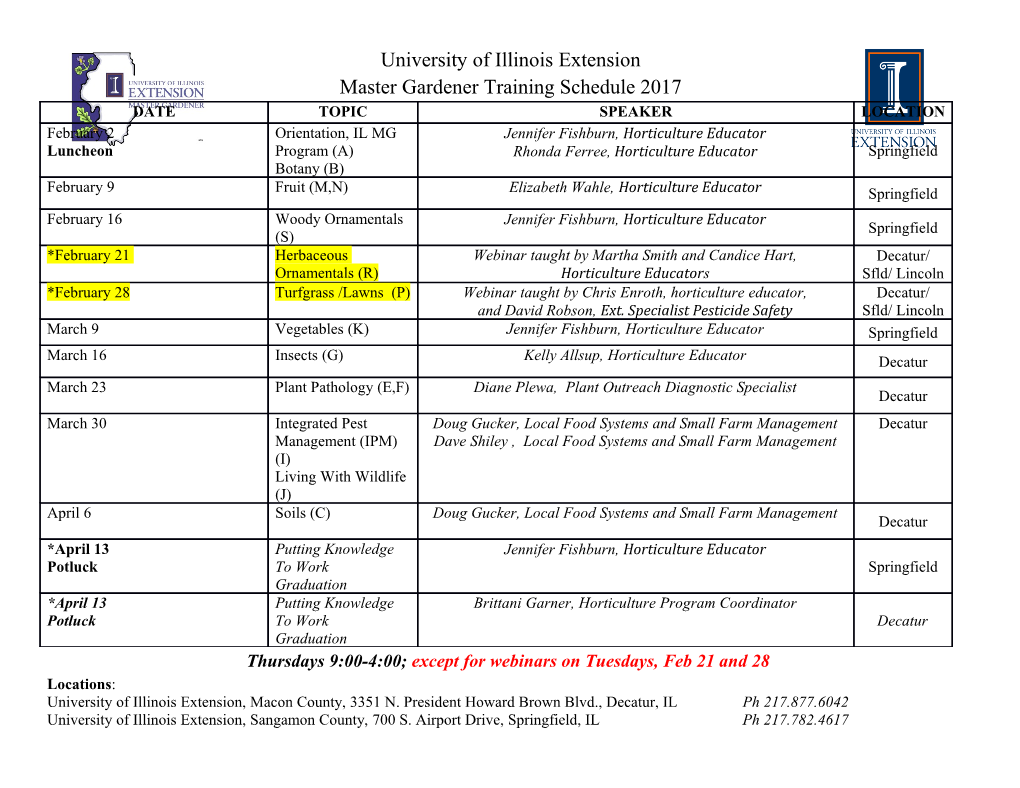
An empirical comparison: autoregressive conditional heteroskedasticity and multi- factor models in asset return predictability by Theodoros Zigkiris [206568] M.Sc. Tilburg University 2016 A thesis submitted in partial fulfillment of the requirements of the degree of Master of Science in Econometrics and Mathematical Economics Tilburg School of Economics and Management Tilburg University Supervisor Prof. dr. B. Melenberg Second reader Dr. P. Cizek Date: February 29, 2016 Abstract In time series analyses of asset returns, the volatility appears to be time-dependent. Several approaches have been proposed in the academic literature in order to predict future stock returns and measure their risk as well. This thesis focuses on the performance of the standard GARCH(1,1) and EGARCH(1,1) models, in comparison with two alternative linear factor models in predicting future stock returns. The linear models are the three-factor model of Fama and French and a macroeconomic four-factor model whose factors are the CPI inflation rate, USD/GBP exchange rates, and the price of crude oil barrel in UK. The final results after the forecast show that the standard GARCH(1,1) as well as EGARCH(1,1) models perform better than the other two multi-factor models in predicting future stock returns. 2 Table of Contents Abstract .......................................................................................................................... 2 1. Introduction ............................................................................................................... 4 2. Literature .................................................................................................................... 7 3.Data description ....................................................................................................... 10 3.1 Sample statistics .................................................................................................. 11 4. Models ...................................................................................................................... 20 4.1 Three-factor model .............................................................................................. 20 4.2 GARCH(1,1) model .............................................................................................. 23 4.3 Four-factor model ................................................................................................ 27 4.4 EGARCH(1,1) model ........................................................................................... 29 5. Comparison of the models ..................................................................................... 34 5.1 Forecast ............................................................................................................... 34 5.2 Vector Autoregressive Models ............................................................................. 41 6. Conclusions ............................................................................................................. 51 References ................................................................................................................... 53 7. Appendix .................................................................................................................. 55 3 1. Introduction There have been a great variety of researches in order to analyse the relationship between risk factors (market, value, size, momentum, etc.) and the cross-section of stock returns. Are there any arbitrage opportunities that financial investors can exploit? Do investors believe in the efficient market hypothesis? Are investors able to explain and more importantly predict future stock returns based on the public information available? This topic at least goes back to Dow Jones1 around 1900 who tried to exploit predictability in asset returns. Fama (1970) suggested that “a market in which prices always “fully reflect” available information is called "efficient””. Some years later, Black (1972) suggests the Capital Asset Pricing Model CAPM which can be considered as an extension of EMH, in which a market factor is added in the model in order to determine the stocks’ prices. Later on, Fama and French (1992, 1993) propose the three-factor model, so as to understand better the portfolio performance and estimate future returns. Engle (1982) proposed the autoregressive conditional heteroskedasticity (ARCH) models for time series, where volatility is allowed to be time-dependent. A lot of different approaches have been proposed in the already existing literature as far as prediction of future returns is concerned. This thesis is aiming to give answers to the following research questions: Which one of the four models – three-factor model of Fama and French, the standard GARCH(1,1), four-factor model and EGARCH(1,1) - can give the most efficient and accurate forecasts as far as future returns are concerned? Which factors should we include in the multi-factor model so that it describes the data better? The models are chosen due to their structures, which can capture some of the stylized facts (volatility clustering and leverage effect) of stock returns as well as give some insights with respect to the correlations across the assets. Linear regression analysis is used in order to estimate two alternative multi-factor asset pricing models. Multi-factor models in financial econometrics are essential not only to describe the data but also to make the estimation of the correlations feasible. In other words, by taking into consideration the large number of stocks that are available in the 1 https://en.wikipedia.org/wiki/Technical_analysis#History 4 market, greater than 15000 (Rachev, et al. (2007)), we are not able to perform the estimation of the correlations without any simplifications. Indices such as the S&P 500 or FTSE 100 contain many stocks and therefore hundreds of thousands of individual correlations. However, the available samples are inadequate to calculate this large number of correlations due to the difficulty in estimating the inverse covariance matrix. Consequently, factor models assist in order to describe all pairwise correlations in respect of less number of correlations among factors. The two linear multi-factor models used in this thesis are the three-factor model of Fama and French (1992, 1993) and a four-factor model that consists of three macroeconomic factors as well as the market’s factor. In particular, the three new factors are the excess returns-in excess of the risk free return rate-of the CPI inflation rate, the USD/GBP exchange rates, and the price of crude oil barrel in UK. In both multi-factor models, the estimated betas are found to be statistically and economically significant for all stock retuns. In addition, the standard GARCH and EGARCH models are used, so as to address the issue of volatility of stock returns, which is also very important as far as forecasts are concerned. GARCH models can capture the "volatility clustering" phenomenon, which is a well known stylized fact of asset returns. In addition, the EGARCH model takes into account the leverage effect, meaning the negative correlation between the past returns and the future volatility. This thesis focuses on the performance of the standard GARCH(1,1) and EGARCH(1,1) models in asset returns’ predictability. According to the standard GARCH(1,1) model it can be observed that four of the thirteen stocks used as sample present high persistence in volatility. Moreover, the results after using the EGARCH(1,1) model show that a leverage effect can be observed in the returns of six stocks. The final purpose of the thesis is to compare the four different models with each other, so as to find which one gives better predictions about future asset returns. The estimated forecasts based on the estimated root mean square error (RMSE), mean absolute error (MAE), and mean absolute percentage error (MAPE) for each model show that both standard GARCH(1,1) and EGARCH(1,1) fit better the data while they also give the most accurate forecasts between the four models. This could be expected since GARCH models capture some of the stylized facts of time series data, while they also successfully 5 predict conditional variances. In addition, VAR(1) models are used in order to test whether the future values of the factors used in the four previous models can be predicted from their past values so as to assist in the accuracy of the conclusions concerning the forecasts. The results show that the forecasted values of the factors and indices used in the models are equal to their conditional means which is optimal. The thesis consists of six parts. The first part is the introduction, which consists of the purpose of study and the problem formulation. In the second part, there is a literature review on various approaches that have been suggested in order to predict future returns. In the third part, data sources are discussed. There is a description of the data which consists of descriptive statistics of returns, indices, and factors as well as multiple graphs. In the fourth part, the model assumptions and formulations of the three-factor model, four- factor model, the standard GARCH (1,1) model, and EGARCH (1,1) model are presented. After that, the coefficients of the estimated models are presented and interpreted as well. In the fifth part, model selection criteria are used in order to compare the four different models with each other and to decide which one fits better on the actual stock returns. In
Details
-
File Typepdf
-
Upload Time-
-
Content LanguagesEnglish
-
Upload UserAnonymous/Not logged-in
-
File Pages60 Page
-
File Size-