one of the values in .Letp(t) be a been used to estimate spatial variation in evolutionary vector describing the marginal probability distribution of rates [4, 5]; these patterns of spatial variation have been the process at a single point in time: pi(t) = P(φ(t) = i). used to predict exon structures of protein-coding genes The time-evolution of this vector is governed by a master [6, 7], foldback structure of non-coding RNA genes equation [8, 9], regulatory elements [10], ultra-conserved elements d [11], protein secondary structures [12], and transmem- p(t) = p(t)R (1) brane structures [13]. They are widely used to reconstruct dt ancestral sequences [14–22], a method that is finding where, for i, j ∈ and i = j, Ri,j is the instantaneous increasing application in synthetic biology [16, 20–22]. rate of mutation from state i to state j. For probabilistic Trees built using substitution models used to classify normalization of Eq. 1, it is then required that species [23], predict protein function [24], inform con- R =− R servation efforts [25], or identify novel pathogens [26]. In i,i i,j ∈ = the analysis of rapidly evolving pathogens, these meth- j ,j i ods are used to uncover population histories [27], analyze The probability distribution of this process at equilib- rium is given by the vector π, which must satisfy the Correspondence: [email protected] equation Dept of Bioengineering, University of California, 94720 Berkeley, USA πR = 0 © The Author(s). 2017 Open Access This article is distributed under the terms of the Creative Commons Attribution 4.0 International License (http://creativecommons.org/licenses/by/4.0/), which permits unrestricted use, distribution, and reproduction in any medium, provided you give appropriate credit to the original author(s) and the source, provide a link to the Creative Commons license, and indicate if changes were made. The Creative Commons Public Domain Dedication waiver (http://creativecommons.org/publicdomain/zero/1.0/) applies to the data made available in this article, unless otherwise stated. Holmes BMC Bioinformatics (2017) 18:255 Page 2 of 10 The general solution to Eq. 1 can be written p(t) = As mentioned above, this mathematical approach is p(0)M(t) where M(t) is the matrix exponential fundamental to statistical phylogenetics and many appli- cations in bioinformatics. For small state spaces ,such ( ) = ( ) M t exp Rt (2) as (for example) the 20 amino acids or 61 sense codons, the matrix exponential M(t) in Eq. 2 can be solved exactly Entry Mi,j(t) of this matrix is the probability P(φ(t) = j|φ(0) = i) that, conditional on starting in state i,the and practically by the technique of spectral decomposi- system will after time t be in state j.Itfollows,bydefini- tion (i.e. finding eigenvalues and eigenvectors). Such an tion, that this matrix satisfies the Chapman-Kolmogorov approach informs the Dayhoff PAM matrix. It was also forward equation: solved for certain specific parametric forms of the rate matrix R by Jukes and Cantor [1], Kimura [31], Felsenstein M(t)M(u) = M(t + u) (3) [3], and Hasegawa et al. [32], among others. This approach is used by all likelihood-based phylogenetics tools, such ( ) That is, if Mi,j t is the probability that state i will, after a as RevBayes [33], BEAST [34], RAxML [35], HyPhy [36], ( ) finite time interval t,haveevolvedintostatej,andMj,k u PAML [37], PHYLIP [38], TREE-PUZZLE [39], and XRate is the analogous probability that state j will after time u [40]. Many more bioinformatics tools use the Dayhoff evolve into state k, then summing out j has the expected PAM matrix or other substitution matrix based on an result: underlying master equation of the form Eq. 1. ( ) ( ) = ( + ) ∀ ∈ Mi,j t Mj,k u Mi,k t u i, k Homogeneity, stationarity, and reversibility j∈ There exists a deep literature on Markov chains, to which This is one way of stating the defining property of a this brief survey cannot remotely do justice, but several Markov chain: its lack of historical memory previous to its concepts must be mentioned in order to survey progress current state. Equation 1 is just an instantaneous version in this area. of this equation, and Eq. 3 is the same equation in matrix A Markov chain is time-homogeneous if the elements form. of the rate matrix R in Eq. 1 are themselves independent The conditional likelihood for an ancestor-descendant of time. If a Markov chain is time-homogeneous and is pair can be converted into a phylogenetic likelihood for a known to be in equilibrium at a given time, for example ( ) =π set of extant taxon states S related by a tree T, as follows. p 0 , then (absent any other constraints) it will be in (I assume for convenience that T is a binary tree, though equilibrium at all times; such a chain is referred to as being relaxing this constraint is straightforward.) stationary. To compute the likelihood requires that one first com- The time-scaling of these models is somewhat arbitrary: /κ putes, for every node n in the tree, the probability F (n) if the time parameter t is replaced by a scaled version t , i κ of all observed states at leaf nodes descended from node while the rate matrix R is replaced by R , then the likeli- n, conditioned on node n being in state i. This is given by hood in Eq. 2 is unchanged. For some models, the rate is Felsenstein’s pruning recursion: allowed to vary between sites [4, 5]. A Markov chain is reversible if it satisfies the instanta- ( )( ) ◦ ( )( ) neous detailed balance condition π R =π R ,orits ( )= M tnl F l M tnr F r if n is an internal node with children l, r i i,j j j,i F n π =π (sn) if n is a leaf node in state sn finite-time equivalent iMi,j jMj,i.Thisamountstoa (4) symmetry constraint on the parameter space of the chain (specifically, the matrix S with elements Si,j = πi/πjRi,j where tmn denotes the length of the branch from tree node is symmetric) which has several convenient advantages: m to tree node n. I have used the notation ( j) for the it effectively halves the number of parameters that must unit vector in dimension j,andthesymbol◦ to denote the be determined, it eases some of the matrix manipulations Hadamard product (also known as the pointwise product), (symmetric matrices have real eigenvalues and the algo- defined such that for any two vectors u, v of the same size: rithms to find them are generally more stable), and it (u ◦ v)i =uivi. allows for some convenient manipulations, such as the so- Supposing that node 1 is the root node of the tree, and called pulley principle allowing for arbitrary re-rooting of that the distribution of states at this root node is given by the tree [3]. From another angle, however, these supposed ρ, the likelihood can be written as advantages may be viewed as drawbacks: reversibility is a simplification which ignores some unreversible aspects ( | ρ) =ρ · ( ) P S T, R, F 1 (5) of real data, limits the expressiveness of the model, and where u ·v denotes the scalar product of u and v.Itiscom- makes the root node placement statistically unidentifiable. mon to assume that the root node is at equilibrium, so that Stationarity has similar advantages and drawbacks. If ρ =π. one assumes the process was started at equilibrium, that is Holmes BMC Bioinformatics (2017) 18:255 Page 3 of 10 one less set of parameters to worry about (since the equi- publications by Ezawa [53–55] and Rivas and Eddy [56] librium distribution is implied by the process itself), but have highlighted this problem once again, directly leading it also renders the model less expressive and makes some to the present review.
Details
-
File Typepdf
-
Upload Time-
-
Content LanguagesEnglish
-
Upload UserAnonymous/Not logged-in
-
File Pages10 Page
-
File Size-