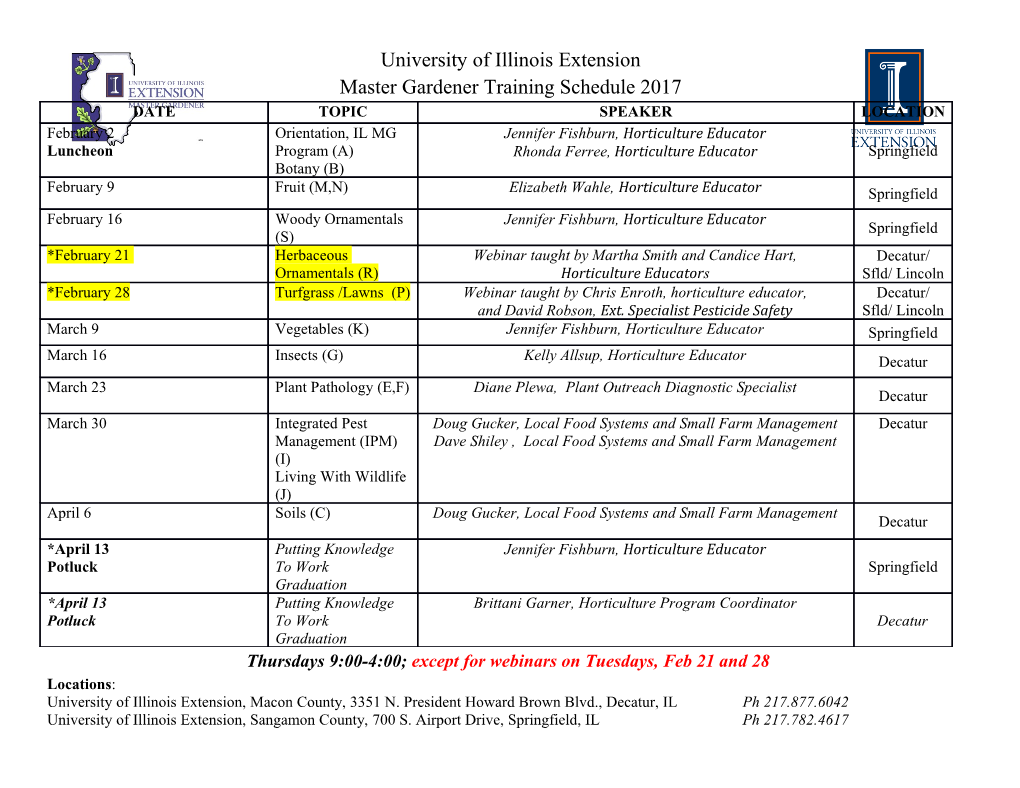
Labour Economics 18 (2011) 464–473 Contents lists available at ScienceDirect Labour Economics journal homepage: www.elsevier.com/locate/labeco The age-productivity gradient: Evidence from a sample of F1 drivers☆ Fabrizio Castellucci a, Mario Padula b, Giovanni Pica c,⁎ a Bocconi University, Italy b University Ca' Foscari of Venice, Italy, CSEF and CEPR c University of Salerno and CSEF, Italy article info abstract Article history: Estimating the effect of aging on productivity requires clean measures of productivity. Additionally, one needs Received 9 July 2009 to control for unobserved heterogeneity at the worker, firm and worker/firm level, to account for the role of Received in revised form 26 August 2010 experience and to correct for selection bias. We tackle these issues exploiting a panel of Gran Prix Formula One Accepted 7 September 2010 drivers, which provides a unique setting to single out the data requirements needed to credibly estimate the Available online 22 September 2011 effect of age on productivity. Results robust to the inclusion of worker, firm and match effects show that the age-productivity link has an inverted U-shape profile with a peak at the age of 30–32. The use of repeated JEL classification: J24 cross-sections of individuals also produces consistent results provided that cohort effects are properly C23 accounted for. Relying on team-average measures of productivity makes instead inference harder. L83 © 2010 Elsevier B.V. All rights reserved. Keywords: Aging Individual effects Firm effects Match effects Formula One Employer–employee matched data 1. Introduction only productivity but also other workers' attributes. Second, unob- served heterogeneity at the worker, firm and worker/firm level The intuitive question of whether individual productivity challenges the identification of the age-productivity gradient in cross- decreases with age is key to many areas of research. The optimality sectional data. Longitudinal data attenuate some identification issues, of wage-seniority schemes rests on the assumption that productivity but give rise to the problem of partialing out the effect of aging from does not fade with age. The decision to invest in human capital the pure effect of time. Thereby, the study of the age-productivity link depends on how individuals expect productivity to change with age in a panel framework requires investigating the role of experience and and on how wages reflect the age-related productivity changes. seniority. Third, selective entry and exit may take place as more Furthermore, the age-productivity gradient is also relevant for labor productive workers may enter the labor force at younger ages and exit demand decisions: the timing of hiring and firing depends on how at older ages. Fourth, jobs differ with respect to the skills they require productivity changes with the age of the workforce. and different skills may evolve very differently over different working Estimating the effect of aging on productivity is however a careers. daunting task. First, it requires clean measures of productivity. In order to overcome these problems, this paper casts the age- Wages are not such measures to the extent that they reward not productivity test within the worker–firm pair in a panel context. We rely on a unique data-set that records the race performances for all Gran Prix Formula One (F1) drivers from 1991 to 1999. Our data-set shares several advantages of both piece rate samples and longitudinal ☆ We are grateful to Markus Frölich and to two anonymous referees for their useful employer–employee matched data-sets. suggestions. We would also like to thank seminar participants at the University Ca' Foscari of Venice and at the University of Milan, and the participants at the Brucchi First, it provides clean measures of productivity. As a measure of Luchino conference, Bologna, December, 11–12, 2008 and the Third ICEE Conference, performance, we use the number of points awarded to each driver at Ancona January 30–31, 2009. The paper was revised while Mario Padula was a Fulbright the end of each race. This is an objective measure of productivity and Scholar at the Department of Economics of Stanford University. The usual disclaimer is not affected by aggregate growth, since the sum of points awarded applies. in each race is constant over time. This is an advantage compared to ⁎ Corresponding author. Tel.: +39 089962831; fax: +39 089963167 E-mail addresses: [email protected] (F. Castellucci), more standard survey data, where the effect of age and time on [email protected] (M. Padula), [email protected] (G. Pica). productivity is much harder to identify separately. 0927-5371/$ – see front matter © 2010 Elsevier B.V. All rights reserved. doi:10.1016/j.labeco.2010.09.002 F. Castellucci et al. / Labour Economics 18 (2011) 464–473 465 Second, it has enough information to identify the age-productivity issues mentioned above, but many remain still unresolved. Overall, profile after controlling for a host of workers' and firms' characteristics. the evidence seems to indicate that the age-productivity profile has an In particular, the panel nature of the data allows to include driver, firm inverted U-shape with substantial decreases after the age of 50. and match effects, thus controlling for the different sources of The early studies of Medoff and Abraham (1980, 1981) and unobserved heterogeneity at the driver, firm, driver/firm level. The Waldman and Avolio (1986) use supervisors' rating to measure presence of driver fixed effects also accounts for the possibility of drivers productivity and find only a slight negative impact of age on entering and exiting the sample in a non-random fashion, as long as performance. These have been important attempts to identify the driver participation decisions are driven by fixed driver attributes.1 relationship between age and productivity, but suffer from severe Third, but equally important, our data allow to account for shortcomings. First, being based on cross-sectional data, they are experience, as measured by the age of entry in the F1 industry, and unable to disentangle the effect of age from the effect of tenure, and still identify the age-productivity gradient. Generally, the age of entry are unable to control for the fact that workers may self-select into cannot be separately identified from the driver effect. However, our firms according to their productivity. Second, supervisors may tend to measure of productivity records the relative performance of each over-reward senior workers for loyalty and past achievements and driver, which allows to restrict to zero the sum of the individual therefore supervisors' rating might be only an imperfect proxy for effects and therefore provides the extra condition needed to identify individual productivity. the effect of the age of entry on productivity. Fourth, F1 drivers all The latter issue has been addressed in later studies. Stephan and perform the same set of tasks, which require the same set of skills. Levin (1998) study researchers in the fields of Physics, Geology, In a first set of estimates we exploit the full set of information Physiology and Biochemistry. The number of publications and the available in the data and show that productivity peaks at the age of standard of the journals they appear in are found to be negatively 30–32 and then decreases. In accordance with the findings of Abowd associated with the researchers' age. Similar evidence is found in the et al. (1999), we also show that worker effects are more important field of economics where Oster and Hamermesh (1998) conclude that than firm and match effects in determining productivity, as they economists above age 50 publish less than younger economists in account respectively for 24, 12, and 2% of the explained variability. leading journals, and that the rate of decline is the same among top and Consistently, we find that omitting either firm or match effects (or non-top researchers.3 The productivity of individuals doing “creative” both) in a model with worker effects does not alter the age- jobs, such as authors and artists, is measured by the quantity and productivity gradient. sometimes the quality of their output. Kanazawa (2003) shows that These results provide new insights on the determinants of individual the age-genius curve of scientists bends down around between 20 and productivity focusing on a sample in which performance is accurately 30 years. Similar curves are also found for jazz musicians and painters. measured and both unobserved heterogeneity and selection bias Fair (2007) focuses on record bests by age for athletes and chess problems can be, at least partially, addressed.2 Given the special players and shows that the decline starts around age 35. These papers nature of the data, it would be of course unwise to generalize our share a common feature, the use of piece-rate samples, which provide results to the general population. However, the richness of the data and a clean measure of productivity. However, given the cross-sectional the neat identification obtained in our setting can be used to nature of these studies, they cannot easily deal with unobserved complement the available evidence on the age-productivity gradient heterogeneity and with selection bias problems. Those settings also do (discussed in section 2) by clarifying what are the minimal data not allow to investigate the effect of the worker–firm match on requirements to credibly estimate the effect of aging on productivity. productivity and to separately identify the effect of age from the effect To this aim, in a second set of estimates we pretend that our data of experience. set is less rich in information than it actually is. We first assume to A different approach is based on the analysis of the age- ignore the identity of the driver, thus relying on cross-sectional productivity relationship exploiting employer–employee matched variation to identify the effect of interest.
Details
-
File Typepdf
-
Upload Time-
-
Content LanguagesEnglish
-
Upload UserAnonymous/Not logged-in
-
File Pages10 Page
-
File Size-